Download PDF
Leveraging Large Scale Data Sets
Technology Category
- Analytics & Modeling - Big Data Analytics
- Analytics & Modeling - Predictive Analytics
Applicable Functions
- Business Operation
Use Cases
- Fraud Detection
Services
- Data Science Services
The Challenge
The insurance company was facing a significant challenge with claims fraud, which is estimated to cost the industry $80 billion annually in the United States alone. The existing process for detecting suspicious claims was entirely manual, relying on the judgment and experience of professional claims examiners. This approach was not scalable for a growing business and was time-consuming due to the need to pull information from multiple systems. The company had consolidated data from various sources into a Hadoop data store, which included a mix of structured and unstructured data. However, Hadoop lacked the capability for sophisticated predictive analytics, and extracting the data to an analytic server was time-consuming.
About The Customer
The customer is a global insurance company that is seeking to detect and prevent claims fraud in its Workman's Compensation business. The company has a growing business and needs a more automated approach to handle the increasing volume of claims. The company has data scientists who use R for advanced analytics, but they were facing challenges in scaling their analytics to handle Hadoop-level data volumes. The company needed a solution that could provide sophisticated predictive analytics on large datasets and enable rapid deployment of fraud detection models.
The Solution
The company implemented H2O, an open-source machine learning platform, to address its challenges. H2O was co-located in the company's Hadoop cluster, allowing analysts to discover insights in the data without extracting it or taking samples. Data scientists could interact with H2O using R, but all of the work was performed in H2O where it was deployed, in the Hadoop cluster. This approach enabled the company to leverage its large datasets for predictive analytics. When an analytics project was completed, H2O exported predictive models as Plain Old Java Objects (POJOs). These POJOs could run anywhere in the organization that Java runs, enabling rapid deployment of fraud detection models in various systems.
Operational Impact
Quantitative Benefit
Related Case Studies.
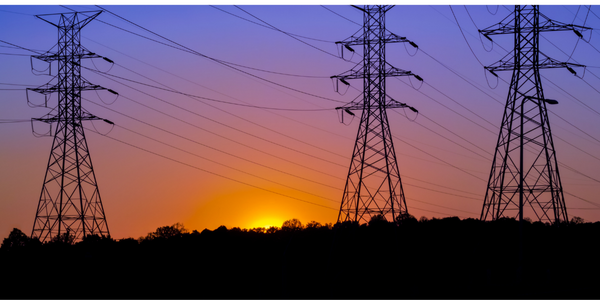
Case Study
Largest Production Deployment of AI and IoT Applications
To increase efficiency, develop new services, and spread a digital culture across the organization, Enel is executing an enterprise-wide digitalization strategy. Central to achieving the Fortune 100 company’s goals is the large-scale deployment of the C3 AI Suite and applications. Enel operates the world’s largest enterprise IoT system with 20 million smart meters across Italy and Spain.
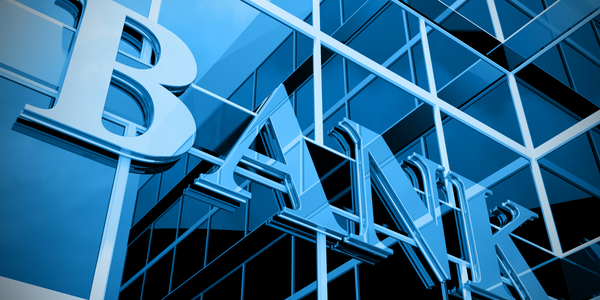
Case Study
KeyBank's Digital Transformation with Confluent's Data in Motion
KeyBank, one of the nation's largest bank-based financial services companies, embarked on a national digital bank initiative following the acquisition of Laurel Road, a digital consumer lending business. The initiative aimed to build a digital bank focused on healthcare professionals looking to refinance student loans and buy homes. A significant challenge was reducing the time to market for new products by democratizing data and decoupling systems across the IT landscape. Like many large enterprises, KeyBank had a variety of vendor applications, custom applications, and other systems that were tightly coupled to one another. New projects often required developing specific point-to-point integrations for exchanging data, which did not address the needs of other downstream systems that could benefit from the same data.
Case Study
Bank BRI: Revolutionizing Financial Inclusion in Asia with Digital Banking
Bank Rakyat Indonesia (Bank BRI), one of the largest banks in Indonesia, was faced with the challenge of increasing financial inclusion among unbanked Indonesians. The bank had an ambitious target of having 84 percent of Indonesians participating in the banking system by 2022. However, the bank's legacy technologies were proving to be a hindrance in achieving this goal. Each of the bank's products had their own public APIs, which were difficult to manage, secure, and monetize. Additionally, the process of onboarding new partners using host-to-host and VPN technology was time-consuming, taking up to six months. The bank also faced the challenge of reaching a largely rural population, with an estimated $8.3 billion in currency being held outside the banking system.
Case Study
Neobank Transformation: Enhancing Compliance and Security
The client, a leading specialist digital challenger bank based in the UK, was faced with the challenge of redesigning and rebuilding their mobile banking application. The goal was to provide a more convenient way for their customers, primarily small businesses, entrepreneurs, and consumers, to interact with their platform. Additionally, they needed to implement Open Banking, a mandatory requirement from the UK financial institution. Prior to this, the client had outsourced the development of its mobile app to other vendors. However, they needed a strong team that would take over the development completely and implement new features to improve the functionality for both the client and its customers.
Case Study
Increasing Efficiency Through Automation and Modernization for Boohoo Group
Boohoo Group, a leading British online fashion retailer, faced significant challenges due to rapid growth and acquisition of other retailers. The company needed to modernize several internal systems used for warehouse management and tax calculation to maintain efficiency. The existing systems were causing data discrepancies and issues in product tracking. Additionally, a lot of data was stored in Excel files and had to be processed manually, which slowed down operations and increased expenses. The company aimed to automate these manual processes and modernize the existing solutions to boost their efficiency.
Case Study
Aerospike Achieves One Million Writes Per Second on Google Compute Engine with Just 50 Nodes
Aerospike, an open-source, flash-optimized, in-memory NoSQL database, was looking to push the boundaries of Google's speed on Google Compute Engine. The challenge was to meet high throughput, consistently low latency, and real-time processing, which are characteristic of future cloud applications. The team at Aerospike was inspired by Ivan Santa Maria Filho, Performance Engineering Lead at Google, who demonstrated 1 Million Writes Per Second with Cassandra on Google Compute Engine. The goal was to benchmark Aerospike's product performance on Google Compute Engine and see if it could scale with consistently low latency, require smaller clusters, and be simpler to operate.