Edge AI: Deploying AI Flexibility in a Virtualized LV/ MV Substation
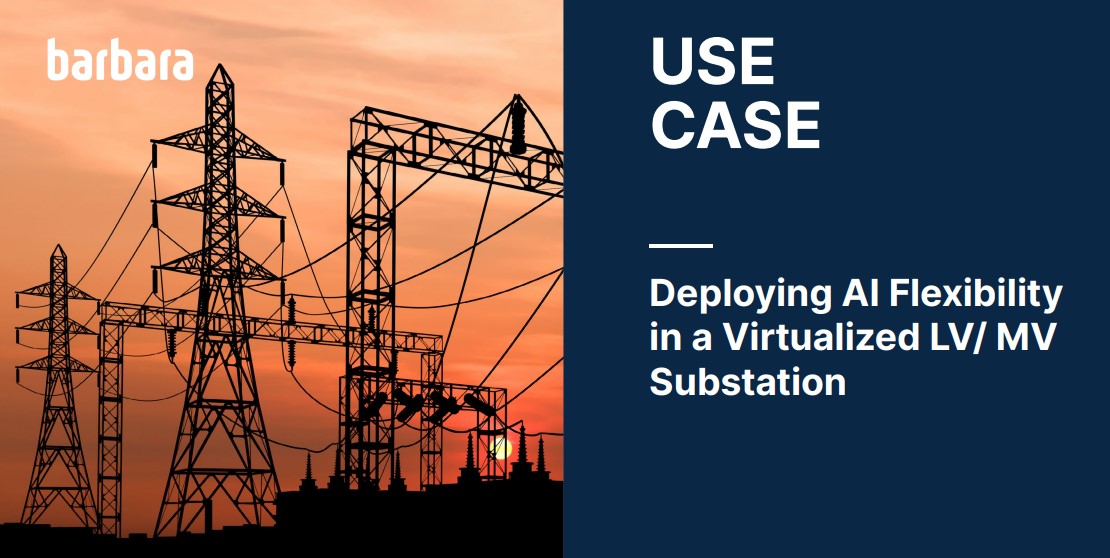
- Platform as a Service (PaaS) - Edge Computing Platforms
- Electrical Grids
- Renewable Energy
- Business Operation
- Maintenance
- Demand Planning & Forecasting
- Digital Twin
- Edge Computing & Edge Intelligence
- Software Design & Engineering Services
Cuerva a Spanish Grip Operator, was seeking to enhance grid knowledge through the implementation of the AI Energy Forecasting Model to obtain precise forecasts of user demand and energy generation.
Cuerva’s grid encompasses over 16,000 diverse supply points, making cloud-based operations intricate and susceptible to issues such as connectivity loss, delays in information transmission, and reliance on centralized infrastructure, which can result in the loss of critical data.
To tackle these challenges, the Edge technology has proven to be the sole alternative capable of addressing these issues effectively. It ensures real-time data access and operates in a decentralized manner, minimizing the impact of device failures on the overall functionality of the network.
In this successful case, we illustrate how with Barbara DSOs can implement AI directly in substations to accurately predict the demand and production values of consumers linked to the transformation center where an Edge node run by Barbara has been deployed.
CUERVA
Cuerva is a Spansih Energy Operator serving the entire energy value chain, from generation, distribution, retail and customer energy services. The business has over 140 employees and operates in 3 countries: Spain, Peru and Panama.
The company is undergoing a huge digitalisation process aimed at becoming more accessible to the end user to improve supply quality and offer new services of greater value. This has involved the rollout of full control systems over the network, from the Substation (operated by Cuerva Distribución) to the end user, covering lines, substations and sectioning, in order to obtain minute-by-minute measurements in real-time, providing full visibility over the network.
The proposed solution is based on Edge Computing nodes distributed across various Transformation Centres (TCs). More precisely the solution is composed by:
• IoT Nodes designed for distributed Edge Computing, installable in TCs.
• An Edge Node Management platform to manage these nodes efficiently.
• And Industrialised computing algorithms for:
.- Predicting active and reactive power on the consumer side to identify potential congestions and overvoltage events on the Low Voltage (LV) grid.
.- Evaluating the flexibility assets within Cuerva’s grid and creating models for them.
.- Determining the flexibility requirements of the system
Related Case Studies.
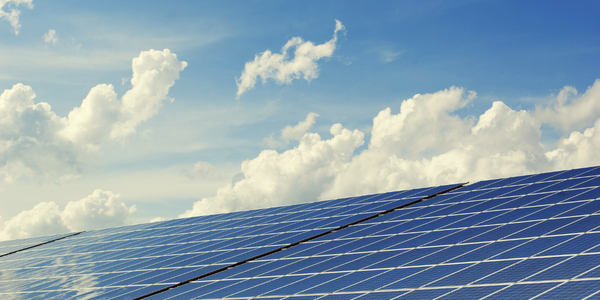
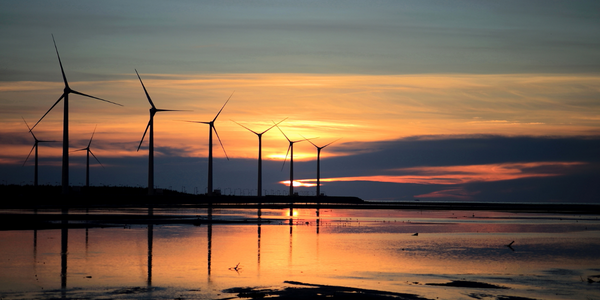
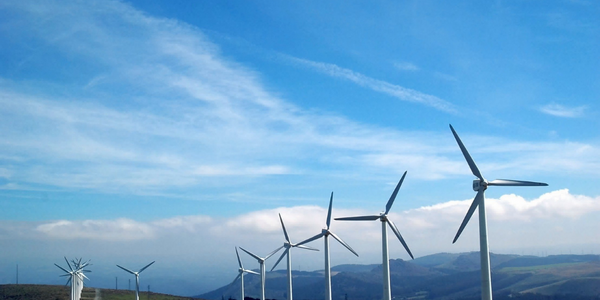
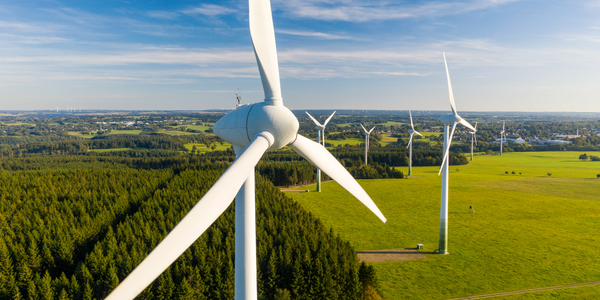
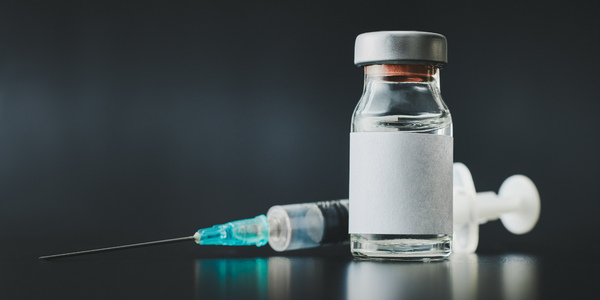
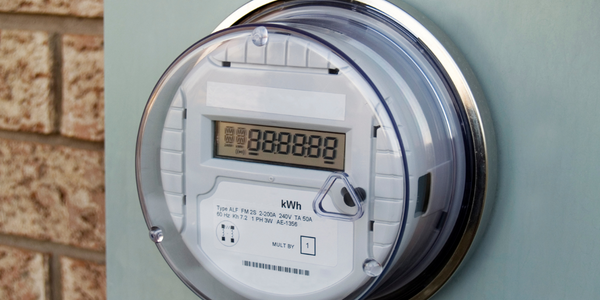