Deep Learning Boosts Robotic Picking Flexibility
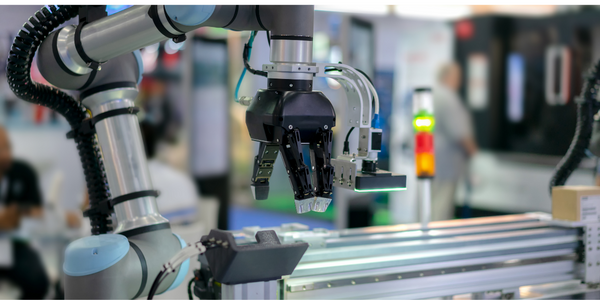
- Analytics & Modeling - Machine Learning
- Discrete Manufacturing
- Logistics & Transportation
- Factory Operations Visibility & Intelligence
- Software Design & Engineering Services
Gripping and manipulating items of diverse shapes and sizes have long been one of the biggest challenges facing industrial robotics. The difficulty is perhaps best summed up by the Polanyi Paradox, which states that we "know more than we can tell." In essence, while it may be easy to teach machines to exhibit a high level of performance on tasks that require abstract reasoning such as running computations, it is substantially harder to grant them the sensory-motor skills of even a small child in all but the most standardized and predictable environments.
However, with the need for flexible picking to accommodate reduced changeover time for more varied product runs on the rise, industry is pursuing new solutions to the problem.
A new collaboration project between Festo and the Karlsruhe Institute of Technology (KIT) called FLAIRPOP (Federated Learning for Robot Picking), seeks to use training data from multiple stations, plants, or even companies to more effectively drive deep learning algorithms with the goal of helping picking robots to become more adaptable. The approach is similar to that used by cloud-driven machine learning algorithms, which leverage larger amounts of data than individual end-users have access to.
Related Case Studies.
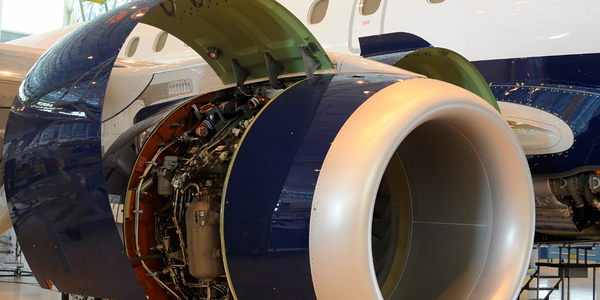
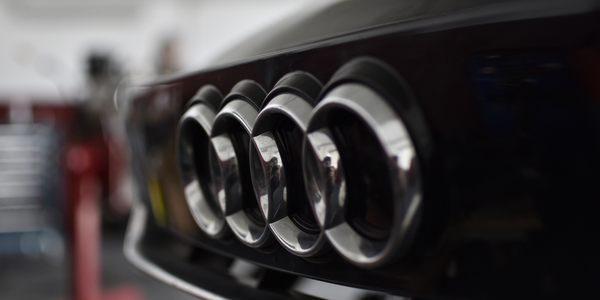
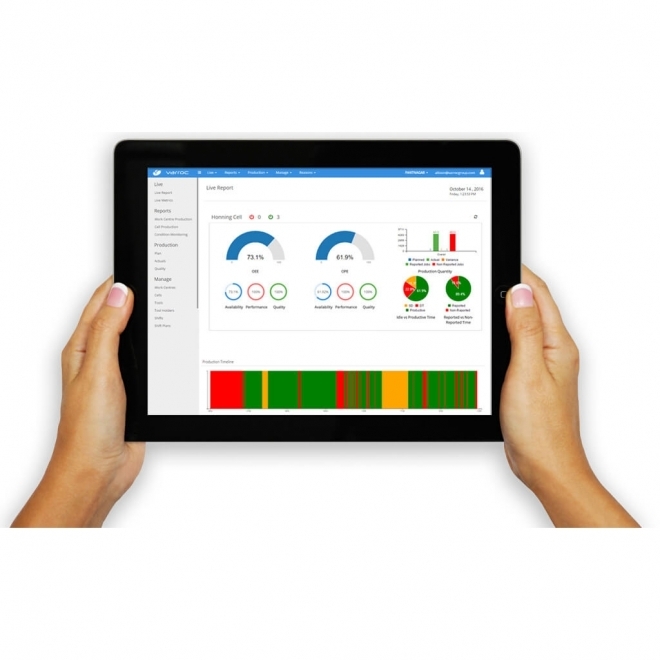
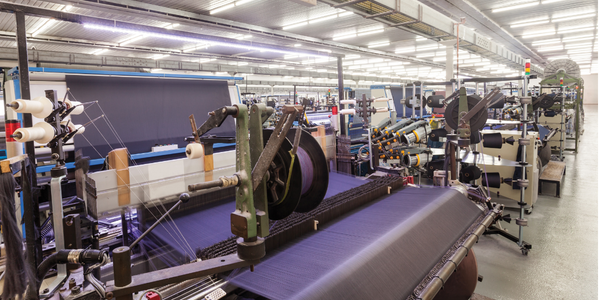
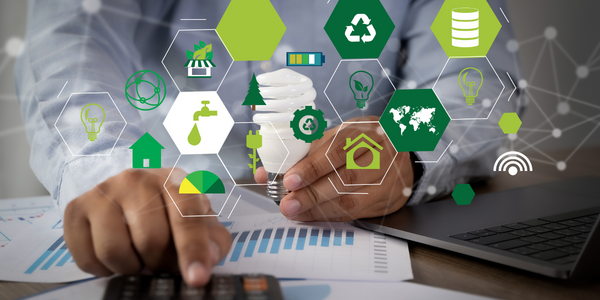