Download PDF
AES's Transformation in Energy Business with AI and H2O.ai
Technology Category
- Functional Applications - Computerized Maintenance Management Systems (CMMS)
- Sensors - Utility Meters
Applicable Industries
- Electrical Grids
- Renewable Energy
Applicable Functions
- Logistics & Transportation
- Maintenance
Use Cases
- Asset Health Management (AHM)
- Predictive Maintenance
Services
- Cloud Planning, Design & Implementation Services
- Data Science Services
The Challenge
AES, a leading renewable-energy company, was faced with the challenge of accelerating their transition to renewable energy at scale. This business transformation necessitated a digital and AI transformation to better predict and optimize the energy output from renewable sources, predict failures, and optimize load distribution. The company had to deal with the complexities of wind-turbine predictive maintenance, energy bidding strategy for hydroelectric power plants, and smart meters. The maintenance of wind turbines, which have numerous moving parts subjected to harsh environments, was particularly costly and time-consuming. The company also needed to optimize its energy bidding strategy to maximize revenue from its hydroelectric power plants. Additionally, the company had to manage over one million smart meters, which sometimes had maintenance issues or were subject to misuse.
About The Customer
AES is a globally operating renewable-energy company that produces and distributes energy for private, public, and governmental organizations. The company has been named one of the World’s Most Ethical Companies for nine consecutive years and has won the Edison Electric Institute’s (EEI’s) Edison Award for contributing to the advancement of the electric industry. AES has successfully transitioned their business from fossil fuels to renewables and is accelerating this change with successful digital and AI transformations. The company runs numerous wind turbine farms, owns and operates numerous global hydroelectric power plants, and leverages more than one-million smart meters to monitor energy consumption.
The Solution
AES started by creating an AI strategy and then worked closely with their business partners to determine the best starting use cases. They selected the H2O AI Cloud to accelerate their ability to make state-of-the-art AI models and get them into production. For wind-turbine predictive maintenance, they built about a dozen models that provided greater than 90% accuracy. The initiative delivered cost savings and more consistent power delivery, leading to the addition of more sensors to multiple wind turbine components and automating oil sampling. For energy bidding strategy, AES used models made and operated with the H2O AI Cloud to help set the daily price and amounts for their power plants. For smart meters, the team created AI based on data from the smart meters to predict actual maintenance issues vs. misuse. The company is also expanding its predictive maintenance efforts to include imagery from drones to assess blade damage and work on solar farms to identify poor performing panels and cleaning schedules.
Operational Impact
Quantitative Benefit
Related Case Studies.
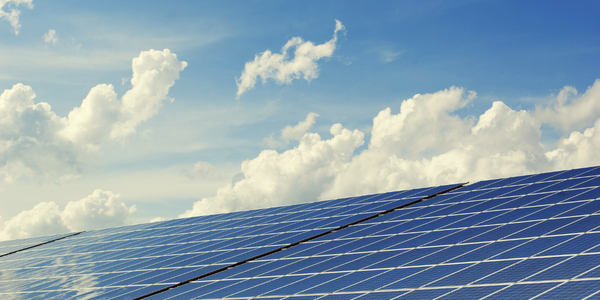
Case Study
Remote Monitoring & Predictive Maintenance App for a Solar Energy System
The maintenance & tracking of various modules was an overhead for the customer due to the huge labor costs involved. Being an advanced solar solutions provider, they wanted to ensure early detection of issues and provide the best-in-class customer experience. Hence they wanted to automate the whole process.
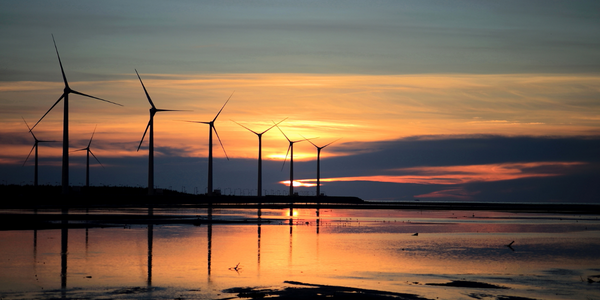
Case Study
Vestas: Turning Climate into Capital with Big Data
Making wind a reliable source of energy depends greatly on the placement of the wind turbines used to produce electricity. Turbulence is a significant factor as it strains turbine components, making them more likely to fail. Vestas wanted to pinpoint the optimal location for wind turbines to maximize power generation and reduce energy costs.
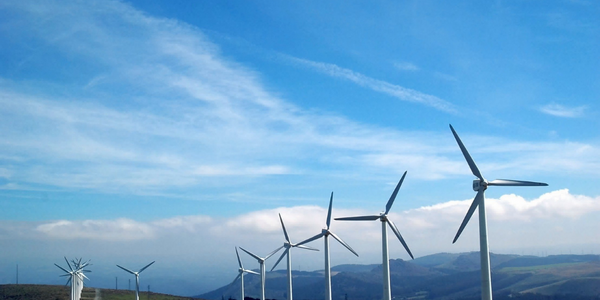
Case Study
Siemens Wind Power
Wind provides clean, renewable energy. The core concept is simple: wind turbines spin blades to generate power. However, today's systems are anything but simple. Modern wind turbines have blades that sweep a 120 meter circle, cost more than 1 million dollars and generate multiple megawatts of power. Each turbine may include up to 1,000 sensors and actuators – integrating strain gages, bearing monitors and power conditioning technology. The turbine can control blade speed and power generation by altering the blade pitch and power extraction. Controlling the turbine is a sophisticated job requiring many cooperating processors closing high-speed loops and implementing intelligent monitoring and optimization algorithms. But the real challenge is integrating these turbines so that they work together. A wind farm may include hundreds of turbines. They are often installed in difficult-to-access locations at sea. The farm must implement a fundamentally and truly distributed control system. Like all power systems, the goal of the farm is to match generation to load. A farm with hundreds of turbines must optimize that load by balancing the loading and generation across a wide geography. Wind, of course, is dynamic. Almost every picture of a wind farm shows a calm sea and a setting sun. But things get challenging when a storm goes through the wind farm. In a storm, the control system must decide how to take energy out of gusts to generate constant power. It must intelligently balance load across many turbines. And a critical consideration is the loading and potential damage to a half-billion-dollar installed asset. This is no environment for a slow or undependable control system. Reliability and performance are crucial.
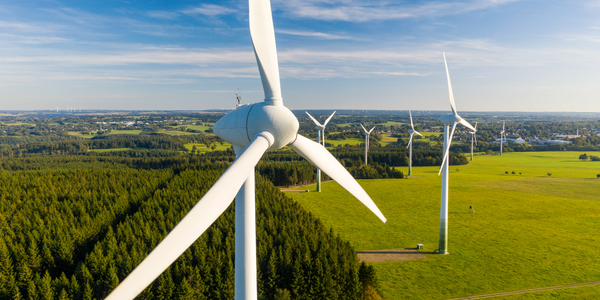
Case Study
Remote Monitoring and Control for a Windmill Generator
As concerns over global warming continue to grow, green technologies are becoming increasingly popular. Wind turbine companies provide an excellent alternative to burning fossil fuels by harnessing kinetic energy from the wind and converting it into electricity. A typical wind farm may include over 80 wind turbines so efficient and reliable networks to manage and control these installations are imperative. Each wind turbine includes a generator and a variety of serial components such as a water cooler, high voltage transformer, ultrasonic wind sensors, yaw gear, blade bearing, pitch cylinder, and hub controller. All of these components are controlled by a PLC and communicate with the ground host. Due to the total integration of these devices into an Ethernet network, one of our customers in the wind turbine industry needed a serial-to-Ethernet solution that can operate reliably for years without interruption.
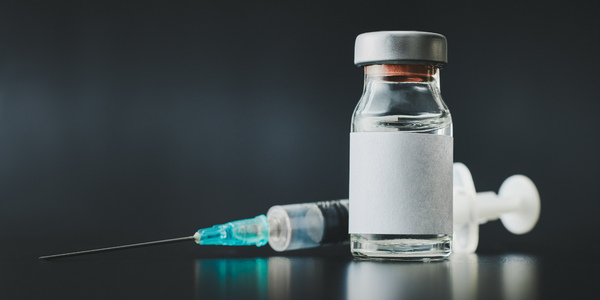
Case Study
Temperature monitoring for vaccine fridges
Dulas wanted a way to improve the reliability of the cold chain, facilitating maintenance and ensuring fewer vaccines are spoiled. Dulas wanted an M2M solution which would enable them to record and report the temperature inside vaccine refrigerators.
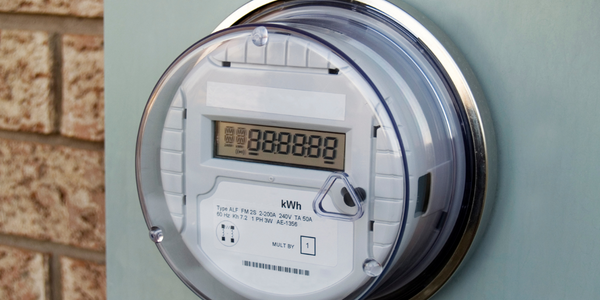
Case Study
Hydro One Leads the Way In Smart Meter Development
In 2010, Ontario’s energy board mandated that time-of-use (TOU) pricing for consumers be available for all consumers on a regulated price plan. To meet this requirement, Hydro One needed to quickly deploy a smart meter and intelligent communications network solution to meet the provincial government’s requirement at a low cost. The network needed to cover Hydro One’s expansive service territory, which has a land mass twice the size of Texas, and its customers live in a mix of urban, rural, and remote areas, some places only accessible by air, rail, boat or snowmobile. Most importantly, the network needed to enable future enterprise-wide business efficiencies, modernization of distribution infrastructure and enhanced customer service. To meet these needs, Hydro One conceptualized an end-to-end solution leveraging open standards and Internet Protocols (IP) at all communication levels. The utility drew upon industry leaders like Trilliant to realize this vision.