Download PDF
Velodyne's Use of Scale Nucleus for Efficient Data Annotation in 3D Lidar Technology
Technology Category
- Sensors - Autonomous Driving Sensors
- Sensors - Lidar & Lazer Scanners
Applicable Industries
- Automotive
- E-Commerce
Applicable Functions
- Warehouse & Inventory Management
Use Cases
- Object Detection
- Virtual Prototyping & Product Testing
Services
- Cloud Planning, Design & Implementation Services
- Training
The Challenge
Velodyne Lidar, a company that builds lidar sensors for safe navigation and autonomy across various industries, was facing a challenge in managing and selecting relevant training data from the large quantities of sensor data they collected. The data team found it relatively easy to classify common indoor robotics scenes as these scenarios made up a large portion of the datasets captured on their test robots. However, finding rarer scenarios, such as a warehouse employee stacking boxes on the top of a scissor lift, proved to be a difficult task. The team was in need of an out-of-the-box solution that could provide the necessary tools for efficient data selection and management.
About The Customer
Velodyne Lidar is a company that builds lidar sensors to enable safe navigation and autonomy. Their revolutionary sensor and software solutions cater to a wide range of industries, including robotics, industrial, infrastructure, and automotive. Velodyne’s lidars generate a high-quality point cloud in a wide variety of light and weather conditions, with advanced sensor-to-sensor interference mitigation, power efficiency, and thermal performance. Their customers, including e-commerce retailers and sidewalk robotics programs, use Velodyne's lidar-equipped robotics for accurate object detection, classification, and path estimation in fulfillment, delivery, and data-center operations.
The Solution
Velodyne Lidar adopted Scale Nucleus, a tool that allowed them to sort through edge cases in 3D sensor fusion data. The machine learning team captured image data along with the lidar point clouds and used the Object Autotag feature in Scale Nucleus to query, browse, and find similar images. These images were tied to 3D point clouds in a scene, enabling Velodyne to capture only point clouds of interest. Through point cloud scenes, Nucleus supported multimodal data, allowing the team to search 2D image data using the model zoo built into Nucleus, Object Autotag, and even natural language text queries to retrieve edge cases or exceptional examples in the associated 3D lidar data.
Operational Impact
Quantitative Benefit
Related Case Studies.
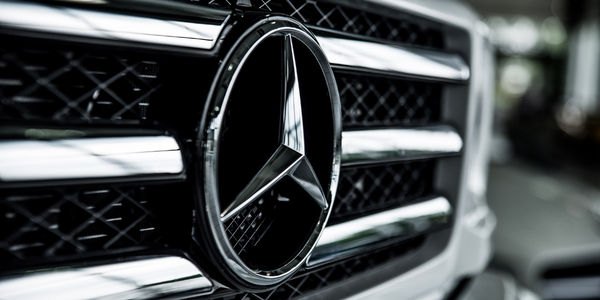
Case Study
Integral Plant Maintenance
Mercedes-Benz and his partner GAZ chose Siemens to be its maintenance partner at a new engine plant in Yaroslavl, Russia. The new plant offers a capacity to manufacture diesel engines for the Russian market, for locally produced Sprinter Classic. In addition to engines for the local market, the Yaroslavl plant will also produce spare parts. Mercedes-Benz Russia and his partner needed a service partner in order to ensure the operation of these lines in a maintenance partnership arrangement. The challenges included coordinating the entire maintenance management operation, in particular inspections, corrective and predictive maintenance activities, and the optimizing spare parts management. Siemens developed a customized maintenance solution that includes all electronic and mechanical maintenance activities (Integral Plant Maintenance).
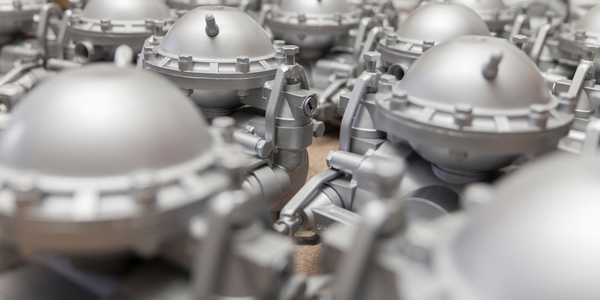
Case Study
Monitoring of Pressure Pumps in Automotive Industry
A large German/American producer of auto parts uses high-pressure pumps to deburr machined parts as a part of its production and quality check process. They decided to monitor these pumps to make sure they work properly and that they can see any indications leading to a potential failure before it affects their process.