ETH Zurich: Deciphering life with the largest-ever DNA search engine
- Analytics & Modeling - Machine Learning
- Infrastructure as a Service (IaaS) - Cloud Computing
- Education
- Life Sciences
- Procurement
- Product Research & Development
- Construction Management
- Infrastructure Inspection
- Cloud Planning, Design & Implementation Services
- Data Science Services
ETH Zurich's Biomedical Informatics (BMI) Group is working on creating the world's largest-ever DNA search index by processing 4 petabytes of sequencing data. The goal is to make the world's genetic code more accessible for medical and scientific research. However, the team faced significant challenges in terms of data accessibility and processing. Despite having access to a vast amount of information in the National Center for Biotechnology Information (NCBI) repository, existing methods did not allow for the most effective use of these datasets. The team's ambitions were curtailed by their other major obstacle: efficient accessibility. Before the switch to Google Cloud, the BMI Group had to limit its operations to smaller sequencing datasets of several terabytes in size, just to keep download and processing times manageable.
ETH Zurich is a leading research institution that aims to find solutions for the defining challenges of our time, while cultivating a team of innovative and critical researchers. Its Biomedical Informatics (BMI) Group combines medicine and biology with computer science to model and make sense of molecular processes and diseases and contribute to improving treatment options together with medical collaborators. The BMI Group is working on creating the world's largest-ever DNA search index by processing 4 petabytes of sequencing data. The goal is to make the world's genetic code more accessible for medical and scientific research. The team is combining machine learning, health informatics, and bioinformatics with clinical data science, bridging medicine and biology with computer science to streamline the analysis of large genomic and medical datasets.
The solution came in the form of Google Cloud, which allowed the researchers to bring the algorithms to the data, instead of the other way around. The BMI Group uses Cloud Storage to store sequencing information and Compute Engine VM instances to process the data. The availability of this data in Google Cloud was a game changer, removing bottlenecks while fast-tracking data processing. The elasticity of cloud computing allowed for optimal parallelization of compute power, increasing the throughput. The team also built a custom server infrastructure, with one central server node distributing worker jobs across the available instances. This checkpointing feature adds resilience to the group’s operations, minimizing the risk of losing progress due to technical failures or errors. To lower the overall compute cost, the ETH team used Compute Engine Preemptible VMs, which allow any compute node to be reclaimed by the provider for other duties at any time.
Related Case Studies.
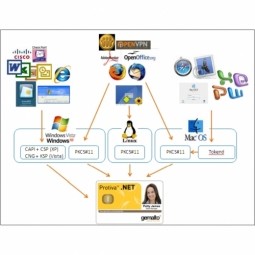
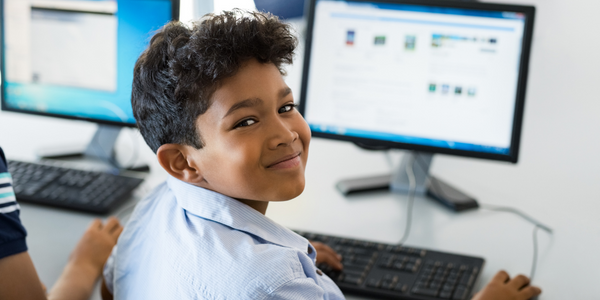
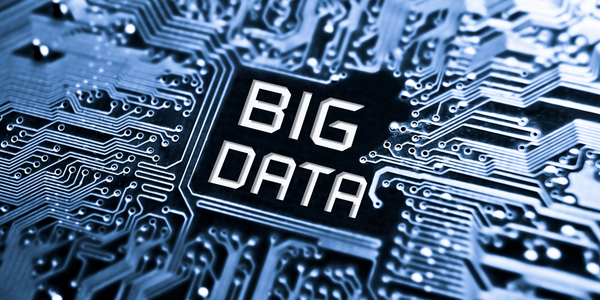
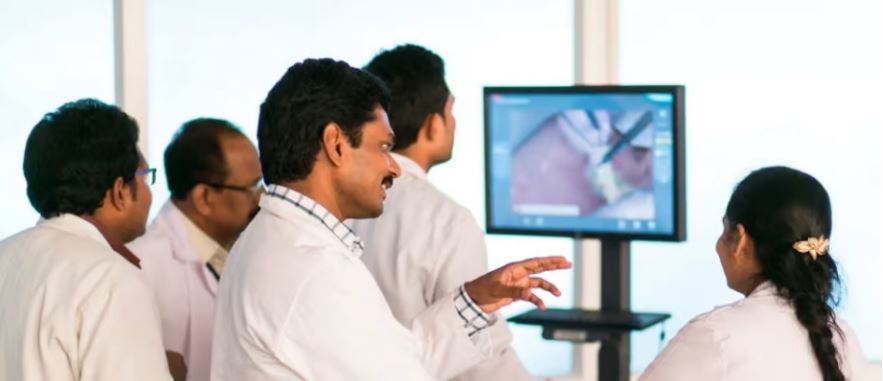
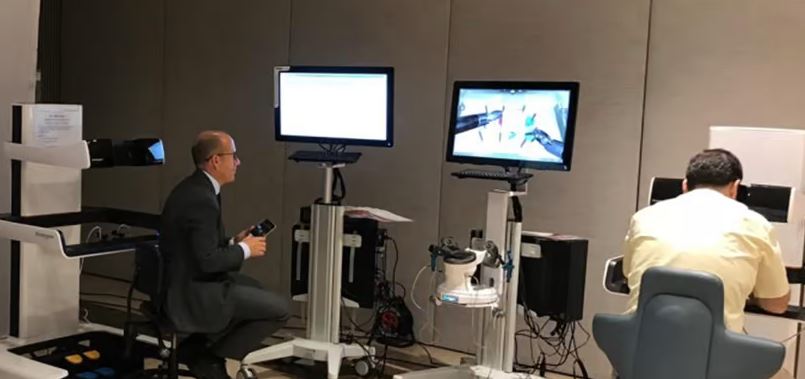
