Download PDF
Leveraging an insurance customer retrieval solution to reactivate lapsed accounts
Technology Category
- Analytics & Modeling - Predictive Analytics
Applicable Industries
- Finance & Insurance
Applicable Functions
- Sales & Marketing
Use Cases
- Predictive Replenishment
Services
- Data Science Services
The Challenge
The client, a leading insurance company, was facing a challenge of accessing lapsed insurance policies with a potential of repayment within a specific time bracket. The company was witnessing revenue loss and wanted to reactivate these lapsed policies. However, they wanted to ensure that the strategy resulted in minimum wastage of money and effort.
About The Customer
The customer in this case study is a leading insurance company operating in the financial services industry. The company was facing a challenge with lapsed insurance policies and was seeking a solution to reactivate these accounts. The company was experiencing revenue loss due to these lapsed policies and was looking for a strategy that would result in minimum wastage of money and effort. The company's goal was to identify these lapsed accounts and focus on their reactivation, which would result in an additional flow of revenue.
The Solution
Blueocean Market Intelligence customized their insurance customer retrieval solution to address this problem. They accessed the lapsed policies that had the potential of repayment by invoking inforce attributes on the defaulted policies. A binary logistic regression was utilized on lapsed and inforce datasets and a KS cutoff was decided based on the model results. A confusion matrix was built consisting of all the four wells. Factors like premium to be paid, income of the policy holder, occupation and the total sum assured at the end of maturity were found to be greatly affecting the model results.
Operational Impact
Quantitative Benefit
Related Case Studies.
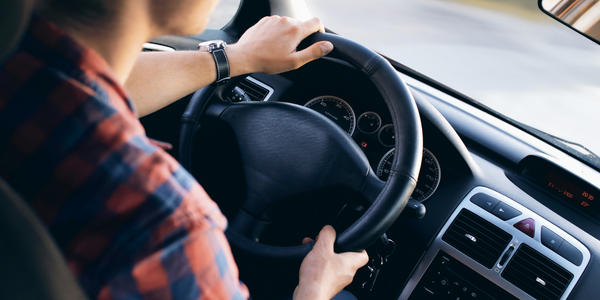
Case Study
Real-time In-vehicle Monitoring
The telematic solution provides this vital premium-adjusting information. The solution also helps detect and deter vehicle or trailer theft – as soon as a theft occurs, monitoring personnel can alert the appropriate authorities, providing an exact location.“With more and more insurance companies and major fleet operators interested in monitoring driver behaviour on the grounds of road safety, efficient logistics and costs, the market for this type of device and associated e-business services is growing rapidly within Italy and the rest of Europe,” says Franco.“The insurance companies are especially interested in the pay-per-use and pay-as-you-drive applications while other organisations employ the technology for road user charging.”“One million vehicles in Italy currently carry such devices and forecasts indicate that the European market will increase tenfold by 2014.However, for our technology to work effectively, we needed a highly reliable wireless data network to carry the information between the vehicles and monitoring stations.”
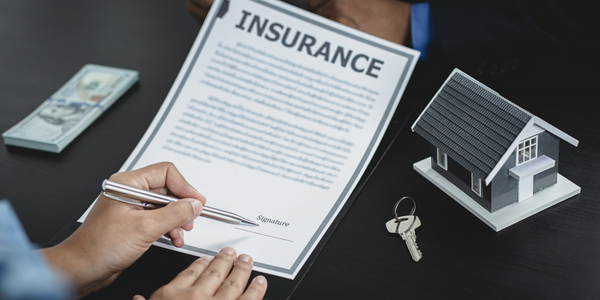
Case Study
Safety First with Folksam
The competitiveness of the car insurance market is driving UBI growth as a means for insurance companies to differentiate their customer propositions as well as improving operational efficiency. An insurance model - usage-based insurance ("UBI") - offers possibilities for insurers to do more efficient market segmentation and accurate risk assessment and pricing. Insurers require an IoT solution for the purpose of data collection and performance analysis
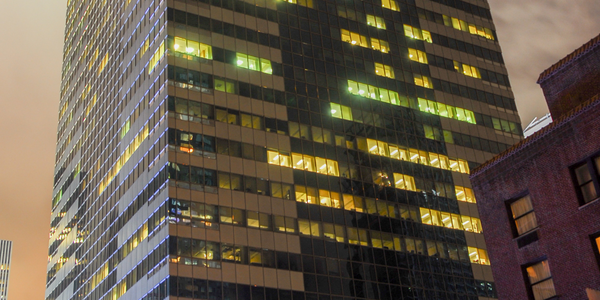
Case Study
Smooth Transition to Energy Savings
The building was equipped with four end-of-life Trane water cooled chillers, located in the basement. Johnson Controls installed four York water cooled centrifugal chillers with unit mounted variable speed drives and a total installed cooling capacity of 6,8 MW. Each chiller has a capacity of 1,6 MW (variable to 1.9MW depending upon condenser water temperatures). Johnson Controls needed to design the equipment in such way that it would fit the dimensional constraints of the existing plant area and plant access route but also the specific performance requirements of the client. Morgan Stanley required the chiller plant to match the building load profile, turn down to match the low load requirement when needed and provide an improvement in the Energy Efficiency Ratio across the entire operating range. Other requirements were a reduction in the chiller noise level to improve the working environment in the plant room and a wide operating envelope coupled with intelligent controls to allow possible variation in both flow rate and temperature. The latter was needed to leverage increased capacity from a reduced number of machines during the different installation phases and allow future enhancement to a variable primary flow system.
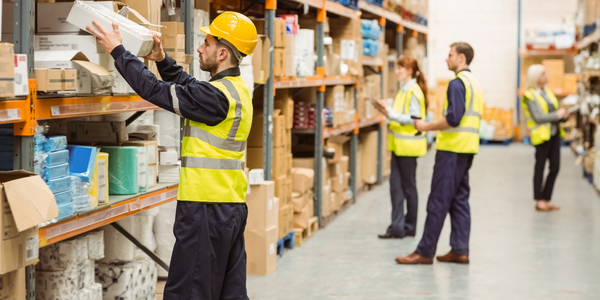
Case Study
Automated Pallet Labeling Solution for SPR Packaging
SPR Packaging, an American supplier of packaging solutions, was in search of an automated pallet labeling solution that could meet their immediate and future needs. They aimed to equip their lines with automatic printer applicators, but also required a solution that could interface with their accounting software. The challenge was to find a system that could read a 2D code on pallets at the stretch wrapper, track the pallet, and flag any pallets with unread barcodes for inspection. The pallets could be single or double stacked, and the system needed to be able to differentiate between the two. SPR Packaging sought a system integrator with extensive experience in advanced printing and tracking solutions to provide a complete traceability system.
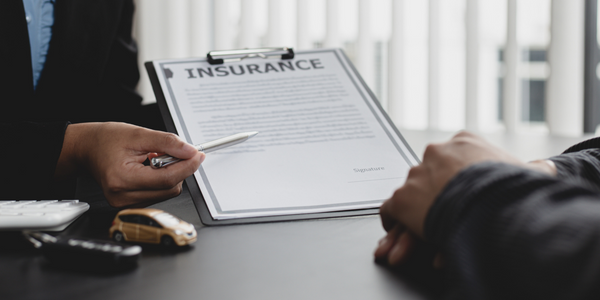
Case Study
Transforming insurance pricing while improving driver safety
The Internet of Things (IoT) is revolutionizing the car insurance industry on a scale not seen since the introduction of the car itself. For decades, premiums have been calculated using proxy-based risk assessment models and historical data. Today, a growing number of innovative companies such as Quebec-based Industrielle Alliance are moving to usage-based insurance (UBI) models, driven by the advancement of telematics technologies and smart tracking devices.
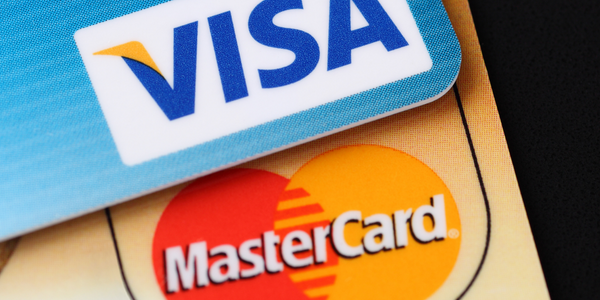
Case Study
MasterCard Improves Customer Experience Through Self-Service Data Prep
Derek Madison, Leader of Business Financial Support at MasterCard, oversees the validation of transactions and cash between two systems, whether they’re MasterCard owned or not. He was charged with identifying new ways to increase efficiency and improve MasterCard processes. At the outset, the 13-person team had to manually reconcile system interfaces using reports that resided on the company’s mainframe. Their first order of business each day was to print 20-30 individual, multi-page reports. Using a ruler to keep their place within each report, they would then hand-key the relevant data, line by line, into Excel for validation. “We’re talking about a task that took 40-80 hours each week,” recalls Madison, “As a growing company with rapidly expanding product offerings, we had to find a better way to prepare this data for analysis.”