Download PDF
Medidata Enhances Machine Data Management with Sumo Logic for Improved Clinical Trials
Technology Category
- Analytics & Modeling - Predictive Analytics
- Platform as a Service (PaaS) - Data Management Platforms
- Infrastructure as a Service (IaaS) - Hybrid Cloud
Applicable Industries
- Life Sciences
- Pharmaceuticals
Applicable Functions
- Quality Assurance
- Business Operation
Services
- System Integration
- Cloud Planning, Design & Implementation Services
- Data Science Services
The Challenge
Pharmaceutical companies and medical device manufacturers rely on Medidata’s solution to power the studies that determine whether their products are ready for delivery to the market. Medidata was generating a tremendous volume of logs from its systems, but this raw, unprocessed data was neither centralized nor efficiently scrutinized. At any given moment, Medidata’s customers are managing more than 5,000 active clinical trials on its platform. This means that, on average, every hour the company must respond to greater than 1 million transactions composed of 3,000 unique logins, up to 300,000 patient data page requests, and 150,000 Web service API calls. The company’s management set a goal to democratize access to its machine data as a critical precursor towards increasing the quality and availability of its Software as a Service (SaaS) offerings.
About The Customer
Medidata is a leading life sciences technology provider. The company’s mission is to aid pharmaceutical firms and medical equipment manufacturers in validating that their experimental drugs or devices are safe, effective, and help treat a given disease. The upshot is that Medidata plays a significant role in helping patients lead healthier lives by expediting the process of bringing a new treatment to market and then monitoring it in the population at large after approval. Customers use its cloud-based products to carry out a wide variety of tasks, such as designing studies, managing them, and analyzing results to glean significant conclusions. All of these activities are carried out within a consistent user interface on information that’s maintained by Medidata. The company offers its SaaS products to customers in the U.S. and Europe, with failover capabilities across geographies. Multiple constituents either directly utilize the platform or are represented within it, including patients of all ages, physicians, and biostatisticians, to name just a few. Medidata also gathers data directly from patients via smartphones, wearable devices, and sensors.
The Solution
Medidata incorporated Sumo Logic’s cloud native, machine data analytics platform into its technology portfolio. As part of this initiative, the company aggregated all machine data into a central location. This made it possible to derive meaningful insights from these logs via an extensive assortment of dashboards, alerts, and custom-built applications. Medidata implemented its solutions on a hybrid cloud environment that’s comprised of U.S.-based private data centers and those from Amazon Web Services (AWS). The company elected to employ distributed data centers to reduce latency, as well as comply with regulations such as the European Union (EU) requirement that patient data be stored on servers within the EU. Medidata was an early AWS adopter and now fields an extensive - and evolving - collection of its services for all aspects of its technical landscape: compute - Elastic Compute Cloud (EC2); storage - Simple Storage Service (S3), Elastic Block Store (EBS); data - Relational Database Service (RDS), Dynamo, Redshift, Kinesis; containerization - Elastic Container Service (ECS), Lambda; machine learning/analytics - Hive, Pig, R, SageMaker, SageMaker Ground Truth, Python; and management - CloudFront, Elastic Load Balancing (ELB), Application Load Balancer (ALB). The company’s application architecture is equally disparate and includes logic written in .NET, Ruby on Rails, and Java/Scala.
Operational Impact
Quantitative Benefit
Related Case Studies.

Case Study
Case Study: Pfizer
Pfizer’s high-performance computing software and systems for worldwide research and development support large-scale data analysis, research projects, clinical analytics, and modeling. Pfizer’s computing services are used across the spectrum of research and development efforts, from the deep biological understanding of disease to the design of safe, efficacious therapeutic agents.
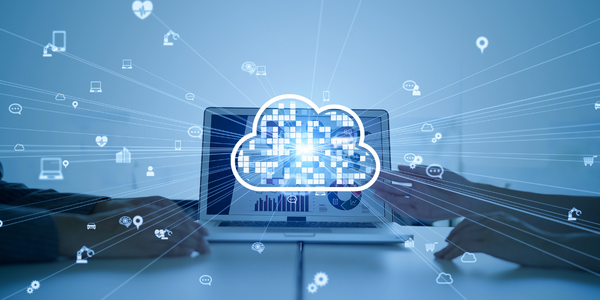
Case Study
Fusion Middleware Integration on Cloud for Pharma Major
Customer wanted a real-time, seamless, cloud based integration between the existing on premise and cloud based application using SOA technology on Oracle Fusion Middleware Platform, a Contingent Worker Solution to collect, track, manage and report information for on-boarding, maintenance and off-boarding of contingent workers using a streamlined and Integrated business process, and streamlining of integration to the back-end systems and multiple SaaS applications.
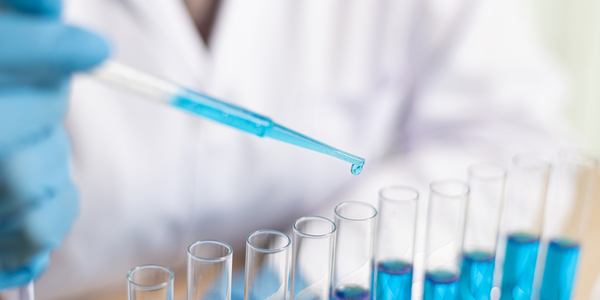
Case Study
Process Control System Support
In many automated production facilities, changes are made to SIMATIC PCS 7 projects on a daily basis, with individual processes often optimised by multiple workers due to shift changes. Documentation is key here, as this keeps workers informed about why a change was made. Furthermore, SIMATIC PCS 7 installations are generally used in locations where documentation is required for audits and certification. The ability to track changes between two software projects is not only an invaluable aid during shift changes, but also when searching for errors or optimising a PCS 7 installation. Every change made to the system is labour-intensive and time-consuming. Moreover, there is also the risk that errors may occur. If a change is saved in the project, then the old version is lost unless a backup copy was created in advance. If no backup was created, it will no longer be possible to return to the previous state if and when programming errors occur. Each backup denotes a version used by the SIMATIC PCS 7 system to operate an installation. To correctly interpret a version, information is required on WHO changed WHAT, WHERE, WHEN and WHY: - Who created the version/who is responsible for the version? - Who released the version? - What was changed in the version i.e. in which block or module of the SIMATIC PCS 7 installation were the changes made? - When was the version created? Is this the latest version or is there a more recent version? - Why were the changes made to the version? If they are part of a regular maintenance cycle, then is the aim to fix an error or to improve production processes? - Is this particular version also the version currently being used in production? The fact that SIMATIC PCS 7 projects use extremely large quantities of data complicates the situation even further, and it can take a long time to load and save information as a result. Without a sustainable strategy for operating a SIMATIC PCS 7 installation, searching for the right software version can become extremely time-consuming and the installation may run inefficiently as a result.
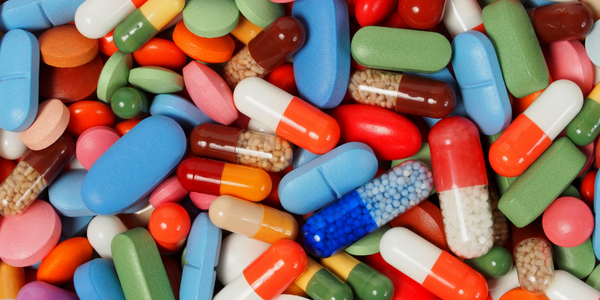
Case Study
Drug Maker Takes the Right Prescription
China Pharm decided to build a cloud-based platform to support the requirements of IT planning for the next five to ten years which includes a dynamic and scalable mail resource pool platform. The platform needed to have the following functions: all nodes support redundancy, ensuring service continuity and good user experience, simple and easy-to-use user interfaces for end users and administrators and good compatibility and supports smooth capacity expansion.
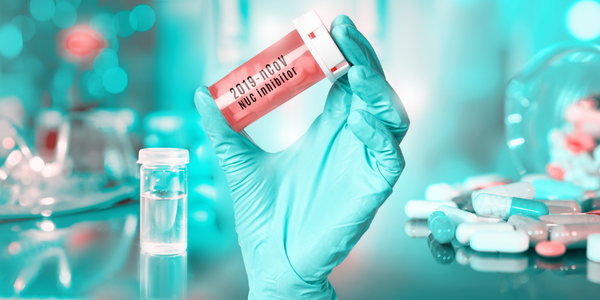
Case Study
ELI LILLY ADOPTS MICROMEDIA’S ALERT NOTIFICATION SYSTEM
Pharmaceutical production is subject to a strict set of enforced rules that must be adhered to and compliance to these standards is critically necessary. Due to the efforts of WIN 911’s strategic partner Micromedia, Lilly was able to adopt an alarm notification infrastructure that integrated smoothly with their existing workflows and emergency hardware and protocols. These raw energy sources enable the industrial process to function: electricity, WIN-911 Software | 4020 South Industrial Drive, Suite 120 | Austin, TX 78744 USA industrial steam, iced water, air mixtures of varying quality. Refrigeration towers, boilers and wastewater are monitored by ALERT. Eli Lilly identified 15000 potential variables, but limitations compelled them to chisel the variable list down to 300. This allowed all major alarms to be covered including pressure, discharge, quantity of waste water discharged,temperature, carbon dioxide content, oxygen & sulphur content, and the water’s pH.