Employing Intel Deep Learning SDK Toward Bettering Image Recognition Models
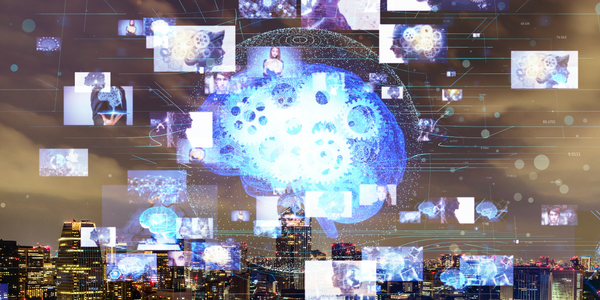
- Application Infrastructure & Middleware - Middleware, SDKs & Libraries
- Computer Vision
In this case study, the challenge explored involves LeNet*, one of the prominent image recognition topologies for handwritten digit recognition. In the case study, we dive into how the training tool can be used to visually set up, tune, and train the Mixed National Institute of Standards and Technology (MNIST) dataset on Caffe* optimized for Intel® architecture. Data scientists are the intended audience.
One of the main advantages of using the Intel Deep Learning SDK to train a model is its ease of use. As a data scientist, your focus would be more on easily preparing training data, using existing topologies where possible, designing new models if required, and train models with automated experiments and advanced visualizations. The training tool provides all of these benefits while also simplifying the installation of popular deep learning frameworks.
Related Case Studies.
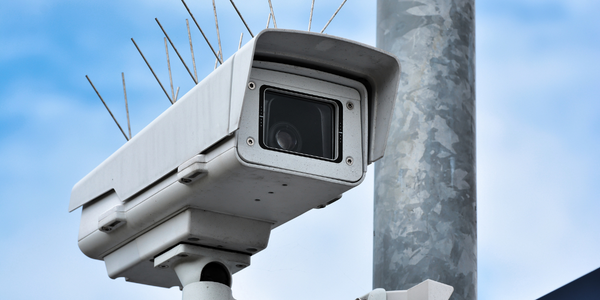
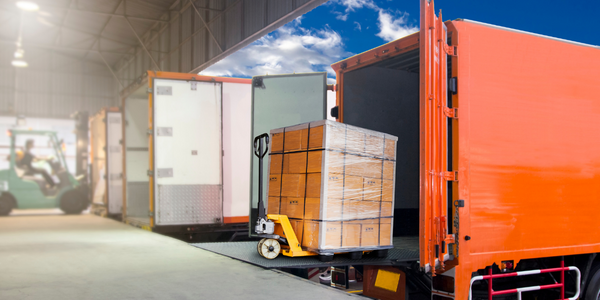
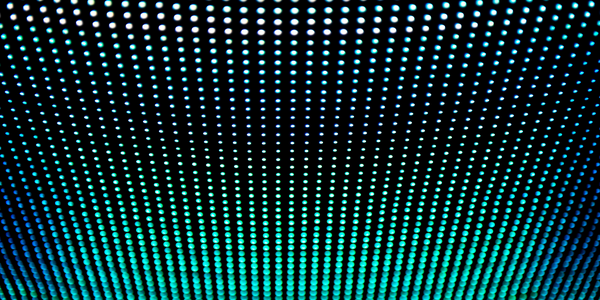
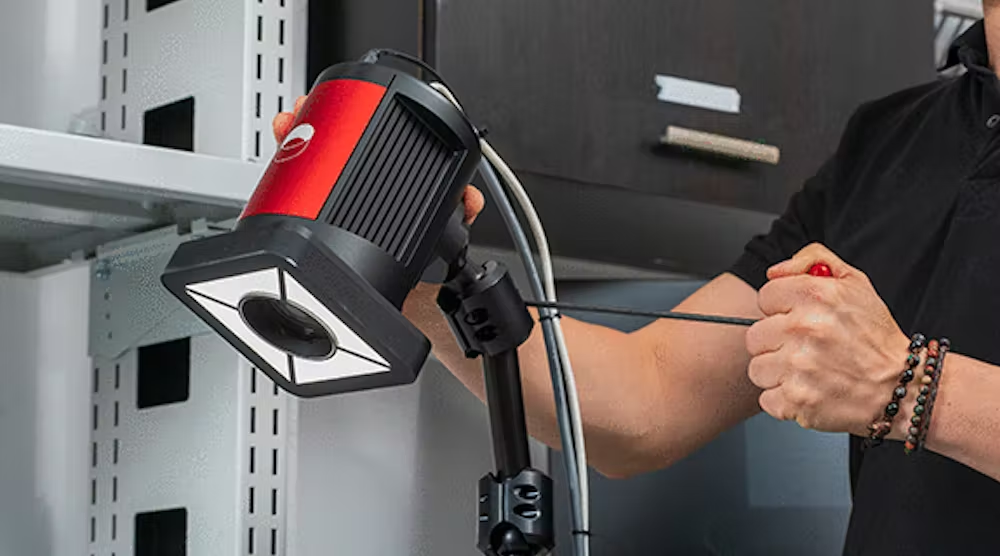
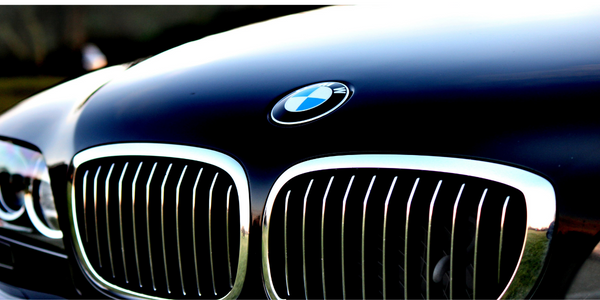