Streamlining Research and Project Management in AI and ML with Neptune: A Case Study at TH Köln
- Analytics & Modeling - Machine Learning
- Application Infrastructure & Middleware - Data Exchange & Integration
- Buildings
- Construction & Infrastructure
- Product Research & Development
- Experimentation Automation
- Time Sensitive Networking
- System Integration
- Training
TH Köln, Germany’s largest University of Applied Sciences in the Electrical Engineering Department, was facing significant challenges in managing large-scale research projects. The department, which focuses on meta-learning research with standard ML frameworks such as TensorFlow and PyTorch, was struggling with experiment-tracking across multiple servers. The team was manually creating CSV files to record details generated during the experiment run such as loss or f2 score. Additionally, there were separate files with hyperparameters and other configurations. This manual management of multiple files made the analysis of past experiments extremely challenging and prone to errors. The team was also facing issues with multi-server project management, access control management, result comparison and presentation, and loss of experiment history when students left.
TH Köln is Germany’s largest University of Applied Sciences in the Electrical Engineering Department. The department focuses on meta-learning research with standard ML frameworks such as TensorFlow and PyTorch. Jan, a staff member and PhD candidate at the university, is regularly engaged in multiple projects collaborating with both students and colleagues. He is responsible for managing large-scale research projects and was seeking a solution to streamline the process of experiment-tracking across multiple servers.
Jan, a staff member and PhD candidate at TH Köln, began looking for a solution to these challenges. Initially, he considered open-source software (OSS) solutions like Sacred. However, he found that these options required a lot of administrative work, which could distract from the core ML experiments. Issues included complex installations, security concerns due to delayed updates, and resource consumption. Jan then discovered Neptune, a solution that required minimal setup time and offered zero-hassle integration. Neptune was ready in 5-10 minutes and offered a one-time integration and smart tracking and presentation capabilities. This made it easy to onboard collaborators or colleagues. Jan has been a satisfied Neptune user for two years.
Related Case Studies.
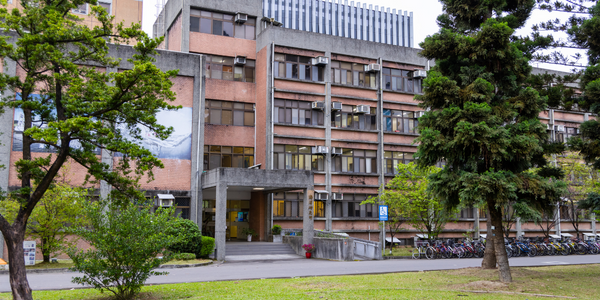
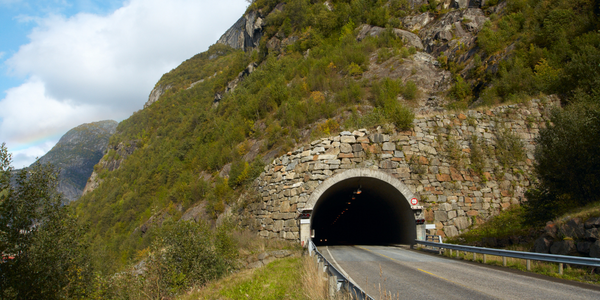
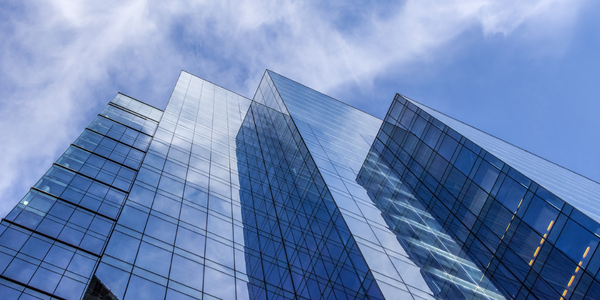
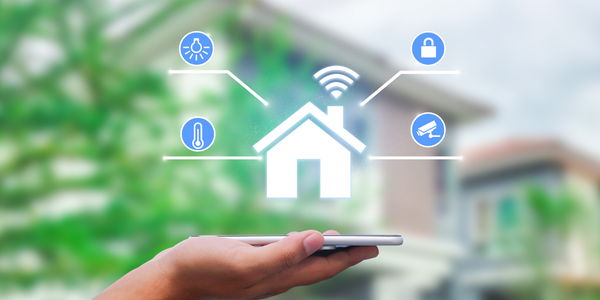
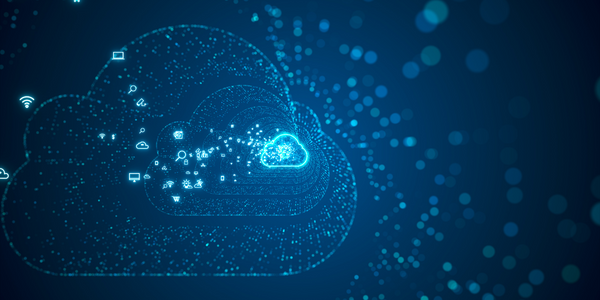