Download PDF
Predicting Carpark Capacity at Ascendas-Singbridge Using Machine Learning
Technology Category
- Analytics & Modeling - Predictive Analytics
- Analytics & Modeling - Machine Learning
Applicable Functions
- Facility Management
Use Cases
- Predictive Maintenance
Services
- Data Science Services
The Challenge
Ascendas-Singbridge Group (ASG), a leading sustainable urban and business space solutions provider in Asia, was facing a challenge with parking capacity at their properties. In densely populated cities like Singapore, parking capacity is a major issue. Despite having high-rise buildings with carparks or garages, parking capacity remained a challenge for both property managers and drivers. ASG wanted to forecast and predict parking lot capacity to optimize their parking services, improve the experience for visitors and drivers, and potentially increase revenue. They had previously used a different platform for model building, but it was costly and did not deliver the accurate predictions they needed.
About The Customer
Ascendas-Singbridge Group (ASG) is Asia’s leading sustainable urban and business space solutions provider, with Assets Under Management totaling more than $20 billion worldwide. Headquartered in Singapore, the Group has a presence across 11 countries in Asia, Australia, Europe, and the United States of America. They are focused on improving parking lot efficiency at their many properties around Singapore and throughout Asia. The company has more than $20 billion worth of Assets Under Management (AUM) in 28 cities across 11 countries, including Australia, China, India, Indonesia, and Singapore.
The Solution
ASG turned to DataRobot’s automated machine learning platform, specifically its Time Series features, to accurately forecast the capacity of their parking lots. They had previously used a different platform for model building, but it was costly and did not deliver the accurate predictions they needed. After a successful proof of concept with DataRobot, they found that the platform could generate more accurate predictions and was easier to use. The ultimate goal is to make carpark availability information accessible to drivers through an app. This would allow drivers to find which buildings around them have hourly parking spots available, providing a new revenue stream for ASG and a better parking experience for drivers.
Operational Impact
Quantitative Benefit
Related Case Studies.
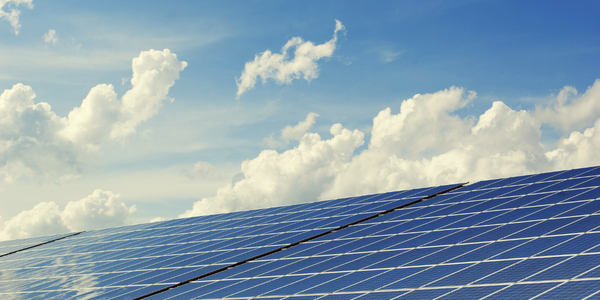
Case Study
Remote Monitoring & Predictive Maintenance App for a Solar Energy System
The maintenance & tracking of various modules was an overhead for the customer due to the huge labor costs involved. Being an advanced solar solutions provider, they wanted to ensure early detection of issues and provide the best-in-class customer experience. Hence they wanted to automate the whole process.
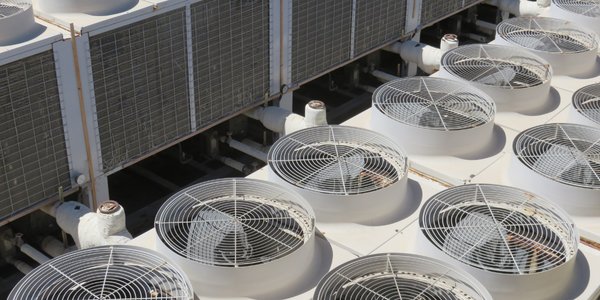
Case Study
Predictive Maintenance for Industrial Chillers
For global leaders in the industrial chiller manufacturing, reliability of the entire production process is of the utmost importance. Chillers are refrigeration systems that produce ice water to provide cooling for a process or industrial application. One of those leaders sought a way to respond to asset performance issues, even before they occur. The intelligence to guarantee maximum reliability of cooling devices is embedded (pre-alarming). A pre-alarming phase means that the cooling device still works, but symptoms may appear, telling manufacturers that a failure is likely to occur in the near future. Chillers who are not internet connected at that moment, provide little insight in this pre-alarming phase.
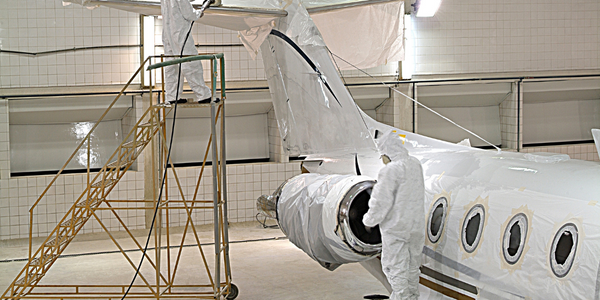
Case Study
Aircraft Predictive Maintenance and Workflow Optimization
First, aircraft manufacturer have trouble monitoring the health of aircraft systems with health prognostics and deliver predictive maintenance insights. Second, aircraft manufacturer wants a solution that can provide an in-context advisory and align job assignments to match technician experience and expertise.
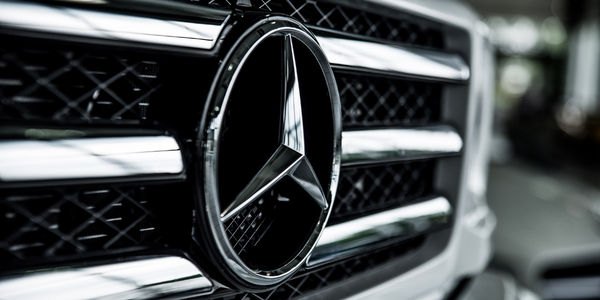
Case Study
Integral Plant Maintenance
Mercedes-Benz and his partner GAZ chose Siemens to be its maintenance partner at a new engine plant in Yaroslavl, Russia. The new plant offers a capacity to manufacture diesel engines for the Russian market, for locally produced Sprinter Classic. In addition to engines for the local market, the Yaroslavl plant will also produce spare parts. Mercedes-Benz Russia and his partner needed a service partner in order to ensure the operation of these lines in a maintenance partnership arrangement. The challenges included coordinating the entire maintenance management operation, in particular inspections, corrective and predictive maintenance activities, and the optimizing spare parts management. Siemens developed a customized maintenance solution that includes all electronic and mechanical maintenance activities (Integral Plant Maintenance).
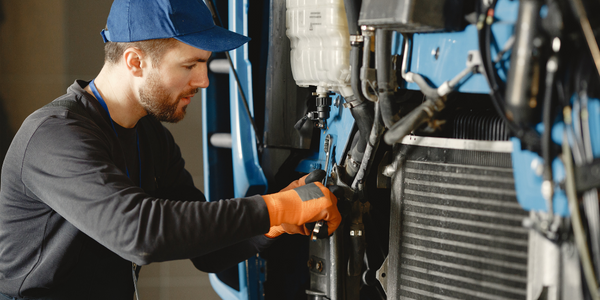
Case Study
Asset Management and Predictive Maintenance
The customer prides itself on excellent engineering and customer centric philosophy, allowing its customer’s minds to be at ease and not worry about machine failure. They can easily deliver the excellent maintenance services to their customers, but there are some processes that can be automated to deliver less downtime for the customer and more efficient maintenance schedules.