下载PDF
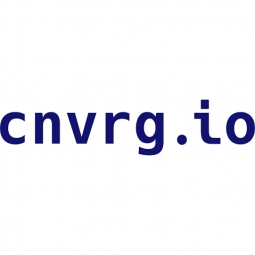
cnvrg.io
概述
公司介绍
物联网应用简介
cnvrg.io 是平台即服务 (paas), 分析与建模, 基础设施即服务 (iaas), 和 应用基础设施与中间件等工业物联网科技方面的供应商。同时致力于水泥, 建筑与基础设施, 教育, 和 设备与机械等行业。
技术栈
cnvrg.io的技术栈描绘了cnvrg.io在平台即服务 (paas), 分析与建模, 基础设施即服务 (iaas), 和 应用基础设施与中间件等物联网技术方面的实践。
-
设备层
-
边缘层
-
云层
-
应用层
-
配套技术
技术能力:
无
弱
中等
强
实例探究.
Case Study
Deploying Large-Scale Real-Time Predictions with Apache Kafka: A Playtika Case Study
Playtika, a leading Game-Entertainment company, faced significant challenges in scaling the production of real-time machine learning. With over 10 million daily active users, 10 billion daily events, and over 9TB of daily processed data, the company's existing batch and web services deployment methods were unable to scale to meet their needs or produce predictions in real-time. The REST APIs in their ML Pipelines led to service exhaustion, client starvation, handling failures and retries, and performance tuning of bulk size for batch partitioning. Playtika’s event-driven ecosystem required a solution that could support real-time streaming of their production models and scale without downtime. They also needed a solution that could integrate with various processes including Airflow and Spark, and handle bursts, peaks, and fast creation of new ML Pipelines.
Case Study
Smart Manufacturing: Seagate's Global Deployment of Defect Detection System with MLOps Automation
Seagate Technology, a global leader in data storage and management solutions, faced significant challenges in deploying a defect detection system across their global manufacturing facilities. The system had the potential to improve ROI by 300%, significantly reducing time processing defects and at a much lower cost. However, Seagate's legacy workflows made it difficult to deploy their model at scale. The team experienced low efficiency at many stages of the workflow due to manual tasks that prolonged the workflow, causing bottlenecks within the pipeline. Seagate was also experiencing low server utilization of their hybrid cloud infrastructure, as they had to run each workload separately, and did not have a mechanism in place to run different workloads on optimal machines. The team required an infrastructure to automate the pipeline components, such that the resources will be scheduled automatically, in real-time with maximum efficiency. At the production level, Seagate required advanced deployments that could serve on TensorFlow and Kafka endpoints.
Case Study
Shotgun's Rapid Transition to AI-Driven Solutions with cnvrg.io
Shotgun, a global live entertainment solution, was seeking to integrate AI into their platform to enhance their services. However, they faced several challenges. Firstly, they had limited experience in AI and needed an efficient, flexible, and intuitive AI platform that would enable their small team of engineers to deliver AI quickly. Secondly, they found most platforms to be fragmented and constrained to only using the compatible computing vendors or tools. Their first AI project was to implement a recommender system that would take user history from various sources to offer advanced recommendations for events based on user’s event and music tastes. The system also required quick recommendations with a cache solution to give users real-time and relevant recommendations. As Shotgun embarked on their AI journey, they needed a platform that was flexible and scalable, so that their team could quickly build and support new AI innovations as they grew.