Download PDF
NASA’s Jet Propulsion Laboratory Leverages Machine Learning for Extraterrestrial Life Search
Technology Category
- Analytics & Modeling - Machine Learning
- Sensors - Camera / Video Systems
Applicable Industries
- Cement
- Education
Applicable Functions
- Product Research & Development
Use Cases
- Intelligent Urban Water Supply Management
- Leakage & Flood Monitoring
Services
- System Integration
- Training
The Challenge
NASA’s Jet Propulsion Laboratory (JPL) is on a mission to find signs of life in our solar system, focusing on the presence of water, a vital element for life. The Ocean World Life Surveyor (OWLS) project at JPL is preparing to send a spacecraft to either Europa, a moon of Jupiter, or Enceladus, a moon of Saturn, where ice and water vapor have been discovered. The spacecraft will be equipped with microscopes to collect video data from water samples, looking for evidence of microbes. However, sending this microscopy data back to Earth is a complex and costly task due to the vast distance. Traditional compression methods are inadequate, and the energy cost of downlinking the data is extremely high. The Machine Learning Instrument Autonomy (MLIA) group at JPL faced the challenge of building a machine learning (ML) model that could identify videos most likely to contain signs of life, capture short clips, and prioritize them for downlinking back to Earth.
About The Customer
The customer in this case study is NASA’s Jet Propulsion Laboratory (JPL), a leading center for robotic exploration of the solar system. JPL has been involved in space exploration since the beginning of the Space Age and has sent spacecraft to all of the planets in the solar system. The Ocean World Life Surveyor (OWLS) project at JPL is currently preparing for the possibility of sending a spacecraft to one of the moons in our solar system where water has been discovered. The Machine Learning Instrument Autonomy (MLIA) group at JPL is responsible for developing machine learning models to aid in this exploration.
The Solution
The MLIA team decided to use traditional ML methods such as decision trees and SVMs to build a model that could fit onboard a spacecraft's computer, find and track moving particles, distinguish life-like motion from drifting or jostling, and efficiently explain its decisions. The team also worked closely with scientists to review model outputs and verify the model's accuracy. To create their algorithm, the team labeled a large, diverse set of data sourced from various field water samples and lab-grown specimens. However, creating and maintaining an in-house labeling solution was challenging. To overcome this, the MLIA team turned to Labelbox for both the training data platform and workforce. The team uploaded video datasets collected from Digital Holographic Microscopes, and the Labelbox Workforce team annotated these assets quickly, enabling the MLIA team to focus on other tasks and move the project forward much faster.
Operational Impact
Related Case Studies.
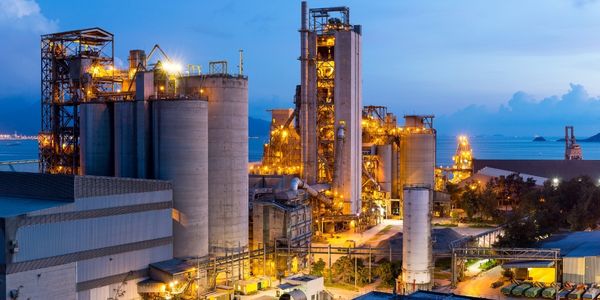
Case Study
System 800xA at Indian Cement Plants
Chettinad Cement recognized that further efficiencies could be achieved in its cement manufacturing process. It looked to investing in comprehensive operational and control technologies to manage and derive productivity and energy efficiency gains from the assets on Line 2, their second plant in India.
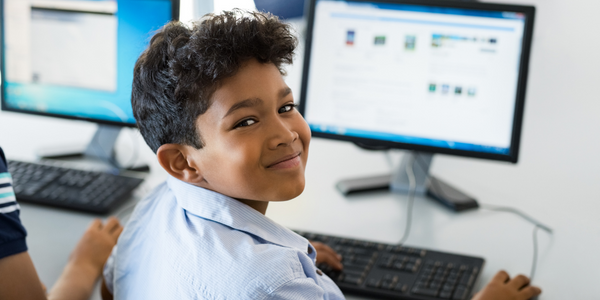
Case Study
IoT platform Enables Safety Solutions for U.S. School Districts
Designed to alert drivers when schoolchildren are present, especially in low-visibility conditions, school-zone flasher signals are typically updated manually at each school. The switching is based on the school calendar and manually changed when an unexpected early dismissal occurs, as in the case of a weather-event altering the normal schedule. The process to reprogram the flashers requires a significant effort by school district personnel to implement due to the large number of warning flashers installed across an entire school district.
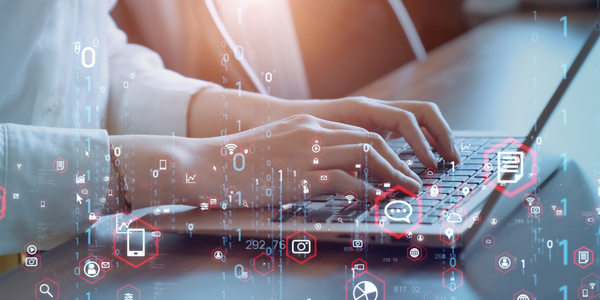
Case Study
Digital Transformation of Atlanta Grout & Tile: An IoT Case Study
Atlanta Grout & Tile, a Tile, Stone & Grout restoration company based in Woodstock, Georgia, was facing challenges with its traditional business model. Despite steady growth over the years, the company was falling behind the web revolution and missing out on the opportunity to tap into a new consumer base. They were using independent software from different vendors for each of their department information and workforce management. This resulted in a lot of manual work on excel and the need to export/import data between different systems. This not only increased overhead costs but also slowed down their response to clients. The company also had to prepare numerous reports manually and lacked access to customer trends for effective business decision-making.
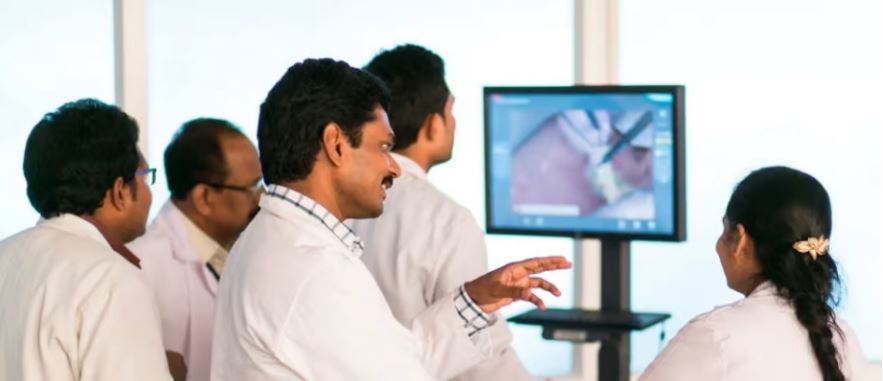
Case Study
Revolutionizing Medical Training in India: GSL Smart Lab and the LAP Mentor
The GSL SMART Lab, a collective effort of the GSL College of Medicine and the GSL College of Nursing and Health Science, was facing a challenge in providing superior training to healthcare professionals. As clinical medicine was becoming more focused on patient safety and quality of care, the need for medical simulation to bridge the educational gap between the classroom and the clinical environment was becoming increasingly apparent. Dr. Sandeep Ganni, the director of the GSL SMART Lab, envisioned a world-class surgical and medical training center where physicians and healthcare professionals could learn skills through simulation training. He was looking for different simulators for different specialties to provide both basic and advanced simulation training. For laparoscopic surgery, he was interested in a high fidelity simulator that could provide basic surgical and suturing skills training for international accreditation as well as specific hands-on training in complex laparoscopic procedures for practicing physicians in India.
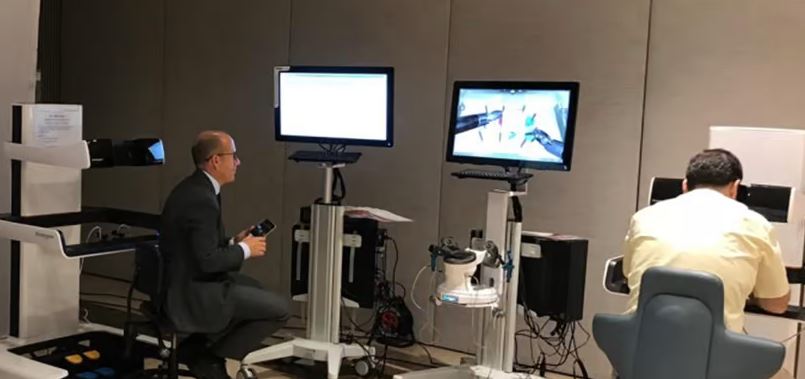
Case Study
Implementing Robotic Surgery Training Simulator for Enhanced Surgical Proficiency
Fundacio Puigvert, a leading European medical center specializing in Urology, Nephrology, and Andrology, faced a significant challenge in training its surgical residents. The institution recognized the need for a more standardized and comprehensive training curriculum, particularly in the area of robotic surgery. The challenge was underscored by two independent studies showing that less than 5% of residents in Italian and German residency programs could perform major or complex procedures by the end of their residency. The institution sought to establish a virtual reality simulation lab that would include endourological, laparoscopic, and robotic platforms. However, they needed a simulator that could replicate both the hardware and software of the robotic Da Vinci console used in the operating room, without being connected to the actual physical console. They also required a system that could provide both basic and advanced simulation training, and a metrics system to assess the proficiency of the trainees before they performed surgical procedures in the operating theater.