Download PDF
Revolutionizing Container Supply Chain Processes: A Case Study on GHD and Alteryx
Technology Category
- Analytics & Modeling - Machine Learning
- Analytics & Modeling - Predictive Analytics
Applicable Industries
- Cement
- Transportation
Applicable Functions
- Logistics & Transportation
Use Cases
- Intelligent Packaging
- Vehicle-to-Infrastructure
Services
- Data Science Services
The Challenge
The Port of Melbourne (PoM) in Australia is mandated to track all shipping containers that enter and exit every five years. This data is crucial for ensuring the right infrastructure, industrial land, planning controls, and policy settings are in place to support efficient supply chains. However, the PoM was using over 57 independent groups to track the data in more than 60 different formats. This process was not only time-consuming, requiring hundreds of hours of manual work, but also inefficient, with a forecasting rate below 30%. Furthermore, they were unable to successfully perform a match analysis. The state government in Melbourne, Australia, therefore, contracted the machine learning (ML) team at GHD, a global consulting company, to improve these container supply chain processes.
About The Customer
GHD is a global consulting company that was contracted by the state government in Melbourne, Australia. The company was tasked with improving the container supply chain processes by collecting and understanding large datasets from industry, government, and transport software service providers. The machine learning team at GHD, led by Nikita Atkins, Data Science Global Leader, was responsible for this project. They used Alteryx to narrow down 100 million shipping container and commodity records to 1.9 million with a 99.9965% match accuracy rate. They also used the Intelligence Suite to forecast and build predictive models to better estimate various aspects of the container supply chain.
The Solution
In 2019, the machine learning team at GHD, led by Nikita Atkins, developed a predictive modeling process using Alteryx. They gathered data from over 250,000 container trips from September to October 2019, standardized it, combined it, and de-duplicated 100 million records. They also included over 200 business rules before making the data consumable. The final data set of 1.9 million records was compared to the PoM data, and the data cleansing with Alteryx yielded a match of 99.9965%. Furthermore, they used Alteryx Intelligence Suite to build 10 predictive models. These models were used to estimate a container’s location, the commodities held, the capacity level of each container, and provide insight into a container’s return trip ending point and timeline. The results showed a 77% accuracy rate in tracking the trip cycle of commodities and shipping containers.
Operational Impact
Quantitative Benefit
Related Case Studies.
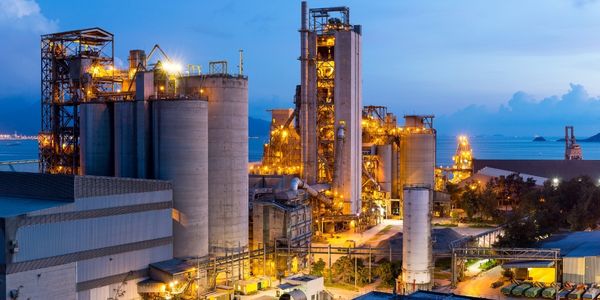
Case Study
System 800xA at Indian Cement Plants
Chettinad Cement recognized that further efficiencies could be achieved in its cement manufacturing process. It looked to investing in comprehensive operational and control technologies to manage and derive productivity and energy efficiency gains from the assets on Line 2, their second plant in India.
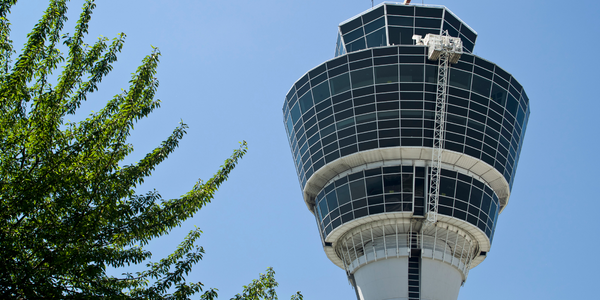
Case Study
Airport SCADA Systems Improve Service Levels
Modern airports are one of the busiest environments on Earth and rely on process automation equipment to ensure service operators achieve their KPIs. Increasingly airport SCADA systems are being used to control all aspects of the operation and associated facilities. This is because unplanned system downtime can cost dearly, both in terms of reduced revenues and the associated loss of customer satisfaction due to inevitable travel inconvenience and disruption.
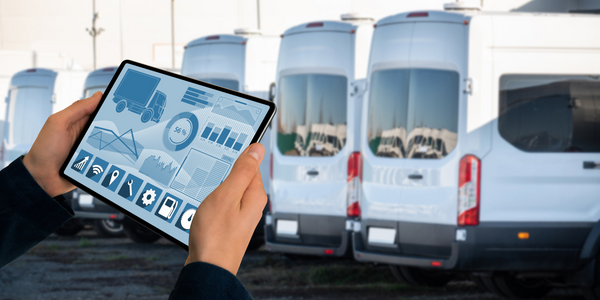
Case Study
IoT-based Fleet Intelligence Innovation
Speed to market is precious for DRVR, a rapidly growing start-up company. With a business model dependent on reliable mobile data, managers were spending their lives trying to negotiate data roaming deals with mobile network operators in different countries. And, even then, service quality was a constant concern.
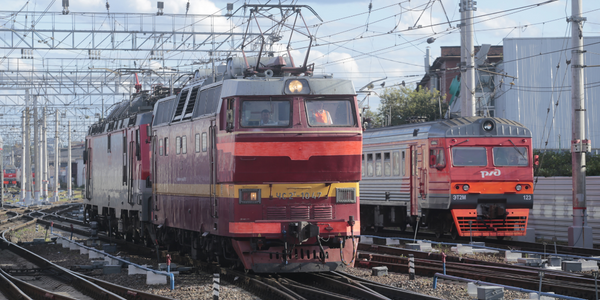
Case Study
Digitize Railway with Deutsche Bahn
To reduce maintenance costs and delay-causing failures for Deutsche Bahn. They need manual measurements by a position measurement system based on custom-made MEMS sensor clusters, which allow autonomous and continuous monitoring with wireless data transmission and long battery. They were looking for data pre-processing solution in the sensor and machine learning algorithms in the cloud so as to detect critical wear.
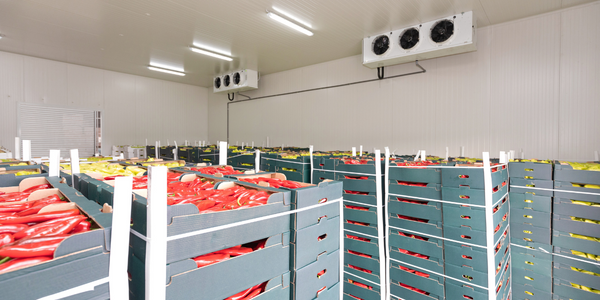
Case Study
Cold Chain Transportation and Refrigerated Fleet Management System
1) Create a digital connected transportation solution to retrofit cold chain trailers with real-time tracking and controls. 2) Prevent multi-million dollar losses due to theft or spoilage. 3) Deliver a digital chain-of-custody solution for door to door load monitoring and security. 4) Provide a trusted multi-fleet solution in a single application with granular data and access controls.
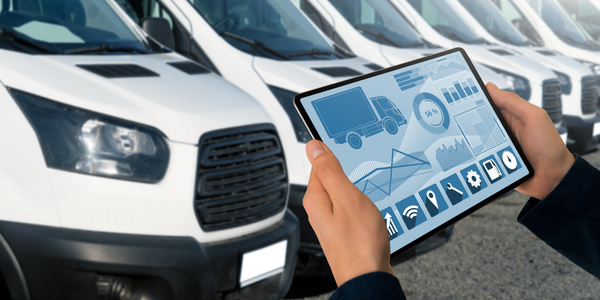
Case Study
Vehicle Fleet Analytics
Organizations frequently implement a maintenance strategy for their fleets of vehicles using a combination of time and usage based maintenance schedules. While effective as a whole, time and usage based schedules do not take into account driving patterns, environmental factors, and sensors currently deployed within the vehicle measuring crank voltage, ignition voltage, and acceleration, all of which have a significant influence on the overall health of the vehicle.In a typical fleet, a large percentage of road calls are related to electrical failure, with battery failure being a common cause. Battery failures result in unmet service agreement levels and costly re-adjustment of scheduled to provide replacement vehicles. To reduce the impact of unplanned maintenance, the transportation logistics company was interested in a trial of C3 Vehicle Fleet Analytics.