Driving Efficiency in Logistics with Industrial Machine Learning
- Analytics & Modeling - Data Mining
- Analytics & Modeling - Machine Learning
- Consumer Goods
- Transportation
- Logistics & Transportation
- Warehouse & Inventory Management
- Computer Vision
- Inventory Management
- Data Science Services
- Training
The client, a Global Fortune 100 multinational engineering and technology company, was facing challenges with its existing logistics platform. The platform was used to manage goods and materials across its 400+ warehouses in over 60 countries. However, the platform had several flaws and was unsuitable for further scaling. The monolithic architecture of the platform made it inefficient and difficult to scale. The client had a vision to refactor the legacy platform but lacked the comprehensive in-house expertise to address the technical issues and make the platform more efficient and scalable. The client needed a solution that would streamline inventory management for more warehouses and improve the efficiency of internal logistics.
The client is a Global Fortune 100 multinational engineering and technology company based in Germany. The company operates in over 60 countries worldwide through a complex network of over 440 subsidiaries and regional entities. Its core operations are divided into four business sectors: Mobility, Industrial Technology, Consumer Goods, and Energy and Building Technology. Being a leading global supplier of technology and services, the client has many factories, warehouses, and suppliers, as well as a lot of raw materials and finished goods, which circulate among them.
N-iX experts helped the client transform their logistics platform by introducing a microservices architecture, DevOps best practices, and implementing advanced technologies including Machine Learning (ML), Artificial Intelligence (AI), Natural Language Processing (NLP), and computer vision. The new cloud-native infrastructure of the platform was based on Azure Kubernetes. The migration to microservices allowed for the smooth addition of new SaaS services like anomaly detection, delivery prediction, route recommendations, object detection in logistics, OCR of labels on boxes, NLP for document verification, data mining, and sensor data processing. A DevOps pipeline was built from scratch, setting up the environment for development and QA in Azure, and introducing CI/CD processes. A Computer Vision (CV) solution for docks was developed that allows contactless tracking of goods with industrial optic sensors and Nvidia Jetson devices. A multiplatform CV mobile app was also developed that covers object detection, package damage detection, OCR, and NLP for document processing.
Related Case Studies.
.png)
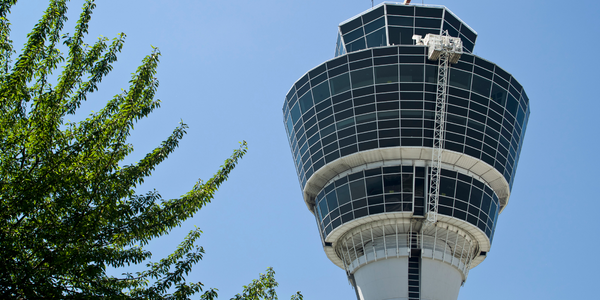
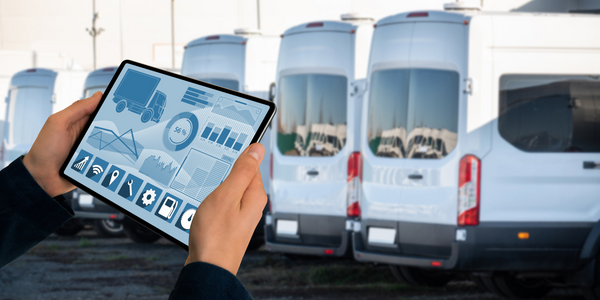
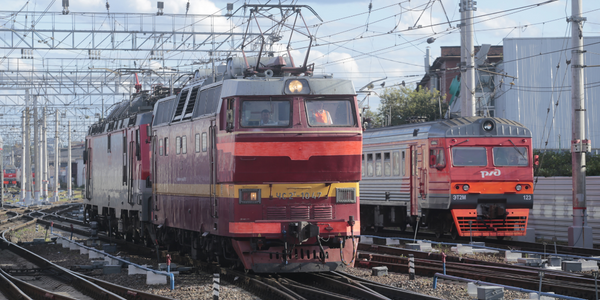
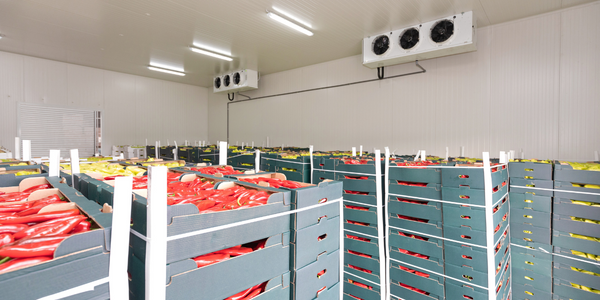