Download PDF
Leveraging Machine Learning for Enhanced Telecommunications Services: A Case Study of Spark New Zealand
Technology Category
- Analytics & Modeling - Machine Learning
- Platform as a Service (PaaS) - Application Development Platforms
Applicable Industries
- Equipment & Machinery
- Telecommunications
Applicable Functions
- Procurement
- Product Research & Development
Use Cases
- Predictive Maintenance
- Time Sensitive Networking
Services
- Data Science Services
- Training
The Challenge
Spark New Zealand, the country's largest telecommunications and digital services company, was faced with the challenge of understanding their customers' needs at a granular level to provide better services. The company aimed to expand the number of machine learning (ML) use cases across the organization to achieve this goal. They started their journey in machine learning by trying to predict churn and understand customer preferences. However, as the number of use cases and the size of the team expanded, they faced issues with model performance and monitoring. The dynamic nature of data and the need for continuous monitoring and troubleshooting of models posed significant challenges. Checking the performance of over 50 models every week was a tedious and time-consuming task. The company needed a solution that could help them monitor these changes more effectively and proactively approach the output of models.
About The Customer
Spark New Zealand is the country's largest telecommunications and digital services company. The company provides mobile, broadband, and digital services. Over the past decade, Spark New Zealand has launched several new digital services, including Spark Health, which supports the digital transformation of the health sector. The company's senior leaders set an ambitious goal to help all of New Zealand win big in a digital world. To achieve this, they aim to understand what New Zealanders want and what their needs are at a granular level. Machine learning plays a crucial role in this endeavor. The company has over 50 models in production and a team of over 20 data scientists and machine learning engineers.
The Solution
To address these challenges, Spark New Zealand adopted the Azure ML platform for end-to-end model development and productionization. They also implemented Arize, an ML observability platform, to monitor and troubleshoot model performance. Arize was selected for its ease of use, ability to calculate and visualize data drift, and its alerting capabilities. The platform also offered tools for fairness and bias, performance tracing, and explainability. With Arize, Spark New Zealand was able to monitor models and better understand and improve them. For example, they could analyze whether a customer was leaving because of the service or some other reason, and if the features captured that behavior. If not, they could do some feature engineering to focus their attention on ways of improving the model based on feedback. The company also used Arize to tie model prediction to business return on investment or other key performance indicators (KPIs).
Operational Impact
Quantitative Benefit
Related Case Studies.
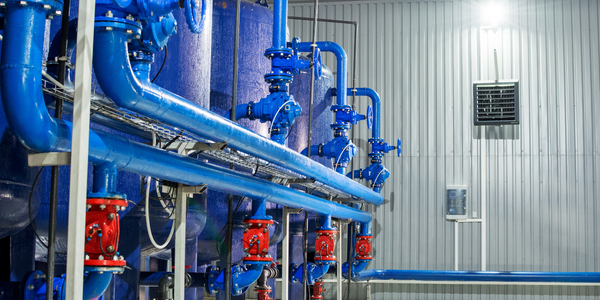
Case Study
Smart Water Filtration Systems
Before working with Ayla Networks, Ozner was already using cloud connectivity to identify and solve water-filtration system malfunctions as well as to monitor filter cartridges for replacements.But, in June 2015, Ozner executives talked with Ayla about how the company might further improve its water systems with IoT technology. They liked what they heard from Ayla, but the executives needed to be sure that Ayla’s Agile IoT Platform provided the security and reliability Ozner required.
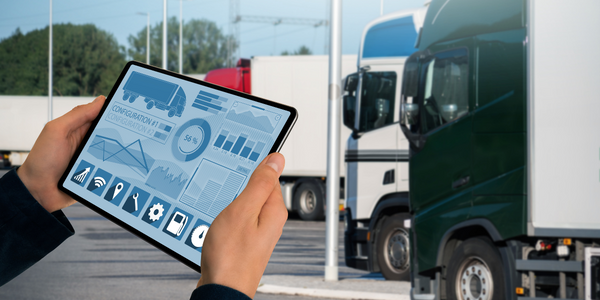
Case Study
IoT enabled Fleet Management with MindSphere
In view of growing competition, Gämmerler had a strong need to remain competitive via process optimization, reliability and gentle handling of printed products, even at highest press speeds. In addition, a digitalization initiative also included developing a key differentiation via data-driven services offers.
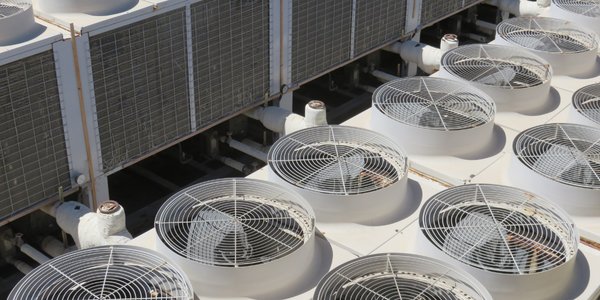
Case Study
Predictive Maintenance for Industrial Chillers
For global leaders in the industrial chiller manufacturing, reliability of the entire production process is of the utmost importance. Chillers are refrigeration systems that produce ice water to provide cooling for a process or industrial application. One of those leaders sought a way to respond to asset performance issues, even before they occur. The intelligence to guarantee maximum reliability of cooling devices is embedded (pre-alarming). A pre-alarming phase means that the cooling device still works, but symptoms may appear, telling manufacturers that a failure is likely to occur in the near future. Chillers who are not internet connected at that moment, provide little insight in this pre-alarming phase.

Case Study
Premium Appliance Producer Innovates with Internet of Everything
Sub-Zero faced the largest product launch in the company’s history:It wanted to launch 60 new products as scheduled while simultaneously opening a new “greenfield” production facility, yet still adhering to stringent quality requirements and manage issues from new supply-chain partners. A the same time, it wanted to increase staff productivity time and collaboration while reducing travel and costs.

Case Study
Integration of PLC with IoT for Bosch Rexroth
The application arises from the need to monitor and anticipate the problems of one or more machines managed by a PLC. These problems, often resulting from the accumulation over time of small discrepancies, require, when they occur, ex post technical operations maintenance.

Case Study
Robot Saves Money and Time for US Custom Molding Company
Injection Technology (Itech) is a custom molder for a variety of clients that require precision plastic parts for such products as electric meter covers, dental appliance cases and spools. With 95 employees operating 23 molding machines in a 30,000 square foot plant, Itech wanted to reduce man hours and increase efficiency.