Download PDF
Mapping Dark Matter
Technology Category
- Analytics & Modeling - Machine Learning
- Analytics & Modeling - Predictive Analytics
Applicable Industries
- Aerospace
- Software
Applicable Functions
- Product Research & Development
Use Cases
- Digital Twin
Services
- Data Science Services
- Software Design & Engineering Services
The Challenge
The universe is filled with 'dark matter'—invisible, heavy matter that distorts light as it travels from distant galaxies. To create an accurate map of the universe, scientists must account for the way dark matter distorts our images of space. NASA, the British Royal Astronomical Society, and the European Space Agency sponsored the Mapping Dark Matter research competition to solve this problem. Participants were given 100,000 galaxy images, blurred to simulate the effects of dark matter. They had three months to create models to find the real shapes of galaxies; their results were scored for accuracy against known measurements.
About The Customer
The competition was sponsored by NASA, the British Royal Astronomical Society, and the European Space Agency. These organizations are at the forefront of astronomical research and space exploration. NASA, the United States' space agency, is known for its pioneering work in space missions and scientific research. The British Royal Astronomical Society is a learned society that promotes the study of astronomy, solar-system science, geophysics, and closely related branches of science. The European Space Agency is an intergovernmental organization dedicated to the exploration of space, with 22 member states. The competition attracted 72 teams from diverse fields, including handwriting recognition and string theory, highlighting the interdisciplinary nature of the challenge.
The Solution
Within the first week of the competition, Martin O’Leary, a British glaciologist, had created a solution so advanced that the White House Blog announced he had 'outperformed the state-of-the-art algorithms most commonly used in astronomy.' Meanwhile, David Kirkby and Daniel Margala, cosmologists at UC Irvine, developed an artificial neural network to recognize patterns in the galaxy images. The competition saw participation from 72 teams, including experts from fields as diverse as handwriting recognition and string theory. The winning team produced a 3x increase in accuracy over the state-of-the-art benchmark that had taken NASA decades to develop. The winners were awarded a trip to present their methods to NASA and other agencies.
Operational Impact
Quantitative Benefit
Related Case Studies.
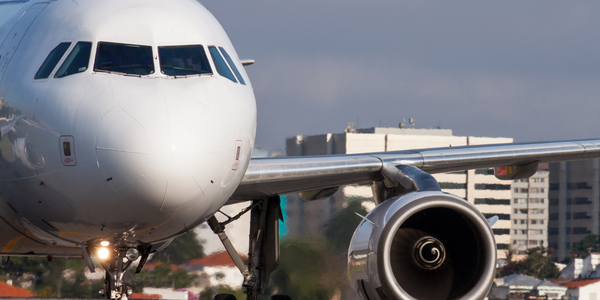
Case Study
Airbus Soars with Wearable Technology
Building an Airbus aircraft involves complex manufacturing processes consisting of thousands of moving parts. Speed and accuracy are critical to business and competitive advantage. Improvements in both would have high impact on Airbus’ bottom line. Airbus wanted to help operators reduce the complexity of assembling cabin seats and decrease the time required to complete this task.
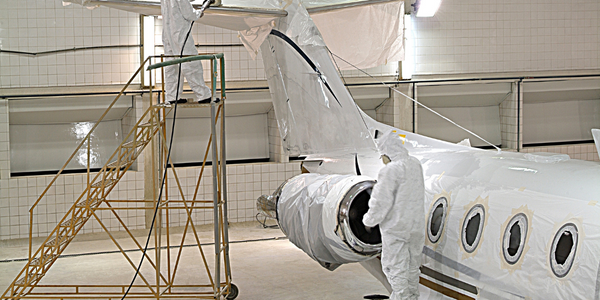
Case Study
Aircraft Predictive Maintenance and Workflow Optimization
First, aircraft manufacturer have trouble monitoring the health of aircraft systems with health prognostics and deliver predictive maintenance insights. Second, aircraft manufacturer wants a solution that can provide an in-context advisory and align job assignments to match technician experience and expertise.
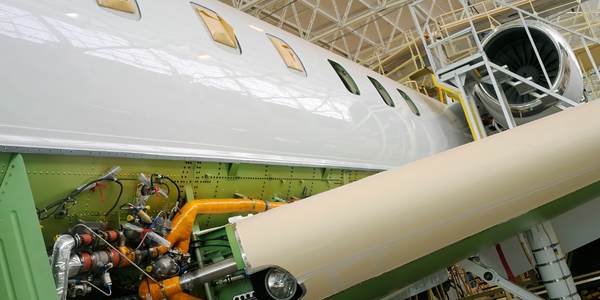
Case Study
Aerospace & Defense Case Study Airbus
For the development of its new wide-body aircraft, Airbus needed to ensure quality and consistency across all internal and external stakeholders. Airbus had many challenges including a very aggressive development schedule and the need to ramp up production quickly to satisfy their delivery commitments. The lack of communication extended design time and introduced errors that drove up costs.
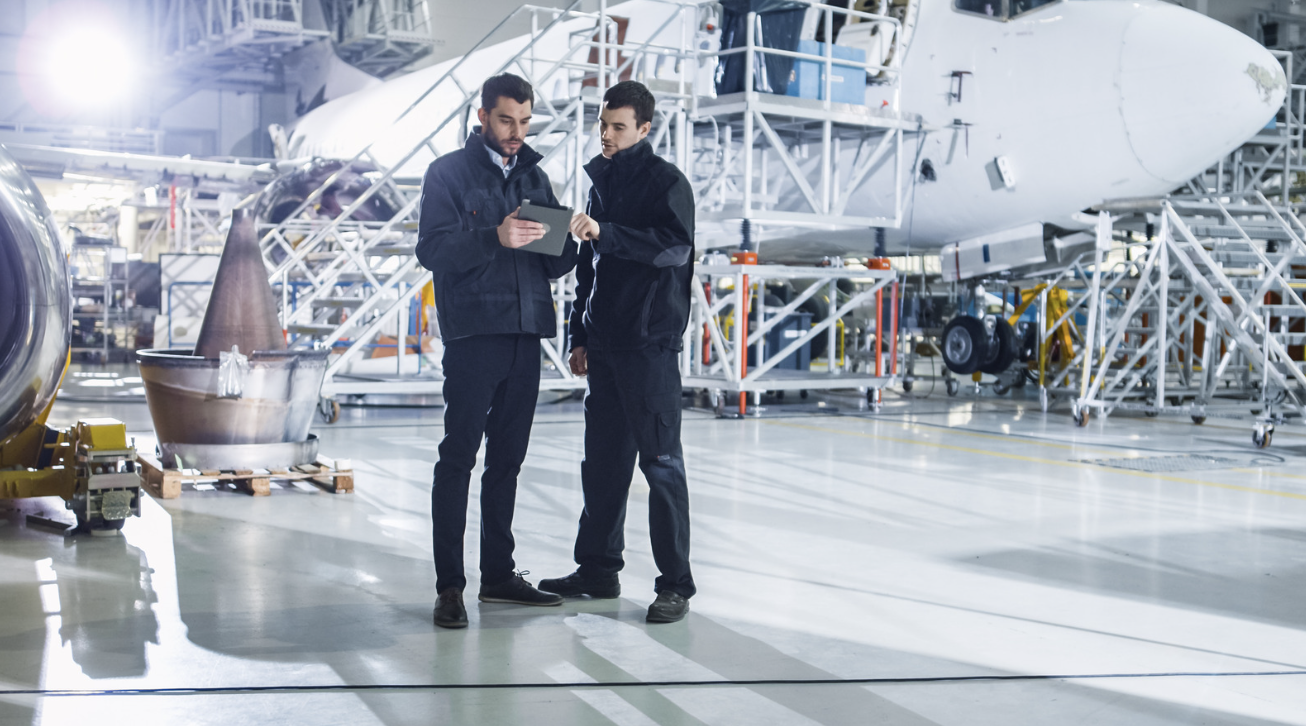
Case Study
Developing Smart Tools for the Airbus Factory
Manufacturing and assembly of aircraft, which involves tens of thousands of steps that must be followed by the operators, and a single mistake in the process could cost hundreds of thousands of dollars to fix, makes the room for error very small.
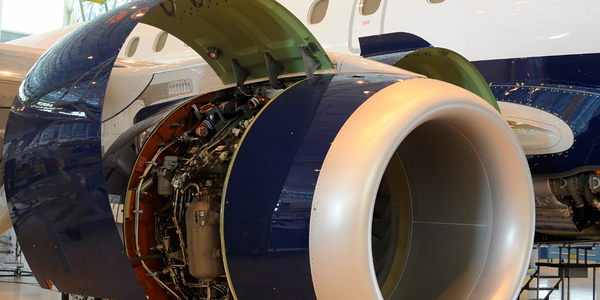
Case Study
Accelerate Production for Spirit AeroSystems
The manufacture and assembly of massive fuselage assemblies and other large structures generates a river of data. In fact, the bill of materials for a single fuselage alone can be millions of rows of data. In-house production processes and testing, as well as other manufacturers and customers created data flows that overwhelmed previous processes and information systems. Spirit’s customer base had grown substantially since their 2005 divestiture from Boeing, resulting in a $41 billion backlog of orders to fill. To address this backlog, meet increased customer demands and minimize additional capital investment, the company needed a way to improve throughput in the existing operational footprint. Spirit had a requirement from customers to increase fuselage production by 30%. To accomplish this goal, Spirit needed real-time information on its value chain and workflow. However, the two terabytes of data being pulled from their SAP ECC was unmanageable and overloaded their business warehouse. It had become time-consuming and difficult to pull aggregate data, disaggregate it for the needed information and then reassemble to create a report. During the 6-8 hours it took to build a report, another work shift (they run three per day) would have already taken place, thus the report content was out-of-date before it was ever delivered. As a result, supervisors often had to rely on manual efforts to provide charts, reports and analysis.