下载PDF
Sharper Shape's Efficient ML Pipeline with Labelbox and Valohai
技术
- 分析与建模 - 机器学习
- 平台即服务 (PaaS) - 应用开发平台
适用行业
- 教育
- 公用事业
用例
- 计算机视觉
- 时间敏感网络
服务
- 数据科学服务
- 培训
挑战
Sharper Shape 是一家为公用事业公司提供安全、高效输电和配电解决方案技术的公司,该公司在开发机器学习 (ML) 模型时面临着挑战。该公司在先进的航空传感器系统中使用计算机视觉模型来支持无人机检查数据的自动收集和分析。他们的技术的一个常见用例是识别危险的电线设置,例如植被生长得太近、绝缘体破损等。然而,训练多个计算机视觉模型需要大量精确标记的图像。在使用 Labelbox 之前,Sharper Shape 团队严重依赖手动工作流程,并尝试使用开源标签工具,但这些工具无法提供满足其需求所需的配置量。此外,每位数据科学家花费了多达三分之一的时间在基础设施和实验管理上。
关于客户
Sharper Shape 是一家为公用事业公司创建安全、高效的输电和配电解决方案的技术公司。他们使用无人机执行公用事业检查,并在先进的航空传感器系统中使用计算机视觉模型来支持无人机检查数据的自动收集和分析。他们的技术通常用于识别危险的电线设置,例如植被生长得太近、绝缘体破损等,以便公用事业公司可以发现并解决潜在危险。作为一家以人工智能为推动力的公司,Sharper Shape 凭借强大、成熟的 ML 模型开发渠道而脱颖而出。
解决方案
Sharper Shape 采用 Labelbox 来简化标记流程,使他们能够使用一系列数据类型(包括平铺图像)并组织现有数据。借助 Labelbox,团队可以通过简单的 API 将原始数据连接到 Labelbox。 Labelbox 的协作功能还支持内部和熟练的外部贴标人员快速入职、培训和提高吞吐量,以便在一个集中式环境中协同工作。在一项新计划中,Sharper Shape 团队正在通过模型辅助标记进一步加速其标记流程,该标记允许团队将其模型导入 Labelbox 并解决边缘情况。在 Labelbox 内对数据进行完全注释后,他们的数据将导出到 Valohai MLOps 平台,Sharper Shape 团队在该平台上运行机器学习实验和训练管道。 Valohai 使 Sharper Shape 能够在强大的云硬件上训练模型,无需 DevOps 支持,并将所有协作实验容纳在单个应用程序中。已建立的 ML 流程可以完全自动化到 Valohai 管道中,因此每次 Labelbox 提供新的注释数据时都可以训练模型。
运营影响
数量效益
相关案例.

Case Study
IoT Solutions for Smart City | Internet of Things Case Study
There were several challenges faced: It is challenging to build an appliance that can withstand a wide range of voltage fluctuations from as low at 90v to as high as 320v. Since the device would be installed in remote locations, its resilience was of paramount importance. The device would have to deal with poor network coverage and have the ability to store and re-transmit data if networks were not available, which is often the case in rural India. The device could store up to 30 days of data.
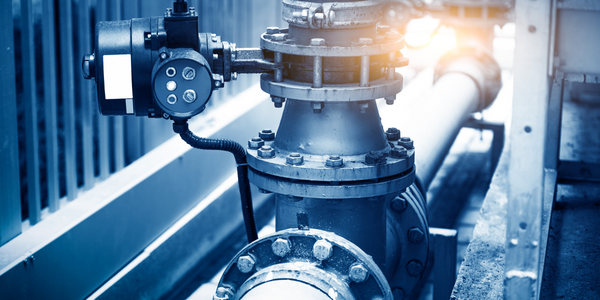
Case Study
Automation of the Oguz-Gabala-Baku water pipeline, Azerbaijan
The Oguz-Gabala-Baku water pipeline project dates back to plans from the 1970’s. Baku’s growth was historically driven by the booming oil industry and required the import of drinking water from outside of the city. Before the construction of the pipeline, some 60 percent of the city’s households received water for only a few hours daily. After completion of the project, 75 percent of the two million Baku residents are now served around the clock with potable water, based on World Health Organization (WHO) standards. The 262-kilometer pipeline requires no pumping station, but uses the altitude differences between the Caucasian mountains and the capital to supply 432,000 m³/d to the Ceyranbatan water reservoir. To the people of Baku, the pipeline is “the most important project not only in 2010, but of the last 20 years.”
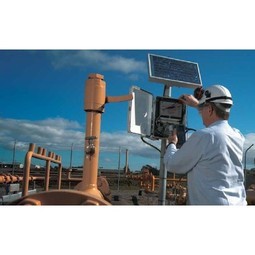
Case Study
GPRS Mobile Network for Smart Metering
Around the world, the electricity supply industry is turning to ‘smart’ meters to lower costs, reduce emissions and improve the management of customer supplies. Smart meters collect detailed consumption information and using this feedback consumers can better understand their energy usage which in turn enables them to modify their consumption to save money and help to cut carbon emissions. A smart meter can be defined in many ways, but generally includes an element of two-way communication between the household meter and the utility provider to efficiently collect detailed energy usage data. Some implementations include consumer feedback beyond the energy bill to include online web data, SMS text messages or an information display in consumers’ premises. Providing a cost-effective, reliable communications mechanism is one of the most challenging aspects of a smart meter implementation. In New Zealand, the utilities have embraced smart metering and designed cost effective ways for it to be implemented. The New Zealand government has encouraged such a move to smart metering by ensuring the energy legislation is consistent with the delivery of benefits to the consumer while allowing innovation in this area. On the ground, AMS is a leader in the deployment of smart metering and associated services. Several of New Zealand’s energy retailers were looking for smart metering services for their residential and small business customers which will eventually account for over 500,000 meters when the multi-year national deployment program is concluded. To respond to these requirements, AMS needed to put together a solution that included data communications between each meter and the central data collection point and the solution proposed by Vodafone satisfied that requirement.
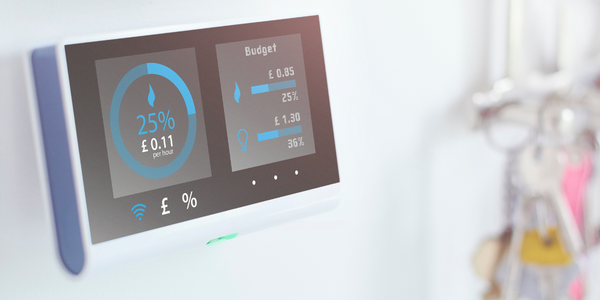
Case Study
NB-IoT connected smart meters to improve gas metering in Shenzhen
Shenzhen Gas has a large fleet of existing gas meters, which are installed in a variety of hard to reach locations, such as indoors and underground, meaning that existing communications networks have struggled to maintain connectivity with all meters. The meter success rate is low, data transmissions are so far unstable and power consumption is too high. Against this background, Shenzhen Gas, China Telecom, Huawei, and Goldcard have jointly trialed NB-IoT gas meters to try and solve some of the challenges that the industry faces with today’s smart gas meters.
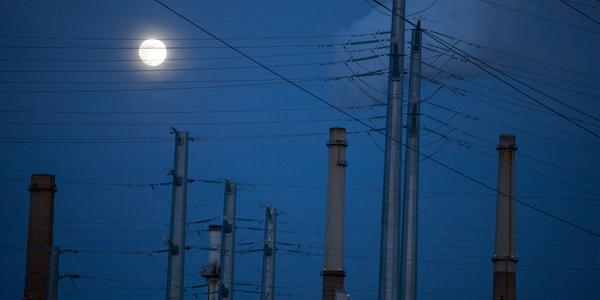
Case Study
OneWireless Enabled Performance Guarantee Test
Tata Power's power generation equipment OEMs (M/s BHEL) is required to provide all of the instrumentation and measurement devices for conducting performance guarantee and performance evaluation tests. M/s BHEL faced a number of specific challenges in conducting PG tests: employing high-accuracy digital communications for instrumentation, shortening setup and dismantling time, reducing hardware required, making portable instrument setup, avoiding temporary cabling work and the material waste costs
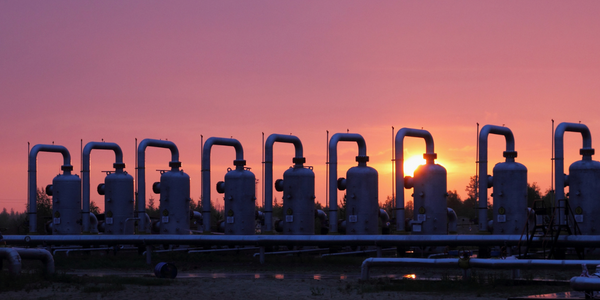
Case Study
British Gas Modernizes its Operations with Innovative Smart Metering Deployment
The UK government has mandated that smart meters are rolled out as standard across Great Britain by end of 2020, and this roll-out is estimated to create £14 billion in net benefits to the UK in consumer energy savings and lower energy generation demand, according to the Oxford Economics report, “The Value of Smart Metering to Great Britain.” While smart-metering systems have been deployed in many countries, the roll-out in Great Britain is unique because it is led by energy retailers, who have responsibility for the Electricity and Gas meters. The decision to have a retailer-led roll out was made by DECC (Department of Energy and Climate Change) to improve customer experience and drive consumer benefits. It has also led to some unique system-level requirements to support the unique local regulatory model.