Download PDF
Flexible and Scalable Automation
Technology Category
- Platform as a Service (PaaS) - Application Development Platforms
- Functional Applications - Remote Monitoring & Control Systems
- Platform as a Service (PaaS) - Data Management Platforms
Applicable Industries
- Agriculture
- Life Sciences
Applicable Functions
- Product Research & Development
- Quality Assurance
Use Cases
- Predictive Maintenance
- Remote Collaboration
- Remote Control
- Digital Twin
Services
- Cloud Planning, Design & Implementation Services
- Data Science Services
- System Integration
The Challenge
Syngenta was looking to improve their use of automation, and methods to make their protein production process more reproducible. Their three main pain points within their existing process were: Low uptake of automation for scientists across the Biologicals team. Automation is a powerful tool, but is difficult to use without considerable expertise. Think of trimming the edges of a poster but only being given a chainsaw; this is the feeling new automation users face, and one that Syngenta’s automation team wants to mitigate. Time-consuming processes refactoring complex liquid handler scripts. Even for expert users on Syngenta’s automation team, scaling automation for different numbers of samples is time intensive and requires careful validation in the lab to ensure the intended actions are scripted properly. This often results in reticence from scientists to invest their time in automation as they can do it quicker manually, which ultimately results in lower productivity over time. Insufficient traceability and reproducibility of experiments and data analyses. Machines improve reproducible execution of experiments, but they also increase throughput. This moved the bottleneck for Syngenta to the ability to track what has happened to each and every sample. While custom solutions were made in-house, their long term support persistence was low due to disparate documentation of the processes.
About The Customer
Syngenta is a world leading agricultural company that develops novel solutions to support farmers worldwide, helping them feed the planet and enabling them to establish sustainable, safe, and consistent agricultural practices. Scientific research forms the foundation of Syngenta, employing 5000+ scientists across chemistry, chemical engineering, biochemistry, and biology. The Biologicals Group at Syngenta develops proteins for use as biological control agents and for regulatory testing of novel chemistries. These proteins are often diverse and challenging to produce, highlighting the need for a more flexible, adaptable, and rapid approach to their production.
The Solution
Building adaptable and scalable experimental workflows. Syngenta Biologicals team used Synthace’s no-code and device-agnostic Core Elements as building blocks to construct an automated bacterial transformation pipeline. The workflow automatically scales to accommodate changes in sample numbers and other process-specific parameters such as the antibiotic to use, making automation more user-friendly and accessible. Achieving routine, data-driven decision-making. The resulting data and sample metadata were used to automatically drive decision points within workflows. Using a combination of Synthace’s Core and Data Elements, Syngenta performed in-line data processing and data-driven decision making, to automate routine analytical tasks that would have otherwise used up the precious time of expert scientists. By automating this routine data processing, the Synthace platform reduces manual data wrangling and gives scientists more time to focus on more critical and sophisticated tasks. An example workflow that incorporates automated data aggregation, in-line data processing and decision making for control of liquid handling actions and outcomes. Syngenta used Synthace to build sophisticated workflows that describe their end-to-end bacterial transformation and cryo preparation processes. The processed data was used in data-driven decision making, prompting different experimental paths based on the success or failure of the transformation process. The above example workflow encodes the following steps (1) the selection of bacterial cultures that have grown, (2) the aliquoting of the cultures into barcoded cryotubes, (3) the addition of a cryopreservative, (4) the addition of barcode information, (5) the export of cryostock information, (6) the export of information about failed transformations, and (7) the return of flagged failed transformations to the user along with information about the DNA used in the failed transformations–allowing for rapid iteration and downstream optimization.
Operational Impact
Quantitative Benefit
Related Case Studies.
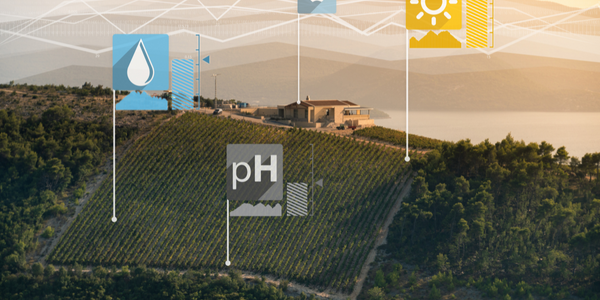
Case Study
Intelligent Farming with ThingWorx Analytics
Z Farms was facing three challenges: costly irrigation systems with water as a limited resource, narrow optimal ranges of soil moisture for growth with difficult maintenance and farm operators could not simply turn on irrigation systems like a faucet.
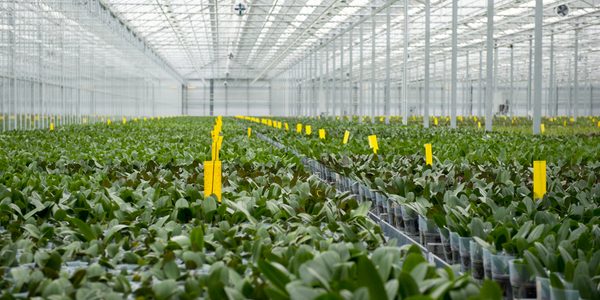
Case Study
Greenhouse Intelligent Monitoring and Control Solution
Farming Orchids is the most successful form of precision farming in Taiwan, and also the most exported flower. Orchids need a specific temperature and humidity conditions to grow and bloom, and its flowering time may not be in line with market demands, so the price collapses when there is overproduction. Therefore, some farmers began to import automated greenhouse control systems for breeding and forcing, which not only improves quality, but also effectively controls the production period and yield to ensure revenue. In 2012, an orchid farmer built a Forcing Greenhouse of about 200 pings (approximately 661 Square Meters) in Tainan, Taiwan. The system integrator adopted Advantech’s APAX-5000 series programmable automation controllers to build the control platform, coupled with Advantech WebAccess HMI/SCADA software, to achieve cloud monitoring. The staff of the orchid field can monitor important data anytime via smart phone, iPad, and other handheld devices, and control the growth and flowering conditions. System requirements: In the past, most environmental control systems of orchid greenhouses in Taiwan used PLCs (Programmable Logic Controller) with poorscalability and control, and could not be connected to the Internet formonitoring from the cloud. For advanced database analysis and networking capability, the PC platform must be adopted. Therefore, PAC Systems (Programmable Automation Controller) with both PLC programming capabilities andPC functions is a better choice.The environmental control of the Orchid greenhouse switches on and off devices like fan, shade net, cooling/heat pump, liquid flow control, water-cooling wall etc. It is controlled by a control panel of electric controllers, and is driven by a motor, to adjust the greenhouse temperature, humidity, and other environmental conditions to the set parameters.
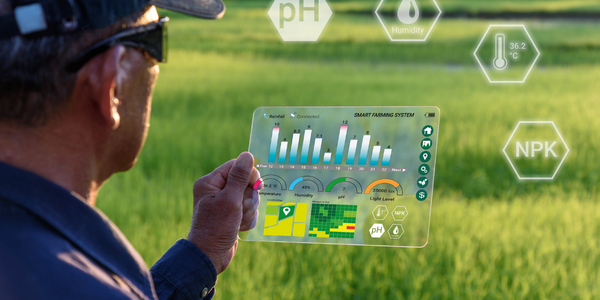
Case Study
Enabling Internet of Things Innovation in Agriculture
DigiBale, wanted to apply technology know-how and IP from implementations successfully to more agriculture sectors including cotton, forestry, sugarcane and cattle. However, farmers and growers still have worries about the connected technology.
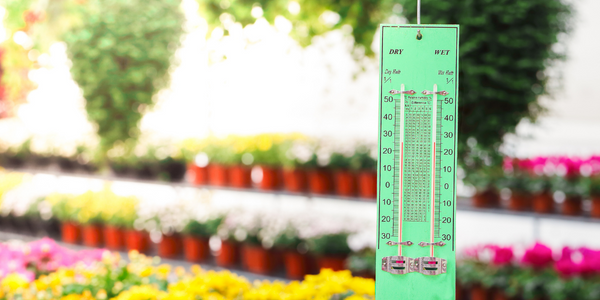
Case Study
Precision beekeeping with wireless temperature monitoring
Honeybees are insects of large economic value and provide a vital service to agriculture by pollinating a variety of crops. In addition, bees provide us with valuable products such as honey, beeswax, propolis, bee venom, etc. Monitoring of honeybee colony health, population, productivity, and environmental conditions affecting the colony health have always been exceedingly difficult tasks in apiculture. Research has shown that even small deviations (by more than 2°C) from the optimal temperatures have a significant influence on the development of the brood and the health of adult bees.