Optimizing Tooling for Composite Drilling Using Deep Learning
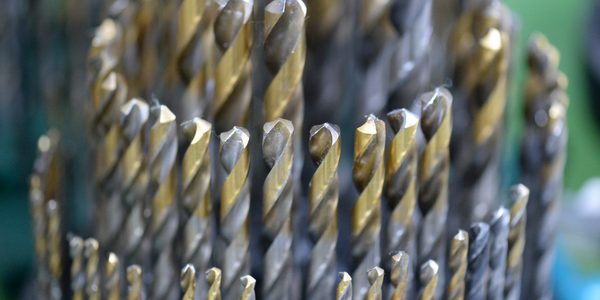
- 分析与建模 - 机器学习
- 航天
- 设备与机械
- 维护
- 产品研发
- 添加剂制造
- 时间敏感网络
- 测试与认证
- 培训
层压纤维增强聚合物 (FRP) 基复合材料在推动高性能轻质部件的行业中越来越普遍,例如航空航天。这是由于它们出色的机械性能和高度可定制的设计。尽管这种可定制性增加了设计选择,但它会对制造过程中的成本、生产力和可持续性产生负面影响。这在加工中尤其明显,在加工中会出现 FRP 零件特有的缺陷。
此处描述的工作建立了一种新的基于机器学习的方法,可以根据寿命开始性能数据预测工具寿命,从而减少实验时间和成本。该项目特别具有挑战性,因为原始数据集很稀疏,缺少 82% 的目标数据。
谢菲尔德大学先进制造研究中心 (AMRC)
谢菲尔德大学先进制造研究中心 (AMRC)是一个由世界领先的研究和创新中心组成的网络,与全球各种规模的制造公司合作。 AMRC 进行了许多具有历史意义的 CFRP 和 CFRP/金属叠层钻孔试验,以帮助该行业开发控制钻孔引起的分层的经济方法。
Intellegens 为工业研发和制造中的实际实验和过程数据问题提供了独特的机器学习解决方案。 Alchemite™ 深度学习软件基于源自剑桥大学的一种方法,可以对其他机器学习方法失败的稀疏、嘈杂的数据进行建模。它加快了合金和部件设计、配方产品开发、药物发现、增材制造和优化化学工艺等领域的创新。
Alchemite™ 是 Intellegens 的新型机器学习软件,它利用深度学习的独特见解从稀疏和嘈杂的数据中构建综合模型。在这项研究中,AMRC 提供了 55 个钻头/复合材料对的工具时间序列数据,记录了 23 个加工响应,包括孔质量指标和过程中测量值。使用直观的拖放界面,这些数据很容易上传到 Alchemite™ Analytics 软件中。
相关案例.
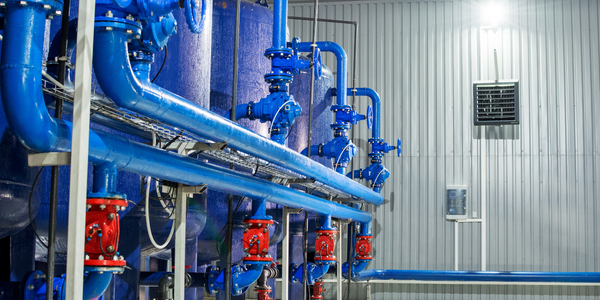
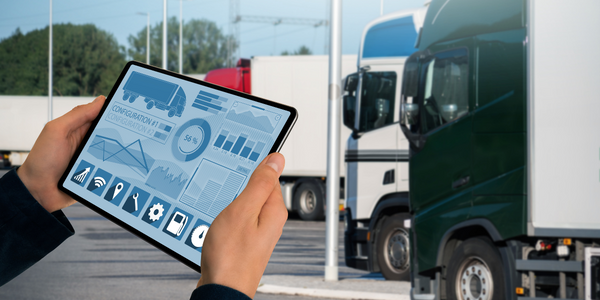
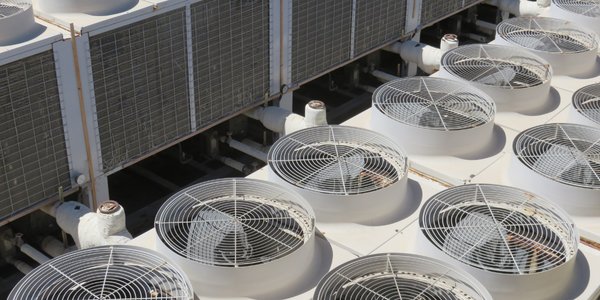


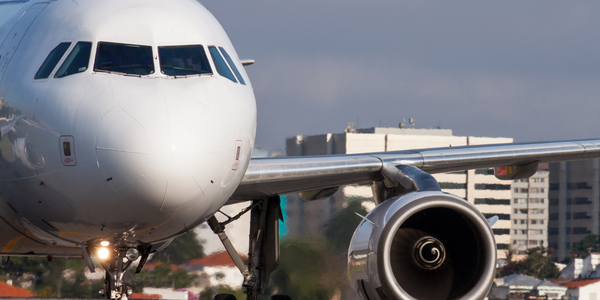