Download PDF
Emulating Engineering Expertise with AI: Crashworthiness Optimization at BMW
Technology Category
- Analytics & Modeling - Machine Learning
Applicable Industries
- Automotive
- Education
Applicable Functions
- Human Resources
- Product Research & Development
Use Cases
- Predictive Maintenance
- Time Sensitive Networking
Services
- Data Science Services
The Challenge
BMW, a leading manufacturer of premium automobiles and motorcycles, faced a significant challenge in ensuring passenger safety during crash events. The complexity of orchestrating the chain of events during a crash, including the timing of discrete events such as bolts breaking or parts coming into contact, was a daunting task. The key performance indicators, including energy absorption, peak force level before failure, local displacements, and weight, were either too complex or resulted in over-constrained optimization problems. This made it difficult to confidently validate crashworthiness within the fast-paced product development process. The challenge was to find a solution that could simplify this process, reduce the number of design iterations required, and ensure the highest level of safety for passengers.
About The Customer
The BMW Group is the world’s leading manufacturer of premium automobiles and motorcycles, with 31 production and assembly facilities in 15 countries and a global sales network. The company is also a provider of premium financial and mobility services. As an innovation leader, BMW sets trends in production technology and sustainability with an intelligent material mix, a technological shift towards digitalization, and resource-efficient production. The company also ensures competitiveness through flexibility and continuous optimization of value chains.
The Solution
BMW turned to Altair’s integrated Machine Learning solutions within HyperWorks to generate optimization constraints that mimic engineering expertise. The solution involved the use of clustering, an unsupervised machine learning algorithm, to help engineers understand how crash kinematics affect key performance indicators. Favourable crash kinematics were then enforced during the optimization process through the use of a classifier that emulates engineering decision making. This approach simplified the formulation of the optimization problem and reduced the number of design iterations required to develop complex automotive crash structures. The machine learning-powered workflow augmented and extended BMW’s existing engineering expertise, allowing them to more efficiently allocate computing and human resources to high-value simulation, analysis, and validation efforts.
Operational Impact
Related Case Studies.
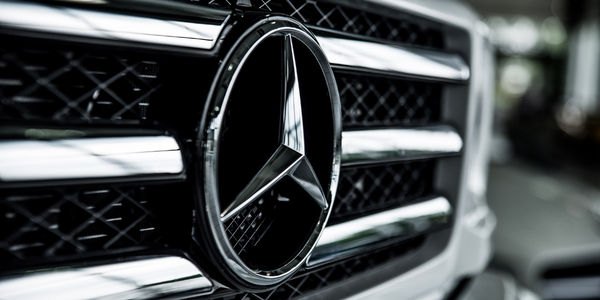
Case Study
Integral Plant Maintenance
Mercedes-Benz and his partner GAZ chose Siemens to be its maintenance partner at a new engine plant in Yaroslavl, Russia. The new plant offers a capacity to manufacture diesel engines for the Russian market, for locally produced Sprinter Classic. In addition to engines for the local market, the Yaroslavl plant will also produce spare parts. Mercedes-Benz Russia and his partner needed a service partner in order to ensure the operation of these lines in a maintenance partnership arrangement. The challenges included coordinating the entire maintenance management operation, in particular inspections, corrective and predictive maintenance activities, and the optimizing spare parts management. Siemens developed a customized maintenance solution that includes all electronic and mechanical maintenance activities (Integral Plant Maintenance).
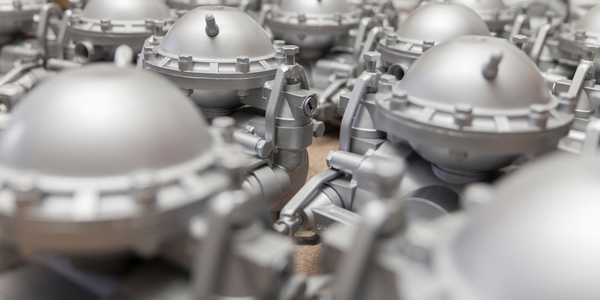
Case Study
Monitoring of Pressure Pumps in Automotive Industry
A large German/American producer of auto parts uses high-pressure pumps to deburr machined parts as a part of its production and quality check process. They decided to monitor these pumps to make sure they work properly and that they can see any indications leading to a potential failure before it affects their process.