Download PDF
Accelerating One of the Most Sophisticated Automated Railway Scheduling Systems in Europe
Technology Category
- Application Infrastructure & Middleware - Data Exchange & Integration
- Application Infrastructure & Middleware - Middleware, SDKs & Libraries
- Platform as a Service (PaaS) - Connectivity Platforms
Applicable Industries
- Transportation
Applicable Functions
- Business Operation
- Logistics & Transportation
Use Cases
- Fleet Management
- Predictive Maintenance
- Real-Time Location System (RTLS)
- Remote Asset Management
Services
- Software Design & Engineering Services
- System Integration
The Challenge
The effort for developing a timetable solution for railways is a well-studied science, but still not an easy one. It is not a problem that has been completely solved at the scale to which SBB aspires, despite much research and many discussions with other railways and academics. Making it automated via high-performance computations would make it much more efficient. But as one of the densest railways in the world, SBB had many different route combinations to consider, making their planning effort a very large number-crunching problem. The SBB IT team currently uses their computer systems to incorporate extra trains in their current daily timetable and plans to calculate timetables for an entire day algorithmically. This is a huge step forward, as the construction of a valid timetable was a time-consuming effort, even for seasoned timetable planners. Additionally, the manual construction of the timetable is an error-prone task that can lead to plans which often do not work out in practice, causing delays and additional work for the train operation teams. Now that the construction of the timetable can be automated, the planners can concentrate on evaluating different timetable options produced by the computer. A key to optimize this process is fast access to the computed timetable data, so that they can produce the commercially best timetable fast.
About The Customer
The Swiss Federal Railways (SBB) is the national railway company of Switzerland, headquartered in Berne. It operates around 3000 passenger and commercial trains daily, excluding cargo, in an extremely efficient and popular system. SBB was rated first among national European railways in the 2017 European Railway Performance index, which assesses utilization, quality of service, and safety. The IT professionals at SBB are organized as a team of teams that work together to help plan the operations of the railway. Each team has its own Hazelcast deployment for its own distinct needs, including use as a distributed cache to enable extremely fast access and processing of data that originates in a separate data store. One major responsibility is contributing to the workflow that develops the updated train schedules, and teams have specific outputs for their stage of the workflow which they hand to the next team. Tasks include calculating travel time, identifying routing alternatives, and creating the final schedule. The result is a conflict-free train schedule for all of Switzerland that can be implemented by the railway operations team.
The Solution
SBB uses the Hazelcast Platform as a high-performance layer to accelerate data accesses in their planning system. It runs in a private-cloud deployment based on OpenShift, leveraging a microservices architecture that runs on the Spring Boot platform, with Rabbit MQ as the communications layer between microservices. They also have various data storage technologies, including on-premises S3 buckets, and they can quickly populate Hazelcast with that data for use by other parts of the system. Since Hazelcast stores the data in memory, accesses are extremely fast. The decision to use Hazelcast was an easy one, as SBB had previously used another in-memory technology that proved to be too complicated and didn’t offer the ability to scale. The company has since standardized on Hazelcast. The final output is essentially a dashboard that shows trains and stations mapped against temporal data. Planners can drill down into specifics in a very comprehensive visual representation of the timetable.
Operational Impact
Quantitative Benefit
Related Case Studies.
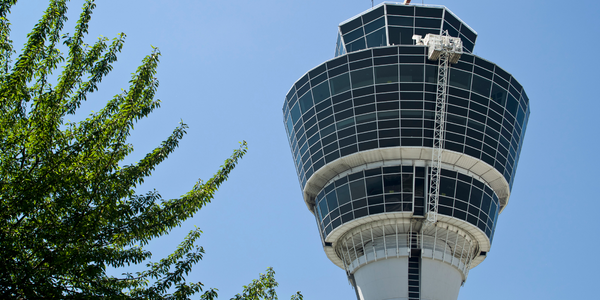
Case Study
Airport SCADA Systems Improve Service Levels
Modern airports are one of the busiest environments on Earth and rely on process automation equipment to ensure service operators achieve their KPIs. Increasingly airport SCADA systems are being used to control all aspects of the operation and associated facilities. This is because unplanned system downtime can cost dearly, both in terms of reduced revenues and the associated loss of customer satisfaction due to inevitable travel inconvenience and disruption.
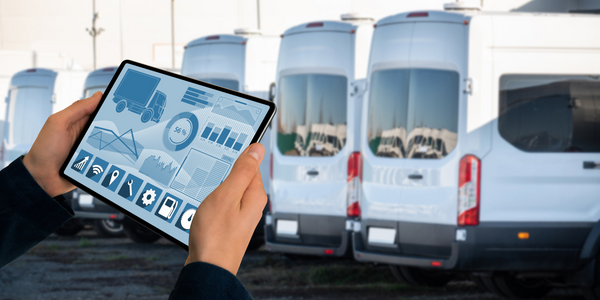
Case Study
IoT-based Fleet Intelligence Innovation
Speed to market is precious for DRVR, a rapidly growing start-up company. With a business model dependent on reliable mobile data, managers were spending their lives trying to negotiate data roaming deals with mobile network operators in different countries. And, even then, service quality was a constant concern.
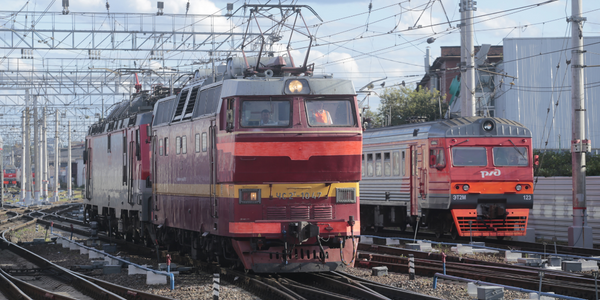
Case Study
Digitize Railway with Deutsche Bahn
To reduce maintenance costs and delay-causing failures for Deutsche Bahn. They need manual measurements by a position measurement system based on custom-made MEMS sensor clusters, which allow autonomous and continuous monitoring with wireless data transmission and long battery. They were looking for data pre-processing solution in the sensor and machine learning algorithms in the cloud so as to detect critical wear.
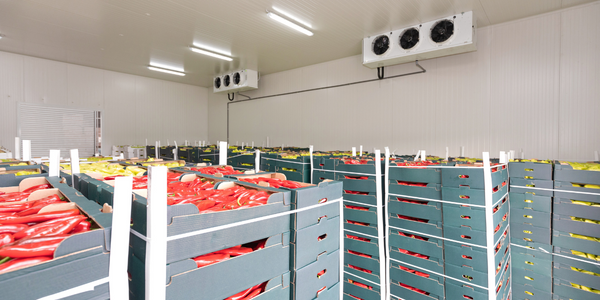
Case Study
Cold Chain Transportation and Refrigerated Fleet Management System
1) Create a digital connected transportation solution to retrofit cold chain trailers with real-time tracking and controls. 2) Prevent multi-million dollar losses due to theft or spoilage. 3) Deliver a digital chain-of-custody solution for door to door load monitoring and security. 4) Provide a trusted multi-fleet solution in a single application with granular data and access controls.
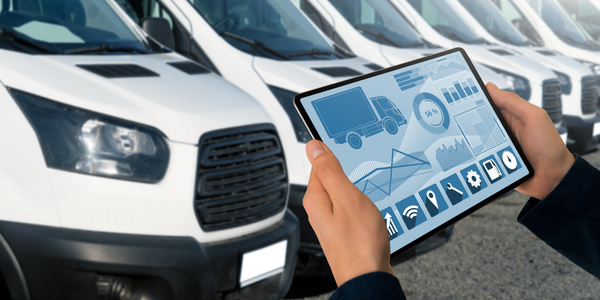
Case Study
Vehicle Fleet Analytics
Organizations frequently implement a maintenance strategy for their fleets of vehicles using a combination of time and usage based maintenance schedules. While effective as a whole, time and usage based schedules do not take into account driving patterns, environmental factors, and sensors currently deployed within the vehicle measuring crank voltage, ignition voltage, and acceleration, all of which have a significant influence on the overall health of the vehicle.In a typical fleet, a large percentage of road calls are related to electrical failure, with battery failure being a common cause. Battery failures result in unmet service agreement levels and costly re-adjustment of scheduled to provide replacement vehicles. To reduce the impact of unplanned maintenance, the transportation logistics company was interested in a trial of C3 Vehicle Fleet Analytics.
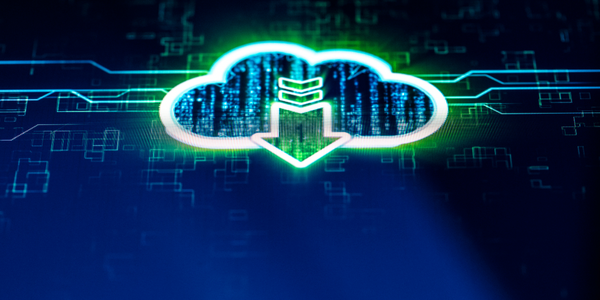
Case Study
3M Gains Real-Time Insight with Cloud Solution
The company has a long track record of innovative technology solutions. For example, 3M helps its customers optimize parking operations by automating fee collection and other processes. To improve support for this rapidly expanding segment, 3M needed to automate its own data collection and reporting. The company had recently purchased the assets of parking, tolling, and automatic license plate reader businesses, and required better insight into these acquisitions. Chad Reed, Global Business Manager for 3M Parking Systems, says, “With thousands of installations across the world, we couldn’t keep track of our software and hardware deployments, which made it difficult to understand our market penetration.” 3M wanted a tracking application that sales staff could use to get real-time information about the type and location of 3M products in parking lots and garages. So that it could be used on-site with potential customers, the solution would have to provide access to data anytime, anywhere, and from an array of mobile devices. Jason Fox, Mobile Application Architect at 3M, upped the ante by volunteering to deliver the new app in one weekend. For Fox and his team, these requirements meant turning to the cloud instead of an on-premises datacenter. “My first thought was to go directly to the cloud because we needed to provide access not only to our salespeople, but to resellers who didn’t have access to our internal network,” says Fox. “The cloud just seemed like a logical choice.”