Download PDF
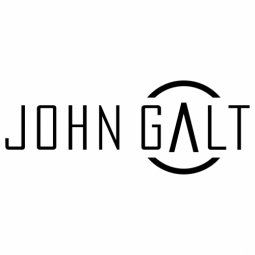
John Galt Solutions
Overview
HQ Location
United States
Year Founded
1996
Company Type
Private
Revenue
$10-100m
Employees
51 - 200
Website
Twitter Handle
Company Description
John Galt Solutions is a privately held software company that provides forecasting and supply chain planning for mid-market companies. Founded in 1996 and headquartered in Chicago, they claim more than 6,000 customers worldwide use John Galt Solutions products every day.
IoT Snapshot
John Galt Solutions is a provider of Industrial IoT platform as a service (paas), and analytics and modeling technologies, and also active in the e-commerce, food and beverage, retail, and transportation industries.
Technologies
Use Cases
Functional Areas
Industries
Services
Technology Stack
John Galt Solutions’s Technology Stack maps John Galt Solutions’s participation in the platform as a service (paas), and analytics and modeling IoT Technology stack.
-
Devices Layer
-
Edge Layer
-
Cloud Layer
-
Application Layer
-
Supporting Technologies
Technological Capability:
None
Minor
Moderate
Strong
Case Studies.
Case Study
MARS Incorporated: Leading a Global Digital Transformation
MARS Incorporated, a multinational manufacturer, faced significant challenges in digitizing and standardizing processes across its mid-markets globally. The company's reliance on legacy solutions necessitated continuous, time-consuming upgrades and made data compilation and comparison across different regions and business units difficult. Excel spreadsheets were extensively used for forecasting in smaller markets, leading to disconnected processes, siloed working environments, and increased risk of data inconsistencies and inaccuracies in demand forecasting. MARS also struggled with a lack of visibility across its midmarket footprint, scattered critical data across various systems and spreadsheets, a low degree of automation, and insufficient statistical analytics for demand planning. The absence of a standard process for demand planning made it challenging to consolidate KPIs and gain a comprehensive view of demand trends and supply chain performance.
Case Study
Wonderland Group's Supply Chain Transformation with IoT
Established in 1983, Wonderland Group, a global manufacturer and distributor of baby gear and nursery products, faced challenges due to the explosive growth of digital sales channels such as e-commerce. This shift in consumer behavior presented opportunities for the manufacturer to increase direct-to-consumer (DTC) sales, including buy online, pick-up in store (BOPIS), and warehouse DTC. As Wonderland Group’s production and channels grew, the company recognized the need to increase agility in their supply chain, enhance flexibility in distribution and warehousing, and improve visibility across the network to respond quicker to changes in demand. The team recognized the need for a powerful supply chain planning platform to translate demand into ecommerce fulfilment strategies. They sought a way to quickly understand channel-specific growth, and to combine data between consumer demand, stock on hand, and replenishment. Additionally, the company was experiencing challenges with distribution and warehousing. Warehouse space and ocean containers were severely constrained across global regions, an issue compounded by increases in both sales and product assortment.
Case Study
PING Enhances Supply Chain Planning with IoT
PING, a leading golf equipment manufacturer, faced a significant challenge in managing its supply chain due to the high level of customization it offers. The company custom-fits each club to the owner's body type, swing style, physical strength, and personal preference, resulting in over 7 million possible specification combinations for its irons alone. This complexity, coupled with the need to pre-build finished goods based on projected orders, created a profound manufacturing and supply chain planning challenge. Additionally, PING had to accommodate changing player preferences and trends in playing styles. The company's existing planning process, which relied heavily on Excel, was unable to handle the volume of SKU configurations or exploit the value of real-time sell-through information from retailers.