Increasing Market Reach with Intelligent Traffic Management Solution: A Case Study on Redflex
- Cybersecurity & Privacy - Intrusion Detection
- Sensors - Autonomous Driving Sensors
- Education
- Transportation
- Logistics & Transportation
- Computer Vision
- Visual Quality Detection
- System Integration
- Training
Redflex, an Australian-based company that develops intelligent transport solutions (ITS) for government, police, and traffic departments, wanted to increase their market presence with a new solution for traffic management. The company needed to validate their product idea and develop an intelligent transport solution with high detection accuracy. The main task was to develop advanced models to detect a certain anomaly based on the image received from the client's cameras. The company decided to increase their presence in Europe and expand the portfolio of their services. Therefore, they needed to create a next-generation solution for traffic management, taking into account the traffic rules and related policies of different countries.
Redflex is an Australian-based company that develops intelligent transport solutions (ITS) for government, police, and traffic departments. The company aims to make cities greener, safer, and smarter. It has a large presence in the USA, the UK, as well as Asia and the Middle East. Redflex offers a range of safety solutions - including speed cameras, red-light cameras, and school bus stop-arm cameras that deter dangerous driving. The company wanted to increase their market presence with a new solution for traffic management and needed to validate their product idea and develop an intelligent transport solution with high detection accuracy.
N-iX, a technology solutions provider, set up a team of computer vision specialists and started the project with a Proof of Concept (PoC). They worked on two main tasks: person detection (capturing distracted driving behavior) and seat belt fastening detection/violation classification. With the help of Computer Vision and Deep Learning, they built a PoC and offered several options for neural networks with different approaches to network training. This allowed the client to identify a person behind the wheel. Another PoC was developed to capture specific behaviors of distracted driving, such as talking or texting on the phone, eating and drinking, etc. The solution developed performs at approximately 88% detection accuracy. The next PoC focused on capturing distracted driving behavior, which divert attention away from driving. The system includes automatic Number Plate Recognition (ANPR) and general identification of vehicles based on Artificial Intelligence (AI) algorithms. The model developed supports a real-time stream (30 FPS) that helps generate fines in real-time based on captured data and specific violations. The solution provides a 91% person detection accuracy both at night and during the day.
Related Case Studies.
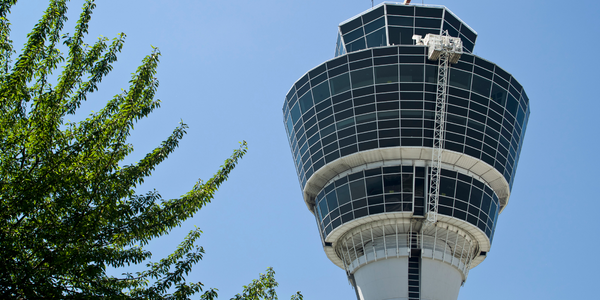
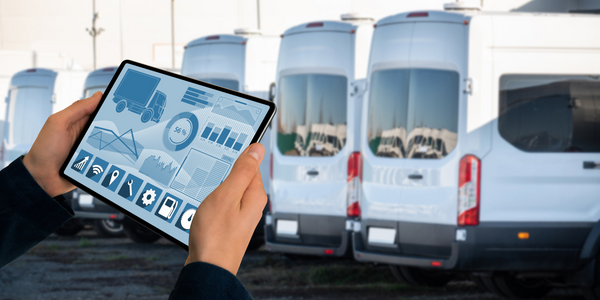
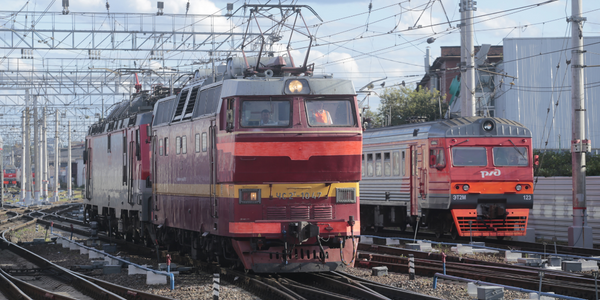
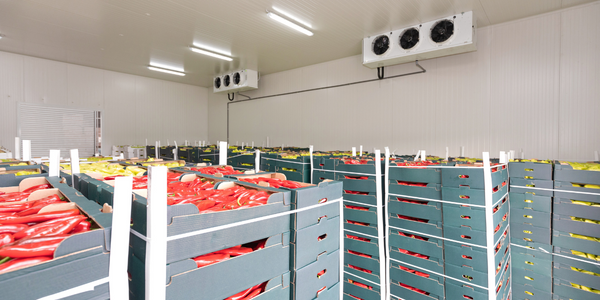
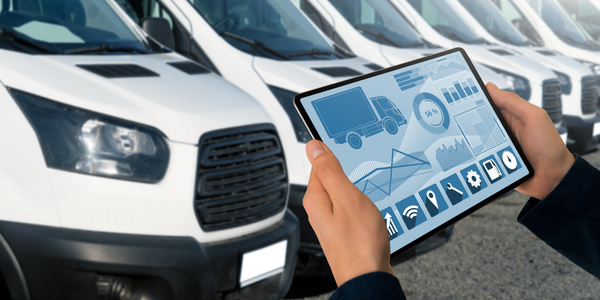
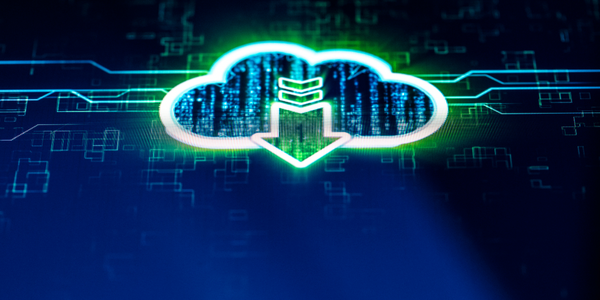