Download PDF
Enhancing Autonomous Trucking with Synthetic Data: A Kodiak Robotics Case Study
Technology Category
- Cybersecurity & Privacy - Identity & Authentication Management
- Sensors - Autonomous Driving Sensors
Applicable Industries
- Plastics
- Transportation
Applicable Functions
- Logistics & Transportation
Use Cases
- Autonomous Transport Systems
- Virtual Training
Services
- System Integration
- Training
The Challenge
Kodiak Robotics, an autonomous technology company, is developing self-driving capabilities for the long-haul trucking industry. The company uses a unique sensor fusion system and a lightweight mapping solution to navigate highway driving and deliver freight efficiently. However, the company faced a significant challenge in training its software to handle rare scenarios, such as pedestrians walking on the highway. These edge cases are crucial for a production-level autonomous vehicle system, but collecting enough real-world examples to train the models reliably was proving difficult.
About The Customer
Kodiak Robotics is a Mountain View, CA-based autonomous technology company that is revolutionizing the long-haul trucking industry. The company is developing self-driving capabilities and technologies to navigate all aspects of highway driving and deliver freight efficiently and on-time. The team, which includes several self-driving industry veterans, is committed to building the world's most efficient, reliable, and respected end-to-end delivery solution. Kodiak's unique approach involves leveraging a sensor fusion system combined with a lightweight mapping solution.
The Solution
To overcome this challenge, Kodiak Robotics partnered with Scale to provide synthetic data to augment their existing ground-truth training data with simulated pedestrians. Scale's unique human-in-the-loop synthetic data generation process creates diverse and realistic synthetic data. Trained taskers validate the placement and poses of synthetic pedestrians to ensure the synthetic data is realistic. The data is delivered via the same dashboard and APIs as Kodiak's existing annotation pipeline, ensuring seamless integration. Additionally, Kodiak uses Nucleus to identify specific scenes in their dataset that had edge cases they needed to improve their model on. This includes scenes where construction workers are present and where a vehicle is traveling under a bridge.
Operational Impact
Related Case Studies.
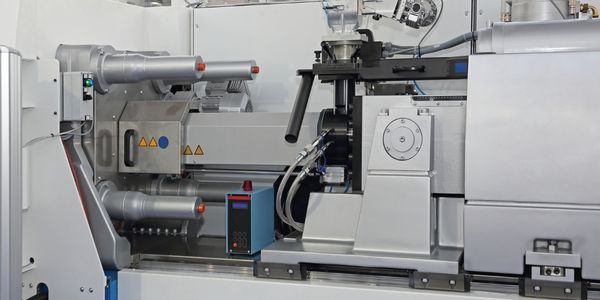
Case Study
Plastic Spoons Case study: Injection Moulding
In order to meet customer expectations by supplying a wide variety of packaging units, from 36 to 1000 spoons per package, a new production and packaging line needed to be built. DeSter wanted to achieve higher production capacity, lower cycle time and a high degree of operator friendliness with this new production line.
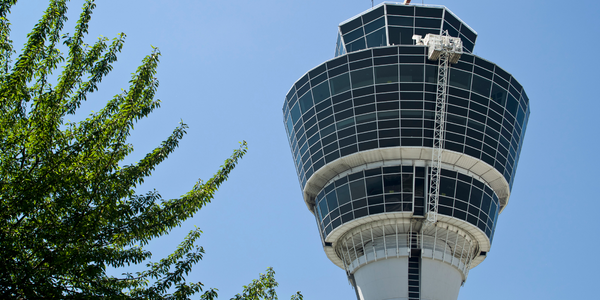
Case Study
Airport SCADA Systems Improve Service Levels
Modern airports are one of the busiest environments on Earth and rely on process automation equipment to ensure service operators achieve their KPIs. Increasingly airport SCADA systems are being used to control all aspects of the operation and associated facilities. This is because unplanned system downtime can cost dearly, both in terms of reduced revenues and the associated loss of customer satisfaction due to inevitable travel inconvenience and disruption.
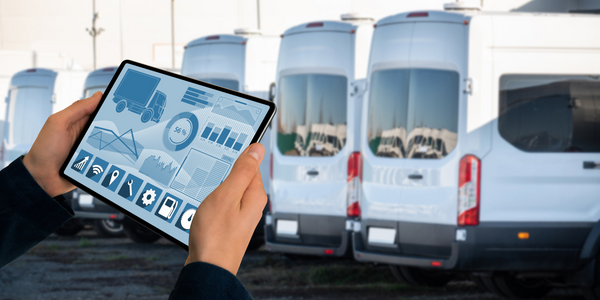
Case Study
IoT-based Fleet Intelligence Innovation
Speed to market is precious for DRVR, a rapidly growing start-up company. With a business model dependent on reliable mobile data, managers were spending their lives trying to negotiate data roaming deals with mobile network operators in different countries. And, even then, service quality was a constant concern.
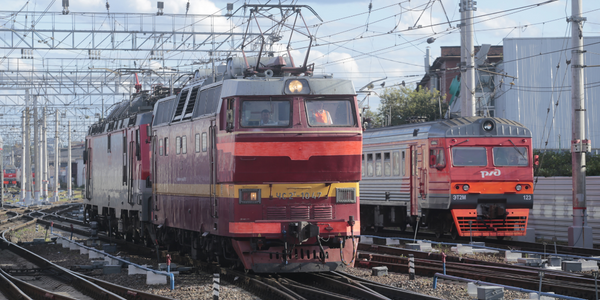
Case Study
Digitize Railway with Deutsche Bahn
To reduce maintenance costs and delay-causing failures for Deutsche Bahn. They need manual measurements by a position measurement system based on custom-made MEMS sensor clusters, which allow autonomous and continuous monitoring with wireless data transmission and long battery. They were looking for data pre-processing solution in the sensor and machine learning algorithms in the cloud so as to detect critical wear.
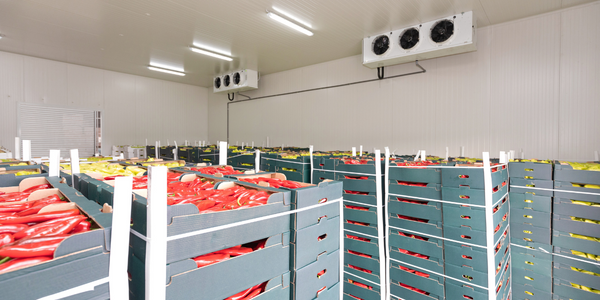
Case Study
Cold Chain Transportation and Refrigerated Fleet Management System
1) Create a digital connected transportation solution to retrofit cold chain trailers with real-time tracking and controls. 2) Prevent multi-million dollar losses due to theft or spoilage. 3) Deliver a digital chain-of-custody solution for door to door load monitoring and security. 4) Provide a trusted multi-fleet solution in a single application with granular data and access controls.
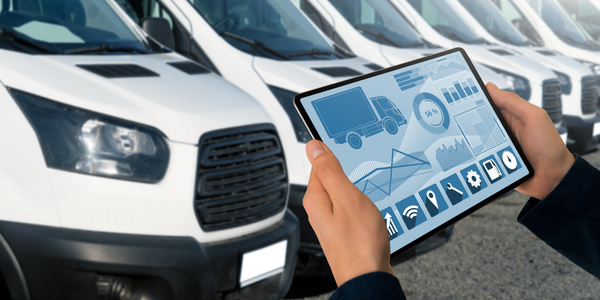
Case Study
Vehicle Fleet Analytics
Organizations frequently implement a maintenance strategy for their fleets of vehicles using a combination of time and usage based maintenance schedules. While effective as a whole, time and usage based schedules do not take into account driving patterns, environmental factors, and sensors currently deployed within the vehicle measuring crank voltage, ignition voltage, and acceleration, all of which have a significant influence on the overall health of the vehicle.In a typical fleet, a large percentage of road calls are related to electrical failure, with battery failure being a common cause. Battery failures result in unmet service agreement levels and costly re-adjustment of scheduled to provide replacement vehicles. To reduce the impact of unplanned maintenance, the transportation logistics company was interested in a trial of C3 Vehicle Fleet Analytics.