Download PDF
Stock Price Forecasting Using Monte Carlo Simulation in Alteryx
Technology Category
- Analytics & Modeling - Digital Twin / Simulation
- Application Infrastructure & Middleware - Middleware, SDKs & Libraries
Applicable Industries
- Equipment & Machinery
- Finance & Insurance
Applicable Functions
- Logistics & Transportation
Use Cases
- Inventory Management
- Virtual Reality
The Challenge
The case study revolves around the use of Monte Carlo simulation for forecasting stock prices. The challenge was to create a sample Alteryx workflow that sources stock price data, performs analysis of the historical prices, uses these metrics to perform Monte Carlo simulations, and then analyzes the output of these simulations to drive business decision making. The aim was to provide an Alteryx template for Monte Carlo simulation-based forecasting that could be used and further enriched by the Alteryx community. The challenge also involved sourcing stock prices from Yahoo Finance, calculating daily percentage change in the stock price, preparing metrics for the simulation, and running the simulation multiple times.
About The Customer
The customer in this case study is not explicitly mentioned. However, it can be inferred that the customer is a user or member of the Alteryx community who would benefit from the Alteryx template for Monte Carlo simulation-based forecasting. The customer could be anyone interested in statistical forecasting, particularly in the context of stock price movements. They could be financial analysts, data scientists, or other professionals who use Alteryx for data analysis and forecasting. The customer would be interested in extending the workflow and customizing it to their specific use case.
The Solution
The solution involved several steps. First, historical stock prices were sourced from Yahoo Finance using the Python library 'pandas_datareader'. The daily percentage change in the stock price was calculated. Next, simulation parameters were prepared using the collected stock information. These parameters included the daily mean change in the stock price, daily volatility for the change in stock price, and the projection period for simulated prices. The Monte Carlo simulation was then performed using either the Python NumPy library or the Alteryx tool 'Simulation Sampling'. The simulation was run multiple times to build a basis for decision making. Finally, the simulation results were analyzed to drive decision making. Various reporting and data analysis tools in Alteryx were used to understand the forecasted prices. A dynamically generated report was prepared that included Price Projections Interactive chart, Histogram of the prices, and associated commentary generated dynamically using various metrics calculated in the workflow.
Operational Impact
Related Case Studies.
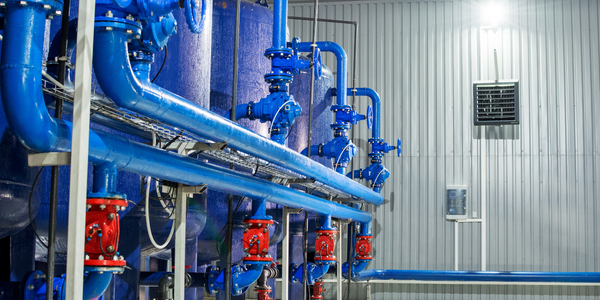
Case Study
Smart Water Filtration Systems
Before working with Ayla Networks, Ozner was already using cloud connectivity to identify and solve water-filtration system malfunctions as well as to monitor filter cartridges for replacements.But, in June 2015, Ozner executives talked with Ayla about how the company might further improve its water systems with IoT technology. They liked what they heard from Ayla, but the executives needed to be sure that Ayla’s Agile IoT Platform provided the security and reliability Ozner required.
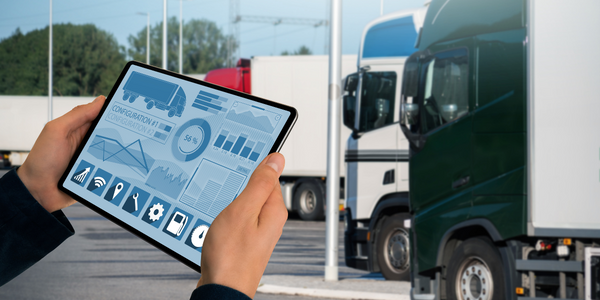
Case Study
IoT enabled Fleet Management with MindSphere
In view of growing competition, Gämmerler had a strong need to remain competitive via process optimization, reliability and gentle handling of printed products, even at highest press speeds. In addition, a digitalization initiative also included developing a key differentiation via data-driven services offers.
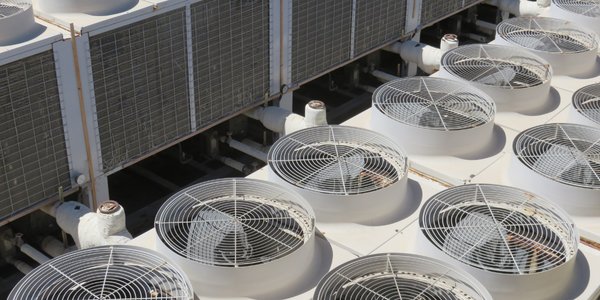
Case Study
Predictive Maintenance for Industrial Chillers
For global leaders in the industrial chiller manufacturing, reliability of the entire production process is of the utmost importance. Chillers are refrigeration systems that produce ice water to provide cooling for a process or industrial application. One of those leaders sought a way to respond to asset performance issues, even before they occur. The intelligence to guarantee maximum reliability of cooling devices is embedded (pre-alarming). A pre-alarming phase means that the cooling device still works, but symptoms may appear, telling manufacturers that a failure is likely to occur in the near future. Chillers who are not internet connected at that moment, provide little insight in this pre-alarming phase.

Case Study
Premium Appliance Producer Innovates with Internet of Everything
Sub-Zero faced the largest product launch in the company’s history:It wanted to launch 60 new products as scheduled while simultaneously opening a new “greenfield” production facility, yet still adhering to stringent quality requirements and manage issues from new supply-chain partners. A the same time, it wanted to increase staff productivity time and collaboration while reducing travel and costs.

Case Study
Integration of PLC with IoT for Bosch Rexroth
The application arises from the need to monitor and anticipate the problems of one or more machines managed by a PLC. These problems, often resulting from the accumulation over time of small discrepancies, require, when they occur, ex post technical operations maintenance.

Case Study
Robot Saves Money and Time for US Custom Molding Company
Injection Technology (Itech) is a custom molder for a variety of clients that require precision plastic parts for such products as electric meter covers, dental appliance cases and spools. With 95 employees operating 23 molding machines in a 30,000 square foot plant, Itech wanted to reduce man hours and increase efficiency.