下载PDF
Redis Enterprise is Mission Critical for Mede Analytics’ eCommerce Analytics Platform
技术
- 分析与建模 - 实时分析
- 应用基础设施与中间件 - 数据库管理和存储
- 平台即服务 (PaaS) - 数据管理平台
适用行业
- 电子商务
- 医疗保健和医院
适用功能
- 商业运营
- 销售与市场营销
服务
- 云规划/设计/实施服务
- 系统集成
挑战
In order to meet the rigorous standards of next-generation applications, businesses need to adopt analytics inline to generate an intelligent customer experience. Therefore, MedeAnalytics needed to guarantee a high level of performance for its customer-facing analytics platform. In order to accomplish that, MedeAnalytics needed high-performance databases that were capable of handling a variety of application scenarios. Below are the business challenges that led MedeAnalytics to evaluate and ultimately select Redis Enterprise: Encountered the following challenges before choosing Redis Enterprise: High latency and slow response times from other databases.
关于客户
MedeAnalytics, a pioneer in healthcare analytics, develops cloud-based healthcare analytics solutions across the healthcare system. The company offers patient performance management solutions for healthcare providers in the U.S. and U.K. Mede Analytic’s intelligent cloud-based analytics platform combines data to deliver state-of-the-art analytics, all in a business context. As MedeAnalytic’s application user count was growing, their previous database couldn’t handle the new level of traffic; high latency and slow response times became an incremental issue. With Redis Enterprise, MedeAnalytics can effortlessly manage these challenges.
解决方案
MedeAnalytics decided to go with Redis Enterprise in order to keep up with industry standards. This meant having a management system capable of facilitating high-speed transactions. For its eCommerce application, MedeAnalytics uses Redis Enterprise as a primary database. Below are the key features and functionalities of Redis Enterprise employed by MedeAnalytics: Uses Redis Enterprise for the following: High-speed transactions, Search/secondary indexing. Redis Enterprise serves as a primary database. Increased their usage of Redis Enterprise for the following reasons: Application usage and user count is growing, They want to scale to multiple locations/sites, They have additional data models/uses for Redis Enterprise. Using Redis Enterprise in the following types of solutions: eCommerce.
运营影响
数量效益
相关案例.
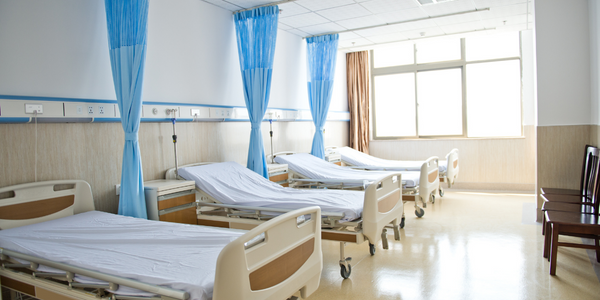
Case Study
Hospital Inventory Management
The hospital supply chain team is responsible for ensuring that the right medical supplies are readily available to clinicians when and where needed, and to do so in the most efficient manner possible. However, many of the systems and processes in use at the cancer center for supply chain management were not best suited to support these goals. Barcoding technology, a commonly used method for inventory management of medical supplies, is labor intensive, time consuming, does not provide real-time visibility into inventory levels and can be prone to error. Consequently, the lack of accurate and real-time visibility into inventory levels across multiple supply rooms in multiple hospital facilities creates additional inefficiency in the system causing over-ordering, hoarding, and wasted supplies. Other sources of waste and cost were also identified as candidates for improvement. Existing systems and processes did not provide adequate security for high-cost inventory within the hospital, which was another driver of cost. A lack of visibility into expiration dates for supplies resulted in supplies being wasted due to past expiry dates. Storage of supplies was also a key consideration given the location of the cancer center’s facilities in a dense urban setting, where space is always at a premium. In order to address the challenges outlined above, the hospital sought a solution that would provide real-time inventory information with high levels of accuracy, reduce the level of manual effort required and enable data driven decision making to ensure that the right supplies were readily available to clinicians in the right location at the right time.
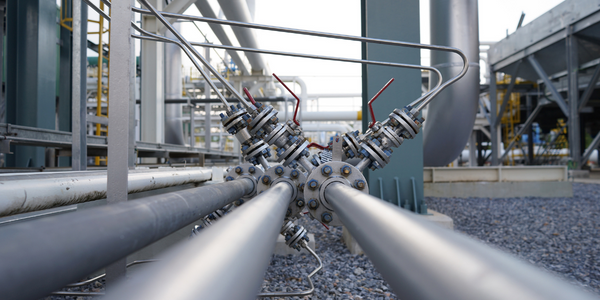
Case Study
Gas Pipeline Monitoring System for Hospitals
This system integrator focuses on providing centralized gas pipeline monitoring systems for hospitals. The service they provide makes it possible for hospitals to reduce both maintenance and labor costs. Since hospitals may not have an existing network suitable for this type of system, GPRS communication provides an easy and ready-to-use solution for remote, distributed monitoring systems System Requirements - GPRS communication - Seamless connection with SCADA software - Simple, front-end control capability - Expandable I/O channels - Combine AI, DI, and DO channels
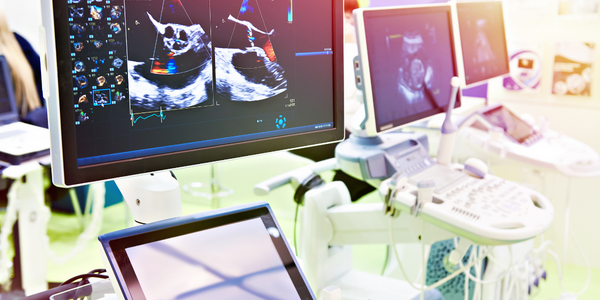
Case Study
Driving Digital Transformations for Vitro Diagnostic Medical Devices
Diagnostic devices play a vital role in helping to improve healthcare delivery. In fact, an estimated 60 percent of the world’s medical decisions are made with support from in vitrodiagnostics (IVD) solutions, such as those provided by Roche Diagnostics, an industry leader. As the demand for medical diagnostic services grows rapidly in hospitals and clinics across China, so does the market for IVD solutions. In addition, the typically high cost of these diagnostic devices means that comprehensive post-sales services are needed. Wanteed to improve three portions of thr IVD:1. Remotely monitor and manage IVD devices as fixed assets.2. Optimizing device availability with predictive maintenance.3. Recommending the best IVD solution for a customer’s needs.
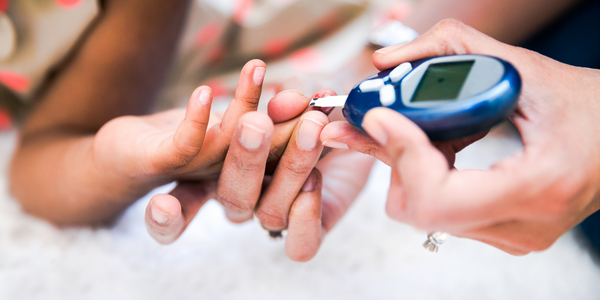
Case Study
HaemoCloud Global Blood Management System
1) Deliver a connected digital product system to protect and increase the differentiated value of Haemonetics blood and plasma solutions. 2) Improve patient outcomes by increasing the efficiency of blood supply flows. 3) Navigate and satisfy a complex web of global regulatory compliance requirements. 4) Reduce costly and labor-intensive maintenance procedures.

Case Study
Harnessing real-time data to give a holistic picture of patient health
Every day, vast quantities of data are collected about patients as they pass through health service organizations—from operational data such as treatment history and medications to physiological data captured by medical devices. The insights hidden within this treasure trove of data can be used to support more personalized treatments, more accurate diagnosis and more advanced preparative care. But since the information is generated faster than most organizations can consume it, unlocking the power of this big data can be a struggle. This type of predictive approach not only improves patient care—it also helps to reduce costs, because in the healthcare industry, prevention is almost always more cost-effective than treatment. However, collecting, analyzing and presenting these data-streams in a way that clinicians can easily understand can pose a significant technical challenge.