Download PDF
Digital Twins Support Supply Chain Optimization
Technology Category
- Analytics & Modeling - Digital Twin / Simulation
- Analytics & Modeling - Predictive Analytics
Applicable Industries
- Chemicals
Applicable Functions
- Maintenance
Use Cases
- Predictive Maintenance
- Supply Chain Visibility
Services
- Data Science Services
The Challenge
The chemicals industry is complex with a small number of raw materials often transformed into hundreds of thousands of final products. The industry employs expensive, heavy, and complex manufacturing assets that can cover the full spectrum of process operations: continuous, semi-continuous, or batch. Shutting down and then restarting the process is expensive; time consuming (think days, not hours); and has environmental, health, and safety implications. A hyper compressor’s job is to build up pressure needed in the conversion process. These compressors typically go down many times a year. Mitigating this problem could be worth millions of dollars to chemical companies.
About The Customer
The customer in this case study is the chemicals industry. This industry is characterized by the transformation of a small number of raw materials into hundreds of thousands of final products. The industry employs expensive, heavy, and complex manufacturing assets that can cover the full spectrum of process operations: continuous, semi-continuous, or batch. Shutting down and then restarting the process is expensive, time-consuming, and has environmental, health, and safety implications. A key piece of machinery in this industry is the hyper compressor, which is used to build up the pressure needed in the conversion process. These compressors typically go down many times a year, which can lead to increased manufacturing costs and service failures for end users.
The Solution
AspenTech offers a solution that includes optimized production scheduling based on an integrated digital twin maintenance model. The digital twin concept combines the ideas of modeling and the Internet of Things (IoT). Most often, the digital twin concept has been applied to assets. A piece of machinery generates data on vibration, temperature, pressure, and other things. That data is used to predict a machine’s failure and apply preventive maintenance to help avoid costly unplanned downtime. For supply chain planning, plant machinery is the key area for which an asset’s failure could lead to increased manufacturing costs and service failures for end users. The supply chain model does not look to predict asset failures, but could use inputs from the digital twin maintenance model to improve scheduling.
Operational Impact
Quantitative Benefit
Related Case Studies.
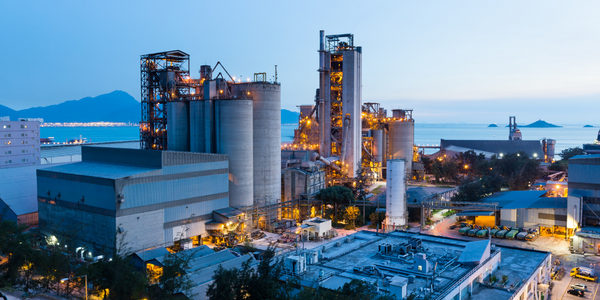
Case Study
Honeywell - Tata Chemicals Improves Data Accessibility with OneWireless
Tata was facing data accessibility challenges in the cement plant control room tapping signals from remote process control areas and other distant locations, including the gas scrubber. Tata needed a wireless solution to extend its control network securely to remote locations that would also provide seamless communication with existing control applications.
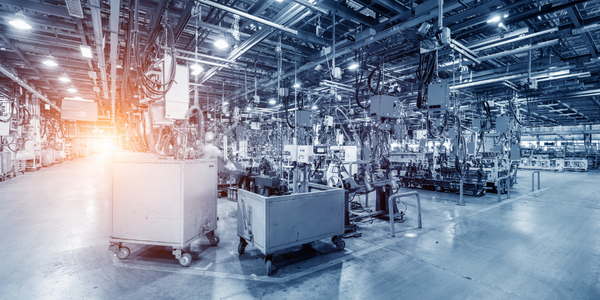
Case Study
Advanced Elastomer Systems Upgrades Production
In order to maintain its share of the international market for thermoplastic elastomers AES recently expanded its Florida plant by adding a new production line. While the existing lines were operating satisfactorily using a PROVOX distributed control system with traditional analog I/O, AES wanted advanced technology on the new line for greater economy, efficiency, and reliability. AES officials were anxious to get this line into production to meet incoming orders, but two hurricanes slowed construction.
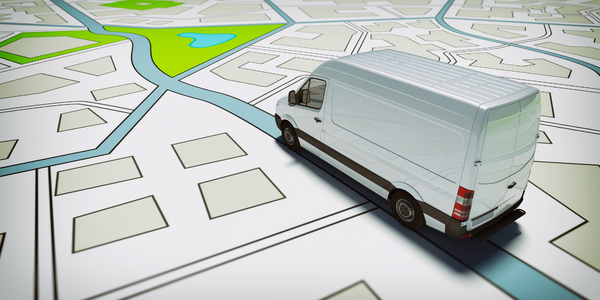
Case Study
Wireless GPS Tracking & Security Monitoring
Enhancing the security of hazardous freight and ensuring compliance with Homeland Security’s Transportation Security Administration mandate that all trains carrying chemicals capable of creating a toxic inhalation condition are equipped with on-board safety monitoring systems.
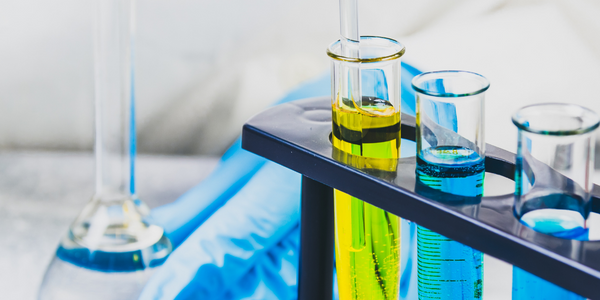
Case Study
Field Device Asset Management For Chemical Company in China
Chinese chemical subsidiary of multinational corporation serves customers throughout the world. Sales offices and research and technology centers are strategically located to provide rapid response to customer requests. Just two workers were assigned to maintain thousands of intelligent instruments in three production units, so they could do little more than react to device issues as they appeared. This costly maintenance method inevitably led to unexpected downtime when a critical instrument failed. Plant management recognized the need to change from reactive to predictive maintenance for all assets, including instruments and control valves, but help was needed in implementing such a technology-based initiative.
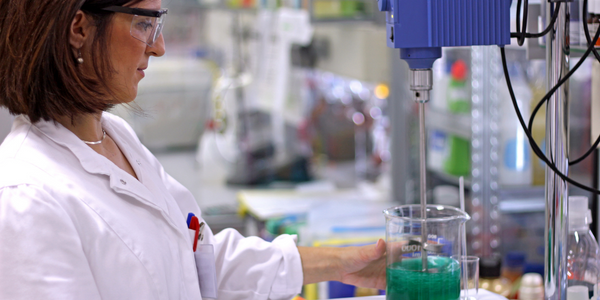
Case Study
Industrial Workforce Mobility for Improved Safety & Operations
Huntsman Corporation, a global manufacturer and marketer of differentiated chemicals, undertook an aggressive program to eliminate injuries, product defects, and environmental releases at their Port Neches facility. Termed “Project Zero”, this program required a completely mobile solution to empower operations and maintenance personnel to capture defects, track work progress and make process and safety related decisions in real-time.