Optimizing Infrastructure Design with Continuum Industries' Optioneer Engine and Neptune
- Application Infrastructure & Middleware - Database Management & Storage
- Infrastructure as a Service (IaaS) - Cloud Databases
- Cement
- Construction & Infrastructure
- Product Research & Development
- Quality Assurance
- Construction Management
- Time Sensitive Networking
- System Integration
- Testing & Certification
Continuum Industries, a company in the infrastructure industry, developed a product called Optioneer to automate and optimize the design of linear infrastructure assets. However, operating the Optioneer engine presented several challenges. The engine needed to be robust enough to handle different problems fed by different customers. Moreover, the company needed to ensure that the solutions provided by the engine were accurate and agreed upon by civil engineers. The team also had to constantly improve the optimization engine without breaking the algorithm. The nature of the problem they were trying to solve presented additional challenges. They could not automatically tell whether an algorithm output was correct or not. They needed a set of example problems that was representative of the kind of problem that the algorithm would be asked to solve in production. The team initially developed a custom solution to these problems, but it proved to be extremely clunky and complex to maintain.
Continuum Industries is a company in the infrastructure industry that aims to automate and optimize the design of linear infrastructure assets like water pipelines, overhead transmission lines, subsea power lines, or telecommunication cables. Their core product, Optioneer, allows customers to input the engineering design assumptions and the geospatial data and uses evolutionary optimization algorithms to find possible solutions to connect point A to B given the constraints. The company is committed to providing accurate and reliable solutions to its customers, and to constantly improve its optimization engine to handle a variety of problems.
After realizing that they faced many of the same challenges that machine learning in production faces, the team decided to investigate the MLOps solutions that were already out there. They wanted a tool that could easily track and visualize different types of data, track both local and cloud runs in the same way, and wouldn't need to self-host or maintain the solution. After evaluating different experiment trackers, they decided to go with Neptune. Neptune was chosen because it was easy to get started, it worked great for comparing, monitoring, and debugging, it offered total flexibility in the metadata structure, and it was easy to access from anywhere including CI/CD pipelines. Neptune improved their entire workflow and sits at the core of their version of the production MLOps pipeline, executed through GitHub actions.
Related Case Studies.
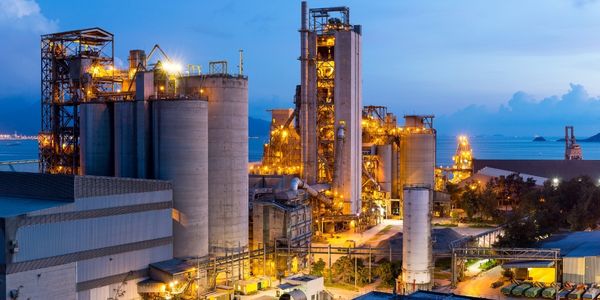
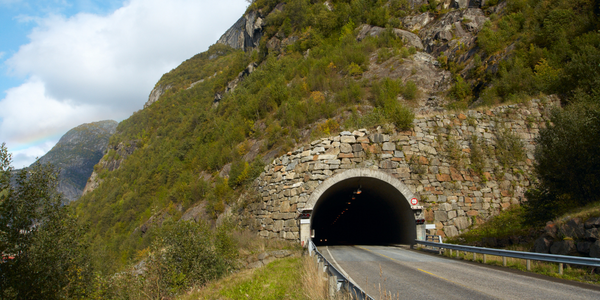
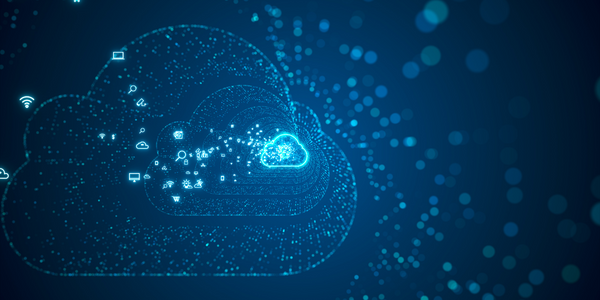
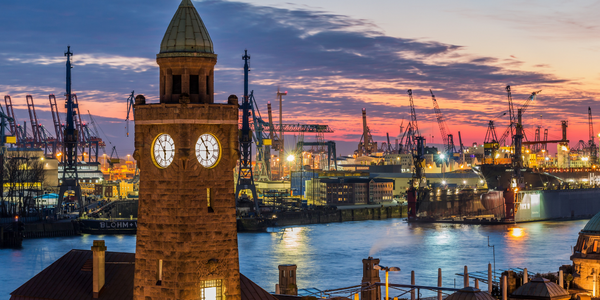
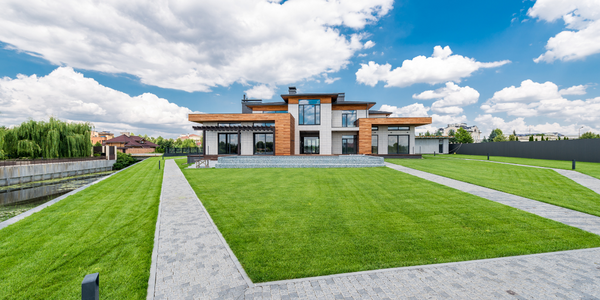