Download PDF
IoT Implementation in PIAGGIO: Enhancing Productivity and Engineering Efficiency
Technology Category
- Cybersecurity & Privacy - Identity & Authentication Management
- Sensors - Level Sensors
Applicable Industries
- Automotive
- Transportation
Applicable Functions
- Product Research & Development
Use Cases
- Autonomous Transport Systems
- Transportation Simulation
Services
- Testing & Certification
The Challenge
PIAGGIO, a leading manufacturer of motorized two-wheeled vehicles, was facing a significant challenge in analyzing complex geometry under test conditions. The company lacked information about the critical areas, which made the model require a high level of detail everywhere. The detailed features, such as rounds, could not be neglected. This situation posed a significant challenge as it required a meticulous and time-consuming process to ensure the accuracy and reliability of the test results.
About The Customer
Founded in 1884, PIAGGIO is a world-leading manufacturer of motorized two-wheeled vehicles and a consolidated leader in the European market. The company operates with several brands, including PIAGGIO, GILERA, VESPA, DERBI, and PUCH, catering to a wide range of mobility demands. Innovation, creativity, and design are the core values that guide PIAGGIO as it faces the challenges of the global market and strives to meet customer expectations.
The Solution
To address this challenge, PIAGGIO imported geometry from Pro/E into the ANSYS Workbench. The Workbench detected a few geometric singularities due to CAD modeling errors. However, only a couple of geometry updates provided the fix. The powerful Workbench tet mesher quickly filled the geometry with about a million nodes and 600K elements. The iterative solver found the solution in about 2 hours on a standard Intel workstation. The most stressed region, which was extremely localized, was sub-modeled and nonlinearly analyzed inside ANSYS. This process involved inserting plastic behavior to evaluate the maximum level of plastic deformation.
Operational Impact
Quantitative Benefit
Related Case Studies.
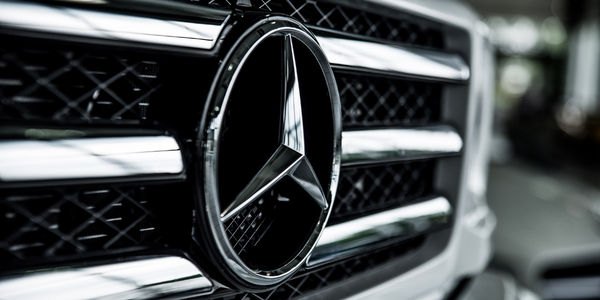
Case Study
Integral Plant Maintenance
Mercedes-Benz and his partner GAZ chose Siemens to be its maintenance partner at a new engine plant in Yaroslavl, Russia. The new plant offers a capacity to manufacture diesel engines for the Russian market, for locally produced Sprinter Classic. In addition to engines for the local market, the Yaroslavl plant will also produce spare parts. Mercedes-Benz Russia and his partner needed a service partner in order to ensure the operation of these lines in a maintenance partnership arrangement. The challenges included coordinating the entire maintenance management operation, in particular inspections, corrective and predictive maintenance activities, and the optimizing spare parts management. Siemens developed a customized maintenance solution that includes all electronic and mechanical maintenance activities (Integral Plant Maintenance).
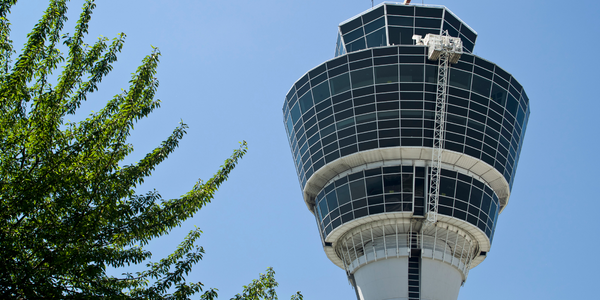
Case Study
Airport SCADA Systems Improve Service Levels
Modern airports are one of the busiest environments on Earth and rely on process automation equipment to ensure service operators achieve their KPIs. Increasingly airport SCADA systems are being used to control all aspects of the operation and associated facilities. This is because unplanned system downtime can cost dearly, both in terms of reduced revenues and the associated loss of customer satisfaction due to inevitable travel inconvenience and disruption.
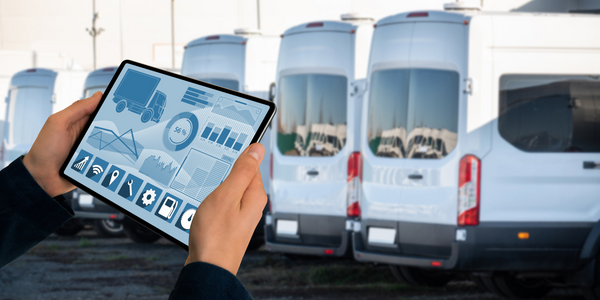
Case Study
IoT-based Fleet Intelligence Innovation
Speed to market is precious for DRVR, a rapidly growing start-up company. With a business model dependent on reliable mobile data, managers were spending their lives trying to negotiate data roaming deals with mobile network operators in different countries. And, even then, service quality was a constant concern.
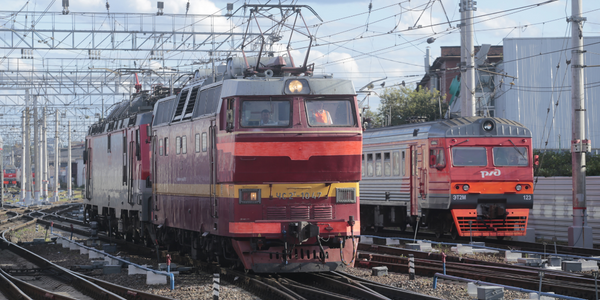
Case Study
Digitize Railway with Deutsche Bahn
To reduce maintenance costs and delay-causing failures for Deutsche Bahn. They need manual measurements by a position measurement system based on custom-made MEMS sensor clusters, which allow autonomous and continuous monitoring with wireless data transmission and long battery. They were looking for data pre-processing solution in the sensor and machine learning algorithms in the cloud so as to detect critical wear.