Appen Enhances Contributor Satisfaction with ML-Driven Ticket Categorization
- Analytics & Modeling - Machine Learning
- Platform as a Service (PaaS) - Application Development Platforms
- Cement
- Education
- Procurement
- Product Research & Development
- Time Sensitive Networking
- Virtual Training
- System Integration
- Training
Appen, a leading provider of high-quality training data for AI systems, was facing challenges with its manual ticketing system. The system was inefficient, time-consuming, and prone to errors, particularly in ticket categorization. The Contributor Success team, consisting of only two members, was overwhelmed with over 11,000 tickets per month, leaving them with only seconds to resolve each issue. The manual categorization often resulted in miscategorized tickets, leading to delays in response times and dissatisfied customers. Appen needed a solution to automate its ticketing system, improve support efficiency, reduce ticket handling time, and decrease contributor churn.
Appen is a global leader in the development of high-quality, human-annotated datasets for machine learning and artificial intelligence. Appen brings over 20 years of experience capturing and enriching a wide variety of data types including speech, text, image, and video. With deep expertise in more than 180 languages and access to a global crowd of over 1 million skilled contractors, Appen partners with technology, automotive and eCommerce companies - as well as governments worldwide - to help them develop, enhance and use products that rely on natural languages and machine learning.
Provectus proposed an ML-driven solution to automate the ticketing system. The solution, based on Natural Language Processing (NLP), was designed to process ticket text and automatically categorize, prioritize, and resolve simpler tickets, while routing more complex tickets directly to the human team. The system was integrated with ZenDesk for ticket routing. The ML model, built using TensorFlow, was hosted on SageMaker’s endpoint. When a ticket was generated in ZenDesk, it was sent to Amazon API Gateway, which triggered AWS Lambda to push data to the ML model for analysis. The model classified and tagged the ticket, and the tags were then pushed back to ZenDesk via AWS Lambda and Amazon API Gateway. The system also allowed for model retraining, using data from manually reviewed tickets to improve accuracy.
Related Case Studies.
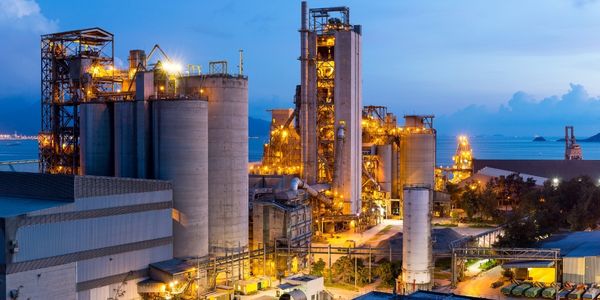
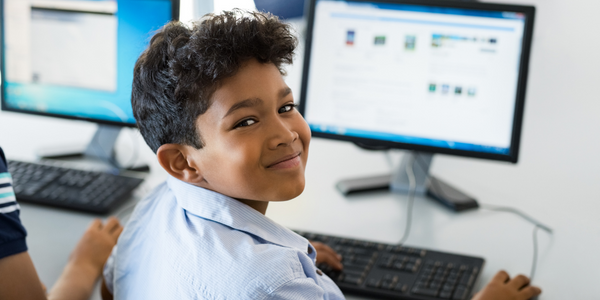
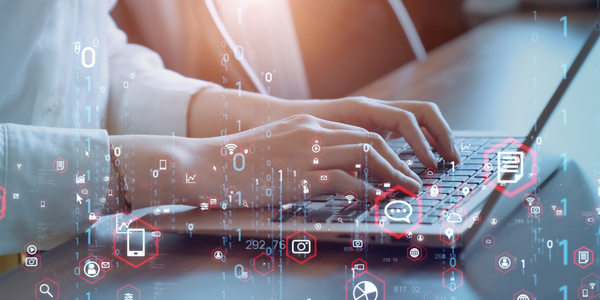
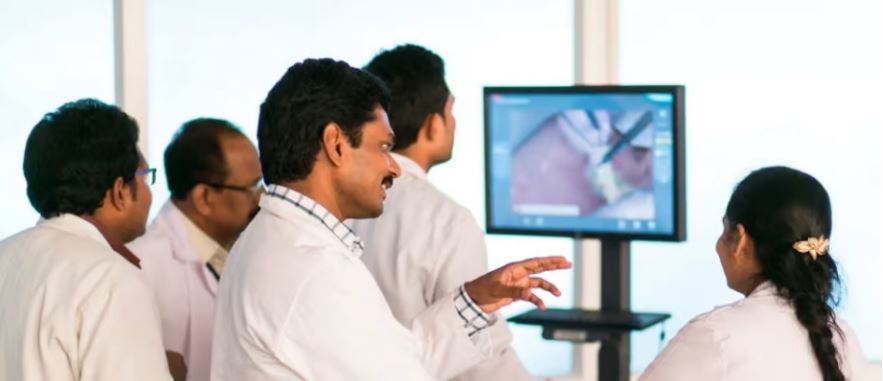
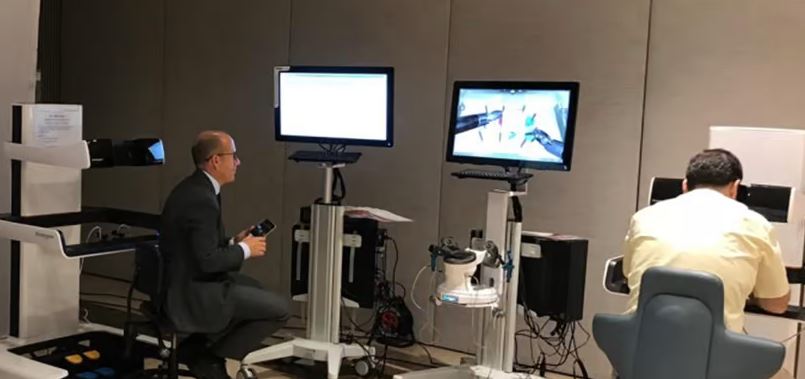