Download PDF
Helped a B2B Retailer analyze potential customers at street level to increase sales conversion ratio
Technology Category
- Analytics & Modeling - Data Mining
- Analytics & Modeling - Predictive Analytics
Applicable Industries
- Retail
Applicable Functions
- Sales & Marketing
Use Cases
- Demand Planning & Forecasting
- Predictive Replenishment
Services
- Data Science Services
The Challenge
The trading and distribution company wanted to increase its market share by adding new customers to its portfolio. The client did not have a comprehensive list of the customers which they were not servicing. The company aimed to increase their customer base by identifying potential customers on a street level basis and obtain a mix of existing and potential customers to identify and target the segments which would be most profitable.
About The Customer
The client is a B2B Retailer company operating in the retail industry. The company is involved in trading and distribution and is looking to increase its market share. The company's goal is to add new customers to its portfolio, but it lacks a comprehensive list of customers it is not currently servicing. The company aims to increase its customer base by identifying potential customers at the street level. The company also wants to obtain a mix of existing and potential customers to identify and target the most profitable segments.
The Solution
The solution involved using Web Crawling/Web Scraping technique to get the exhaustive list of customers available in that region. Variables such as menu price, longitude & latitude, customer group, chain/non-chain and derived variables (distance from the nearest existing customer) were used for further analysis. A highly interactive web app was built using R for easy viewing of existing and non-customers along with magnifier features like zoom in & zoom out. The concentration of both existing and non-customers was analyzed to identify low or high coverage districts and/or streets. A model was built using SVM Classification technique to find the probability score of non customers and categorize them into ‘high’, ‘medium’ and ‘low’ potential customers.
Operational Impact
Quantitative Benefit
Related Case Studies.
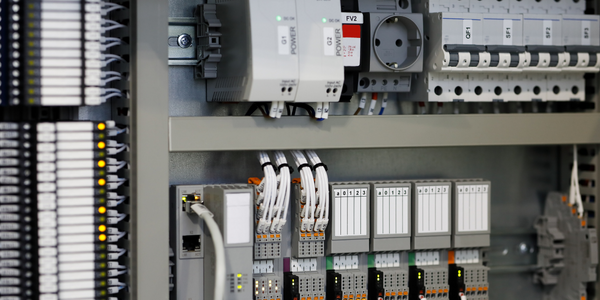
Case Study
Improving Production Line Efficiency with Ethernet Micro RTU Controller
Moxa was asked to provide a connectivity solution for one of the world's leading cosmetics companies. This multinational corporation, with retail presence in 130 countries, 23 global braches, and over 66,000 employees, sought to improve the efficiency of their production process by migrating from manual monitoring to an automatic productivity monitoring system. The production line was being monitored by ABB Real-TPI, a factory information system that offers data collection and analysis to improve plant efficiency. Due to software limitations, the customer needed an OPC server and a corresponding I/O solution to collect data from additional sensor devices for the Real-TPI system. The goal is to enable the factory information system to more thoroughly collect data from every corner of the production line. This will improve its ability to measure Overall Equipment Effectiveness (OEE) and translate into increased production efficiencies. System Requirements • Instant status updates while still consuming minimal bandwidth to relieve strain on limited factory networks • Interoperable with ABB Real-TPI • Small form factor appropriate for deployment where space is scarce • Remote software management and configuration to simplify operations
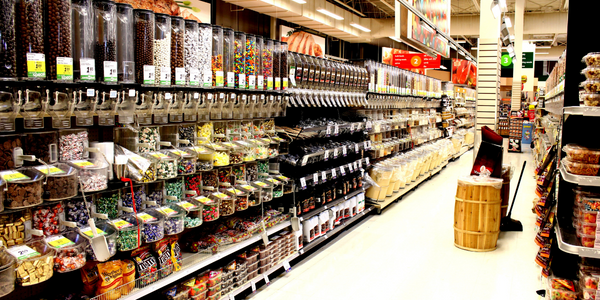
Case Study
How Sirqul’s IoT Platform is Crafting Carrefour’s New In-Store Experiences
Carrefour Taiwan’s goal is to be completely digital by end of 2018. Out-dated manual methods for analysis and assumptions limited Carrefour’s ability to change the customer experience and were void of real-time decision-making capabilities. Rather than relying solely on sales data, assumptions, and disparate systems, Carrefour Taiwan’s CEO led an initiative to find a connected IoT solution that could give the team the ability to make real-time changes and more informed decisions. Prior to implementing, Carrefour struggled to address their conversion rates and did not have the proper insights into the customer decision-making process nor how to make an immediate impact without losing customer confidence.
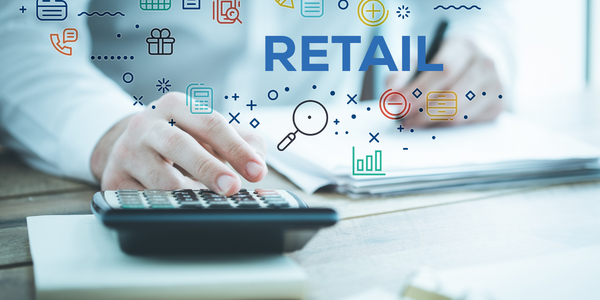
Case Study
Digital Retail Security Solutions
Sennco wanted to help its retail customers increase sales and profits by developing an innovative alarm system as opposed to conventional connected alarms that are permanently tethered to display products. These traditional security systems were cumbersome and intrusive to the customer shopping experience. Additionally, they provided no useful data or analytics.
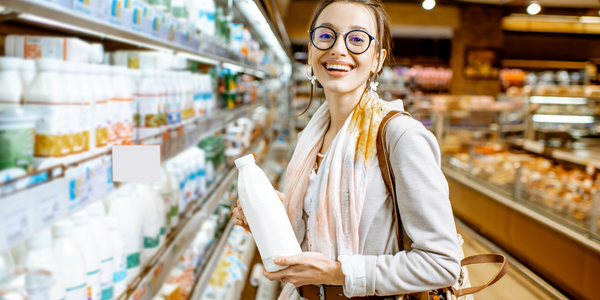
Case Study
Ensures Cold Milk in Your Supermarket
As of 2014, AK-Centralen has over 1,500 Danish supermarkets equipped, and utilizes 16 operators, and is open 24 hours a day, 365 days a year. AK-Centralen needed the ability to monitor the cooling alarms from around the country, 24 hours a day, 365 days a year. Each and every time the door to a milk cooler or a freezer does not close properly, an alarm goes off on a computer screen in a control building in southwestern Odense. This type of alarm will go off approximately 140,000 times per year, equating to roughly 400 alarms in a 24-hour period. Should an alarm go off, then there is only a limited amount of time to act before dairy products or frozen pizza must be disposed of, and this type of waste can quickly start to cost a supermarket a great deal of money.
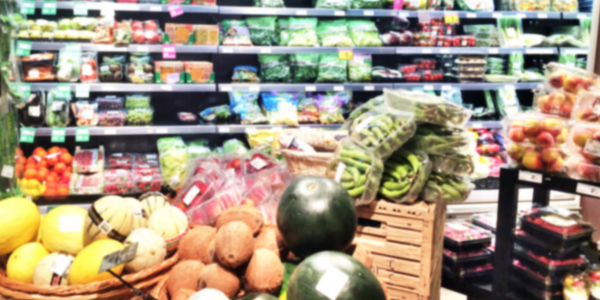
Case Study
Supermarket Energy Savings
The client had previously deployed a one-meter-per-store monitoring program. Given the manner in which energy consumption changes with external temperature, hour of the day, day of week and month of year, a single meter solution lacked the ability to detect the difference between a true problem and a changing store environment. Most importantly, a single meter solution could never identify root cause of energy consumption changes. This approach never reduced the number of truck-rolls or man-hours required to find and resolve issues.