Download PDF
Improving Uptime and Asset Reliability with AI in Biomanufacturing
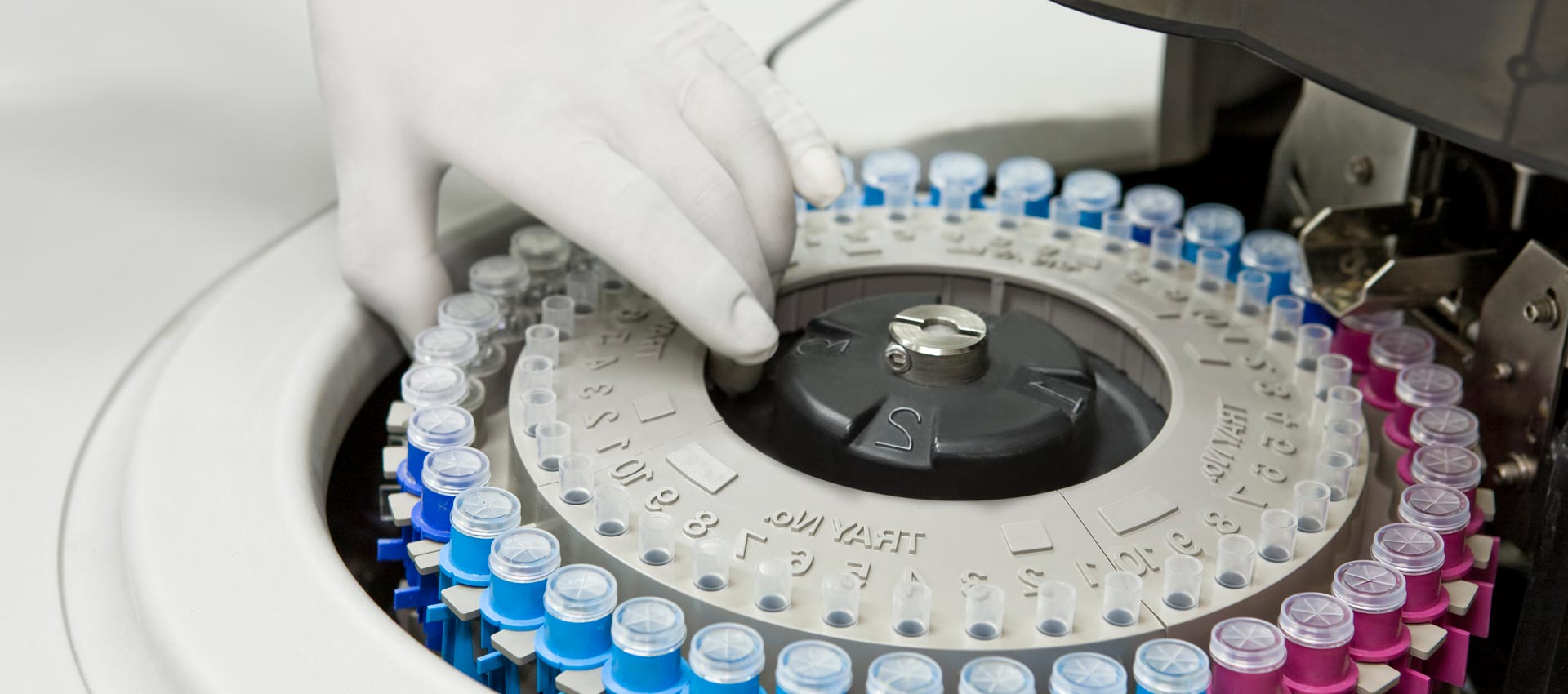
Technology Category
- Analytics & Modeling - Machine Learning
- Application Infrastructure & Middleware - Event-Driven Application
Applicable Industries
- Education
- Equipment & Machinery
Use Cases
- Predictive Maintenance
- Time Sensitive Networking
Services
- Data Science Services
- System Integration
The Challenge
Centrifuges, critical assets in the upstream stage of the biomanufacturing process, were experiencing unplanned failures that halted operations and caused significant revenue loss for a biotechnology company. The existing rules-based monitoring systems were producing an overwhelming number of false and inadequate alerts, with an average of 60 alerts a month. However, these alerts only captured 13% of actual failures and 71% of them were false alarms. The system also provided an average of only 45 minutes of lead time before a shutdown, leaving operators with a narrow window for preventative actions. The existing system was unable to flexibly incorporate new data sources or adjust preset sensor thresholds to improve the accuracy or comprehensiveness of alerts. The company needed an AI-led approach that could integrate all relevant data sources and apply advanced machine learning techniques to improve the recall and precision of failure predictions.
The Customer
Not disclosed
About The Customer
The customer is a biotechnology company with over $60 billion in annual revenue. The company serves more than 15 million patients worldwide and operates over 10 manufacturing sites. The company's operations heavily rely on centrifuges in the upstream stage of the biomanufacturing process. Unplanned centrifuge failures can halt operations and cause the company to miss a production slot entirely, reducing run rates and the number of batches produced. This can result in product being discarded from centrifuges, leading to millions in lost revenue.
The Solution
The biotechnology company chose C3 AI Reliability to implement an AI-driven approach to centrifuge monitoring. Over 12 weeks, the C3 AI team worked with subject matter experts at the company to configure the application to surface predictive and prescriptive insights for 3 centrifuges. The team ingested and unified over 6 years of historical data from 6 disparate systems, including batch reports, work orders, system alerts, sensor data, and deviation reports, to create a unified data model. Advanced machine learning techniques were applied to the unified data model, with over 500 ML model configurations tested to identify the optimal models for predicting centrifuge failures. The C3 AI Reliability user interface was configured to surface AI-based alerts and prescriptive insights, with user-friendly dashboards providing high-level site and asset KPIs, prioritized AI alerts, and details for risk investigation and mitigation.
Operational Impact
Quantitative Benefit
Related Case Studies.
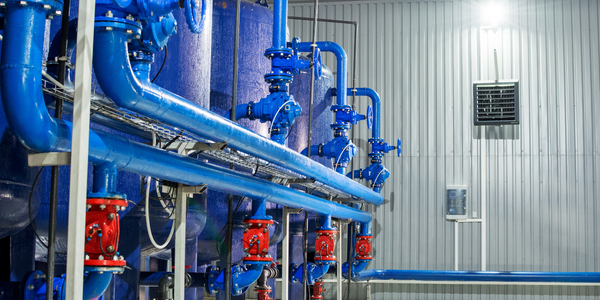
Case Study
Smart Water Filtration Systems
Before working with Ayla Networks, Ozner was already using cloud connectivity to identify and solve water-filtration system malfunctions as well as to monitor filter cartridges for replacements.But, in June 2015, Ozner executives talked with Ayla about how the company might further improve its water systems with IoT technology. They liked what they heard from Ayla, but the executives needed to be sure that Ayla’s Agile IoT Platform provided the security and reliability Ozner required.
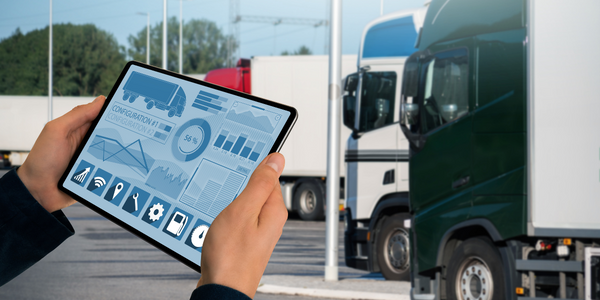
Case Study
IoT enabled Fleet Management with MindSphere
In view of growing competition, Gämmerler had a strong need to remain competitive via process optimization, reliability and gentle handling of printed products, even at highest press speeds. In addition, a digitalization initiative also included developing a key differentiation via data-driven services offers.
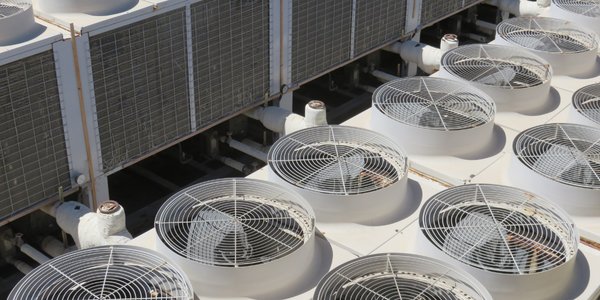
Case Study
Predictive Maintenance for Industrial Chillers
For global leaders in the industrial chiller manufacturing, reliability of the entire production process is of the utmost importance. Chillers are refrigeration systems that produce ice water to provide cooling for a process or industrial application. One of those leaders sought a way to respond to asset performance issues, even before they occur. The intelligence to guarantee maximum reliability of cooling devices is embedded (pre-alarming). A pre-alarming phase means that the cooling device still works, but symptoms may appear, telling manufacturers that a failure is likely to occur in the near future. Chillers who are not internet connected at that moment, provide little insight in this pre-alarming phase.

Case Study
Premium Appliance Producer Innovates with Internet of Everything
Sub-Zero faced the largest product launch in the company’s history:It wanted to launch 60 new products as scheduled while simultaneously opening a new “greenfield” production facility, yet still adhering to stringent quality requirements and manage issues from new supply-chain partners. A the same time, it wanted to increase staff productivity time and collaboration while reducing travel and costs.

Case Study
Integration of PLC with IoT for Bosch Rexroth
The application arises from the need to monitor and anticipate the problems of one or more machines managed by a PLC. These problems, often resulting from the accumulation over time of small discrepancies, require, when they occur, ex post technical operations maintenance.

Case Study
Robot Saves Money and Time for US Custom Molding Company
Injection Technology (Itech) is a custom molder for a variety of clients that require precision plastic parts for such products as electric meter covers, dental appliance cases and spools. With 95 employees operating 23 molding machines in a 30,000 square foot plant, Itech wanted to reduce man hours and increase efficiency.