Download PDF
Sharper Shape's Efficient ML Pipeline with Labelbox and Valohai
Technology Category
- Analytics & Modeling - Machine Learning
- Platform as a Service (PaaS) - Application Development Platforms
Applicable Industries
- Education
- Utilities
Use Cases
- Computer Vision
- Time Sensitive Networking
Services
- Data Science Services
- Training
The Challenge
Sharper Shape, a company that creates technology for safe, efficient transmission and distribution solutions for utilities, was facing challenges in developing their machine learning (ML) models. The company uses computer vision models in advanced aerial sensor systems to power the automatic collection and analysis of unmanned aerial inspection data. A common use case for their technology is the identification of dangerous setups with electric wiring, such as vegetation growing too close, broken insulators, and more. However, training multiple computer vision models required a vast amount of accurately labeled images. Prior to using Labelbox, the Sharper Shape team relied on heavily manual workflows and experimented with open-source labeling tools that did not provide the required amount of configuration needed for their needs. Additionally, each data scientist had spent up to a third of their time on infrastructure and experiment management.
About The Customer
Sharper Shape is a technology company that creates safe, efficient transmission and distribution solutions for utilities. They use drones to perform utility inspections and use computer vision models in advanced aerial sensor systems to power the automatic collection and analysis of unmanned aerial inspection data. Their technology is commonly used for the identification of dangerous setups with electric wiring, such as vegetation growing too close, broken insulators, and more, so that utility companies can find and address potential hazards. As a company fueled by AI, Sharper Shape sets itself apart with a strong, established pipeline for developing their ML models.
The Solution
Sharper Shape turned to Labelbox to streamline the labeling process, enabling them to use an array of data types, including tiled imagery, and organize their existing data. With Labelbox, the team could connect their raw data into Labelbox via a simple API. Labelbox’s collaboration features also enabled rapid onboarding, training, and throughput for both internal and skilled external labelers to work together in one centralized environment. In a new initiative, the Sharper Shape team is accelerating their labeling process even more with model-assisted labeling, which allows teams to import their model into Labelbox and address edge cases. After their data is fully annotated inside of Labelbox, their data is exported to the Valohai MLOps platform, where the Sharper Shape team runs their machine learning experiments and training pipelines. Valohai enables Sharper Shape to train their models on powerful cloud hardware without DevOps support and to house all their collaborative experiments under a single application. Established ML processes can be fully automated into Valohai pipelines, so models can be trained each time new annotated data is available from Labelbox.
Operational Impact
Quantitative Benefit
Related Case Studies.

Case Study
IoT Solutions for Smart City | Internet of Things Case Study
There were several challenges faced: It is challenging to build an appliance that can withstand a wide range of voltage fluctuations from as low at 90v to as high as 320v. Since the device would be installed in remote locations, its resilience was of paramount importance. The device would have to deal with poor network coverage and have the ability to store and re-transmit data if networks were not available, which is often the case in rural India. The device could store up to 30 days of data.
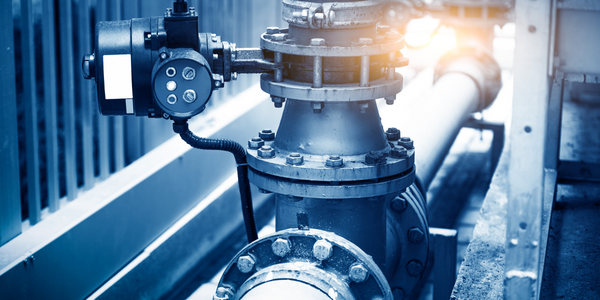
Case Study
Automation of the Oguz-Gabala-Baku water pipeline, Azerbaijan
The Oguz-Gabala-Baku water pipeline project dates back to plans from the 1970’s. Baku’s growth was historically driven by the booming oil industry and required the import of drinking water from outside of the city. Before the construction of the pipeline, some 60 percent of the city’s households received water for only a few hours daily. After completion of the project, 75 percent of the two million Baku residents are now served around the clock with potable water, based on World Health Organization (WHO) standards. The 262-kilometer pipeline requires no pumping station, but uses the altitude differences between the Caucasian mountains and the capital to supply 432,000 m³/d to the Ceyranbatan water reservoir. To the people of Baku, the pipeline is “the most important project not only in 2010, but of the last 20 years.”
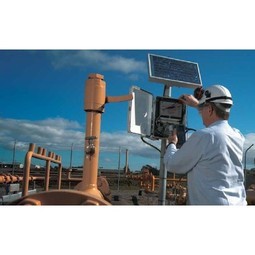
Case Study
GPRS Mobile Network for Smart Metering
Around the world, the electricity supply industry is turning to ‘smart’ meters to lower costs, reduce emissions and improve the management of customer supplies. Smart meters collect detailed consumption information and using this feedback consumers can better understand their energy usage which in turn enables them to modify their consumption to save money and help to cut carbon emissions. A smart meter can be defined in many ways, but generally includes an element of two-way communication between the household meter and the utility provider to efficiently collect detailed energy usage data. Some implementations include consumer feedback beyond the energy bill to include online web data, SMS text messages or an information display in consumers’ premises. Providing a cost-effective, reliable communications mechanism is one of the most challenging aspects of a smart meter implementation. In New Zealand, the utilities have embraced smart metering and designed cost effective ways for it to be implemented. The New Zealand government has encouraged such a move to smart metering by ensuring the energy legislation is consistent with the delivery of benefits to the consumer while allowing innovation in this area. On the ground, AMS is a leader in the deployment of smart metering and associated services. Several of New Zealand’s energy retailers were looking for smart metering services for their residential and small business customers which will eventually account for over 500,000 meters when the multi-year national deployment program is concluded. To respond to these requirements, AMS needed to put together a solution that included data communications between each meter and the central data collection point and the solution proposed by Vodafone satisfied that requirement.
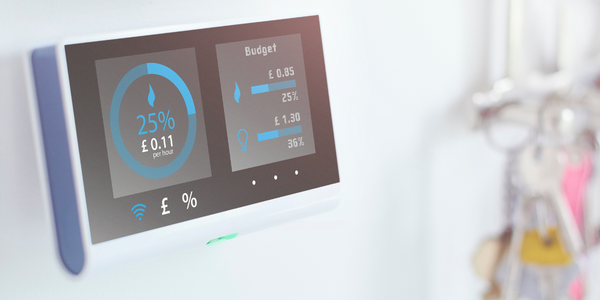
Case Study
NB-IoT connected smart meters to improve gas metering in Shenzhen
Shenzhen Gas has a large fleet of existing gas meters, which are installed in a variety of hard to reach locations, such as indoors and underground, meaning that existing communications networks have struggled to maintain connectivity with all meters. The meter success rate is low, data transmissions are so far unstable and power consumption is too high. Against this background, Shenzhen Gas, China Telecom, Huawei, and Goldcard have jointly trialed NB-IoT gas meters to try and solve some of the challenges that the industry faces with today’s smart gas meters.
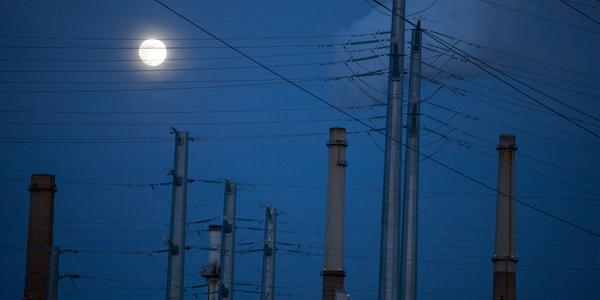
Case Study
OneWireless Enabled Performance Guarantee Test
Tata Power's power generation equipment OEMs (M/s BHEL) is required to provide all of the instrumentation and measurement devices for conducting performance guarantee and performance evaluation tests. M/s BHEL faced a number of specific challenges in conducting PG tests: employing high-accuracy digital communications for instrumentation, shortening setup and dismantling time, reducing hardware required, making portable instrument setup, avoiding temporary cabling work and the material waste costs
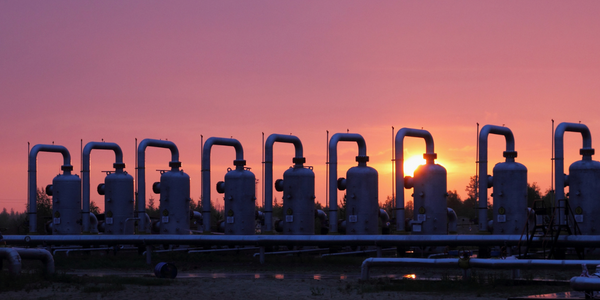
Case Study
British Gas Modernizes its Operations with Innovative Smart Metering Deployment
The UK government has mandated that smart meters are rolled out as standard across Great Britain by end of 2020, and this roll-out is estimated to create £14 billion in net benefits to the UK in consumer energy savings and lower energy generation demand, according to the Oxford Economics report, “The Value of Smart Metering to Great Britain.” While smart-metering systems have been deployed in many countries, the roll-out in Great Britain is unique because it is led by energy retailers, who have responsibility for the Electricity and Gas meters. The decision to have a retailer-led roll out was made by DECC (Department of Energy and Climate Change) to improve customer experience and drive consumer benefits. It has also led to some unique system-level requirements to support the unique local regulatory model.