下载PDF
Deep Learning Boosts Robotic Picking Flexibility
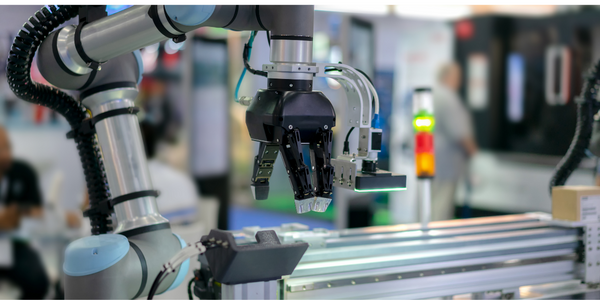
技术
- 分析与建模 - 机器学习
适用功能
- 离散制造
- 物流运输
用例
- 工厂可见化与智能化
服务
- 软件设计与工程服务
挑战
长期以来,抓取和操作各种形状和大小的物品一直是工业机器人面临的最大挑战之一。波兰尼悖论也许最好地概括了这个困难,它指出我们“知道的比我们能说的多”。从本质上讲,虽然教机器在需要抽象推理的任务(如运行计算)上表现出高水平的性能可能很容易,但要让它们具备即使是小孩的感觉运动技能,也很难。最标准化和可预测的环境。
然而,随着对灵活拣选的需求不断增加,以适应更多不同产品运行的更短的转换时间,行业正在寻求新的解决方案来解决这个问题。
解决方案
Festo 与卡尔斯鲁厄理工学院 (KIT) 之间的一个名为 FLAIRPOP(机器人拣选联合学习)的新合作项目旨在使用来自多个工作站、工厂甚至公司的训练数据来更有效地驱动深度学习算法,其目标是帮助采摘机器人变得更具适应性。该方法类似于云驱动的机器学习算法所使用的方法,后者利用的数据量比单个最终用户访问的数据量更大。
运营影响
相关案例.
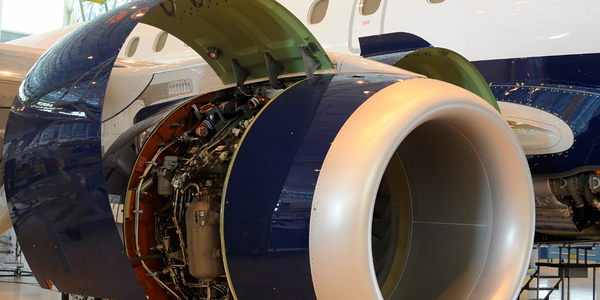
Case Study
Accelerate Production for Spirit AeroSystems
The manufacture and assembly of massive fuselage assemblies and other large structures generates a river of data. In fact, the bill of materials for a single fuselage alone can be millions of rows of data. In-house production processes and testing, as well as other manufacturers and customers created data flows that overwhelmed previous processes and information systems. Spirit’s customer base had grown substantially since their 2005 divestiture from Boeing, resulting in a $41 billion backlog of orders to fill. To address this backlog, meet increased customer demands and minimize additional capital investment, the company needed a way to improve throughput in the existing operational footprint. Spirit had a requirement from customers to increase fuselage production by 30%. To accomplish this goal, Spirit needed real-time information on its value chain and workflow. However, the two terabytes of data being pulled from their SAP ECC was unmanageable and overloaded their business warehouse. It had become time-consuming and difficult to pull aggregate data, disaggregate it for the needed information and then reassemble to create a report. During the 6-8 hours it took to build a report, another work shift (they run three per day) would have already taken place, thus the report content was out-of-date before it was ever delivered. As a result, supervisors often had to rely on manual efforts to provide charts, reports and analysis.
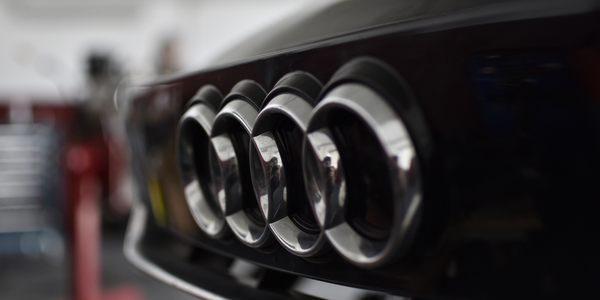
Case Study
AUDI AG 20% PRODUCTIVITY IMPROVEMENT
In the automotive industry, niche products are becoming more and more important. The automobile industry has changed considerably over the past few years. No doubt: to remain competitive, instant reaction to specific customer requests is of utmost importance. In a parallel to the growing individualization of our society, the range of models available from Audi has increased as well.
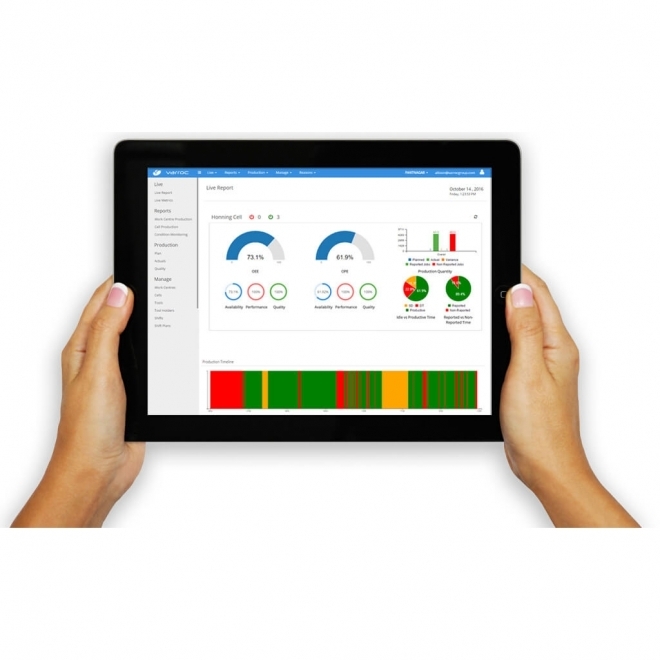
Case Study
Automotive Component Manufacturer Improves with Datonis IoT Solution
As a part of their Industry 4.0 initiative, the customer primarily wished to leverage IoT for maximizing operational efficiencies, productivity, reducing the energy footprints and maximizing capacity utilization. But there were several challenges at the outset:Firstly, the customer had multiple assembly lines with a diverse set of machines, systems and sensors, all communicating on different protocols. As such, primarily they needed a partner who could connect diverse set of assets on to a single platform and make use of underutilized ‘dark’ data.Secondly, the amount of data, data types and their applications was so vast, that the platform handling it, needed to be scalable and flexible.Lastly, Varroc faced the typical challenge of innovating in ‘Brownfield’ markets – wherein the real bottleneck is in integrating IoT in tandem with both the new and legacy equipment without any further CAPEX for asset substitution.
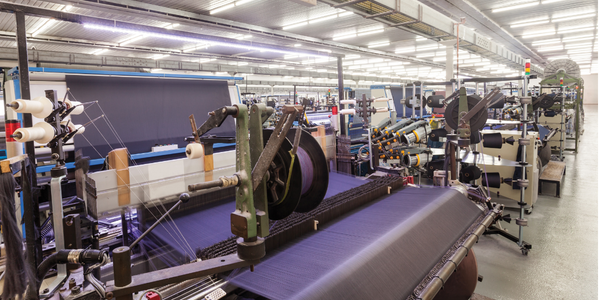
Case Study
Improving productivity and quality in Textiles
VISIBILITY - Currently unable to see amount of power consumed per machine or plant; need to account for energy used per batch. WASTE - 10% of product fails quality standards, unable to identify point of failure (initial spinning, weaving, final processing, etc). INACCURACY - Acquiring OEE measurements and compiling them is a manual process, which lacks visibility into all parts of the production process and is error-prone. LACK OF INTEROPERABILITY - Outdated machinery still has long, effective lifespans but needs to connect to newer, digital assets
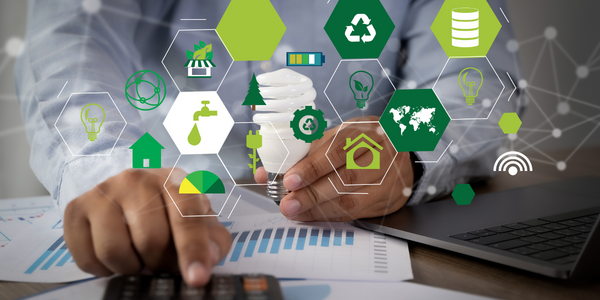
Case Study
IoE Increases Operational Efficiencies and Improves Energy Management
For many manufacturing companies today, the opportunity to connect people, process, data, and things created by the Internet of Everything (IoE) presents a new way to look at factory automation. The opportunity for digital innovation often arises when a company is expanding capacity or building a new production facility. Mahindra and Mahindra, one of India’s leading automakers, seized the opportunity to deploy a connected factory of the future at their new Chakan facility.