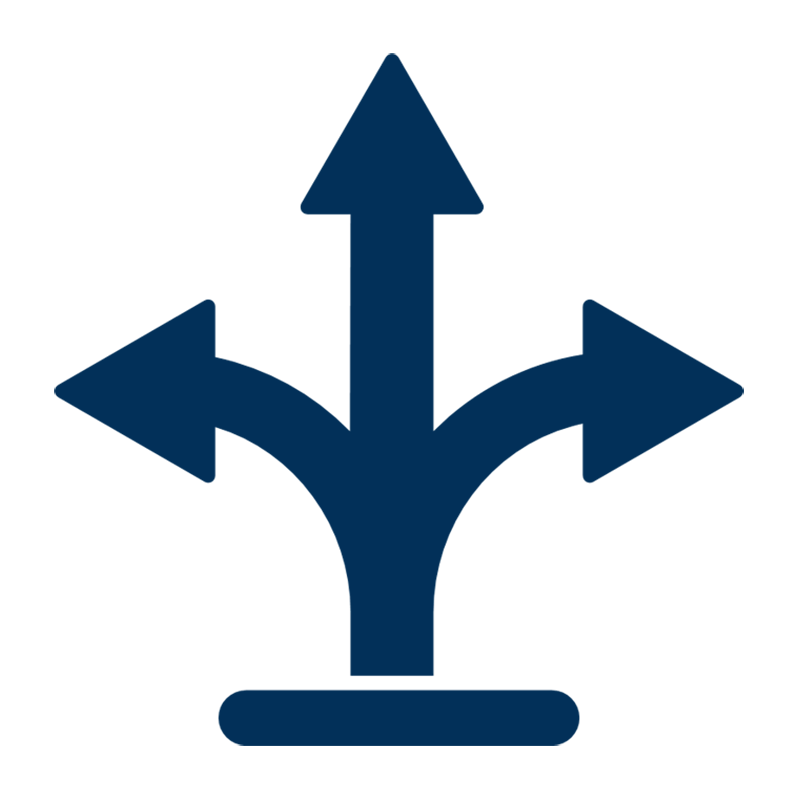
Movement Prediction
Movement prediction is a new field of deep-learning system that can do the very human task of interpreting what is happening in a photo or video and predicting the likely future movement of objects in the image. The system can express the most plausible futures by adding animations to still images, such as cars that would ultimately crash, people who might move in a street, or a train that might roll forward on its tracks. Current generations of computer vision focus on Tracking past movement or identifying objects in the present. Movement prediction provides a new direction for exploring computer vision by giving machines the ability to understand how objects move in the real world in order to predict future action.
Optimized Logistics: Movement prediction enables businesses to optimize logistics and supply chain management by forecasting the movement of goods and materials. By accurately predicting the flow of inventory, businesses can streamline operations, reduce transportation costs, and improve delivery efficiency.
Enhanced Customer Experience: Businesses can use movement prediction to enhance the customer experience by anticipating customer behavior and preferences. For example, in retail environments, predicting customer movement within a store can help optimize product placement, personalize marketing messages, and improve overall customer satisfaction.
Data Scientists and Analysts: Data scientists and analysts play a crucial role in developing and implementing movement prediction models. They are responsible for collecting and analyzing data, building predictive algorithms, and evaluating model performance to ensure accuracy and reliability.
Operations Managers: Operations managers rely on movement prediction insights to make informed decisions about resource allocation, inventory management, and workforce planning. By understanding future movement patterns, operations managers can optimize workflows, minimize bottlenecks, and improve operational efficiency.
Machine Learning Algorithms: Movement prediction models often leverage machine learning algorithms, such as regression, classification, or time series forecasting techniques. These algorithms learn from historical data to make predictions about future movements based on patterns and relationships in the data.
Big Data Platforms: Movement prediction requires processing large volumes of data from multiple sources. Big data platforms, such as Hadoop or Spark, provide the scalability and processing power needed to analyze diverse data sets and train complex prediction models.
Historical Data: Movement prediction models leverage historical data on past movements, such as location tracking data, sensor data, or transaction logs. This data is used to identify patterns, trends, and correlations that can be used to predict future movements.
Real-Time Data: Real-time data sources, such as GPS data, IoT sensors, or live video feeds, provide up-to-date information on current movements. By combining historical data with real-time observations, movement prediction models can adjust predictions in response to changing conditions and events.
Model Integration: Movement prediction models are integrated into business applications, platforms, or processes where they can generate actionable insights. This may involve developing APIs or interfaces to connect prediction models with data sources and downstream systems.
Performance Monitoring: Once deployed, movement prediction models are monitored regularly to assess their accuracy, reliability, and performance. Continuous monitoring allows for model refinement, optimization, and recalibration to ensure that predictions remain accurate over time.