Predictive Maintenance for Building Equipment
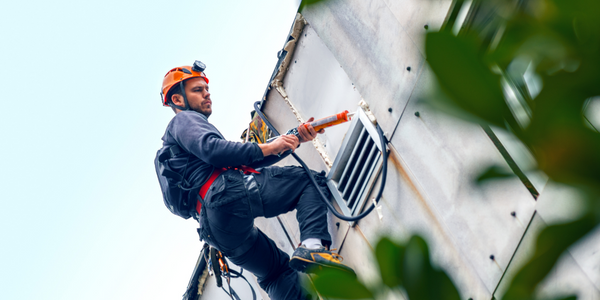
- Analytics & Modeling - Big Data Analytics
- Analytics & Modeling - Predictive Analytics
- Infrastructure as a Service (IaaS)
- Equipment & Machinery
- Maintenance
- Predictive Maintenance
Unexpected failures of building equipment can result in significant problems for facility operators. For example, refrigeration system downtimes result in expensive loss of perishables for retailers, or drugs for pharmacies. HVAC system downtimes drive need for emergency maintenance activities, and result in reduced customer satisfaction.
A global building equipment manufacturer completed a trial of C3 Predictive Maintenance in less than 1 week, demonstrating the ability to rapidly develop big data analytic predictive applications on the C3 IoT Platform. To reduce the impact of these unplanned failures, the building equipment provider completed a trial of C3 Predictive Maintenance. The trial was scoped to analyze 165 chillers with readily available sensor data. As part of the C3 IoT analytic software suite, C3 Predictive Maintenance employs machine learning-based algorithms to enhance failure prediction and diagnostic capabilities. The application augments traditional systems by continuously monitoring all instrument signals, tracking complex failure modes, and detecting operating anomalies associated with impending equipment failures for a large range of assets. In this trial, C3 IoT first loaded sensor readings with a range of widely different intervals. Significantly complicating the issue – the building equipment manufacturer did not have labels for the sensor data, and the sensor data did not match up (i.e., sensor 1 from chiller 1 did not match sensor 1 from chiller 2, and they did not know what the reading from sensor 1 represented). One of the benefits of the C3 IoT Platform, is that the data model is managed through the metadata based C3 Type System. This allowed the C3 IoT team to rapidly load data without understanding the sensor labels, and to group and label the sensors later using deep analytics. The C3 IoT Platform also has native support for time series data, allowing the team to load data with different time granularity together – but then group them and analyze them all in a consistent way – without having to write any code. C3 IoT rapidly developed 163 time series analytic inputs based on the sensor data using the platform’s time series and feature generation capabilities. These analytic inputs were then fed into a machine learning classifier. Over a single day of iteration and tuning, the predictive model results were improved by 10%. With less than a week of work, the C3 IoT team achieved failure prediction precision of 73% and a recall of 71%.
Related Case Studies.
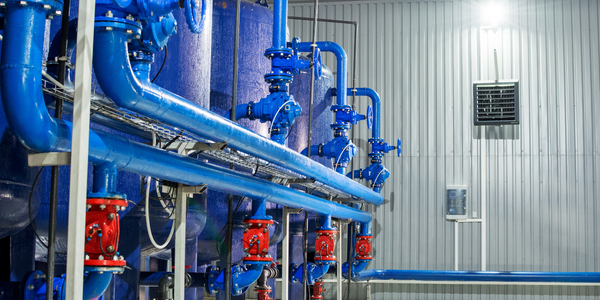
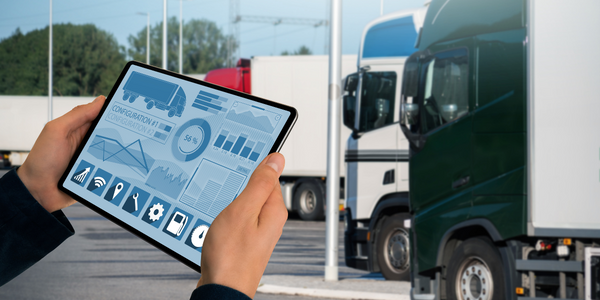
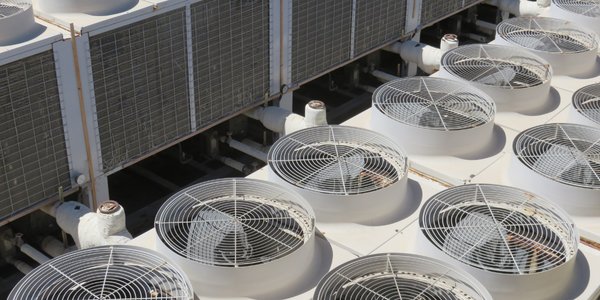


