下载PDF
Trupanion Increases Productivity 10X with DataRobot
技术
- 分析与建模 - 机器学习
适用功能
- 销售与市场营销
- 商业运营
用例
- 质量预测分析
服务
- 数据科学服务
挑战
Trupanion 是一家领先的猫狗医疗保险提供商,该公司正在处理来自其业务不同方面的大量数据,包括定价、销售、索赔预测、客户保留等。他们在报告指标方面做得很好,但他们还没有技术能力对这些数据进行更深入的分析,以便做出最佳决策。这需要更复杂的技术和大量时间。Trupanion 正在寻找快速准确的预测建模软件,该软件足够强大,可以支持来自其业务不同功能的所有不同数据和信息。
关于客户
Trupanion 是美国和加拿大领先的猫狗医疗保险提供商。近二十年来,Trupanion 让宠物主人安心无忧,他们可以专注于宠物的康复,而不必担心财务压力。Trupanion 致力于为宠物主人提供最高价值的宠物医疗保险。Trupanion 在纳斯达克上市,股票代码为 TRUP。该公司于 2000 年在加拿大不列颠哥伦比亚省温哥华成立,总部位于华盛顿州西雅图。Trupanion 保单在美国由其全资保险实体 American Pet Insurance Company 签发,在加拿大由 Omega General Insurance Company 签发。
解决方案
由于 Trupanion 刚进入机器学习领域,因此由 Amazon Web Services (AWS) 提供支持的 DataRobot 平台是完美的解决方案,因为它直观且易于使用。使用 DataRobot 几周后,Trupanion 惊喜地发现这个平台具有一系列优势,而且提供的服务水平远远超出了预期。DataRobot 将构建模型所需的时间缩短了 10 倍以上,节省了大量模型测试和培训资源时间,现在这些时间可以用于解决其他问题。借助使用 DataRobot 构建的流失模型,Trupanion 能够更好地量化不同变量对客户流失的影响。
运营影响
数量效益
相关案例.
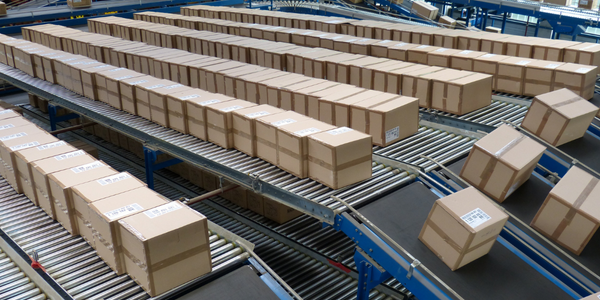
Case Study
IoT Data Analytics Case Study - Packaging Films Manufacturer
The company manufactures packaging films on made to order or configure to order basis. Every order has a different set of requirements from the product characteristics perspective and hence requires machine’s settings to be adjusted accordingly. If the film quality does not meet the required standards, the degraded quality impacts customer delivery causes customer dissatisfaction and results in lower margins. The biggest challenge was to identify the real root cause and devise a remedy for that.
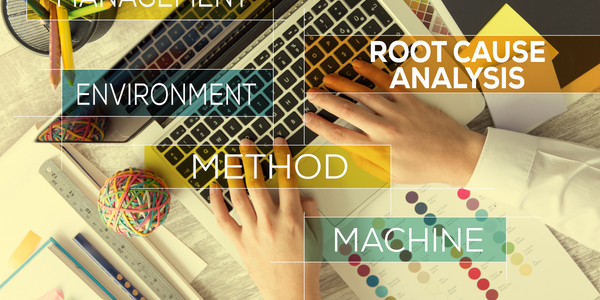
Case Study
Prevent Process Inefficiencies with Automated Root Cause Analysis
Manufacturers mostly rely on on-site expert knowledge for root cause analysis. When the defective product is sent to lab for analysis, it is laborious and always a post-mortem one. Manufacturers that collect data from IT and OT also need a comprehensive understanding of a variety of professionals to make sense of it. This is not only time consuming, but also inefficiencient.
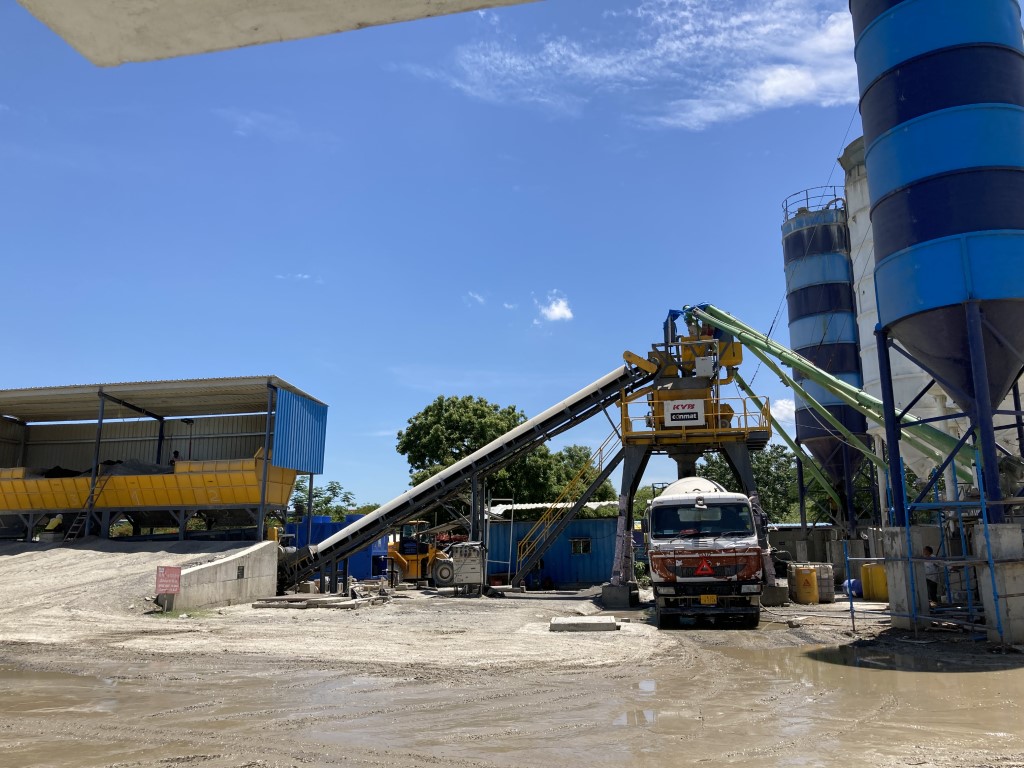
Case Study
Digitalising QC records
Ready-mix concrete batching plant with seasonal demand 6,000 to 12,000 cu.metre per month.Batch-cycle records for each truck is stored in paper format. 1000 to 2000 truck loads per month, generating ~2000 to 6000 paper records.QC anomaly detection in chemical batch-mixing is manual & time consuming.
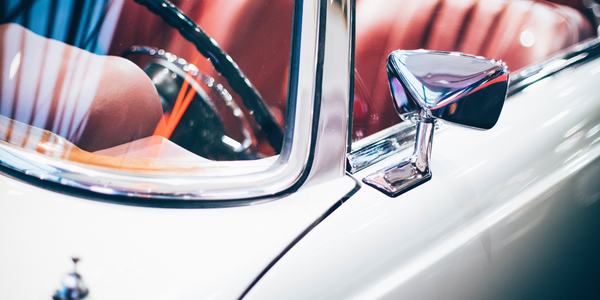
Case Study
Automotive manufacturer increases productivity for cylinder-head production by 2
Daimler AG was looking for a way to maximize the number of flawlessly produced cylinder-heads at its Stuttgart factory by making targeted process adjustments. The company also wanted to increase productivity and shorten the ramp-up phase of its complex manufacturing process.
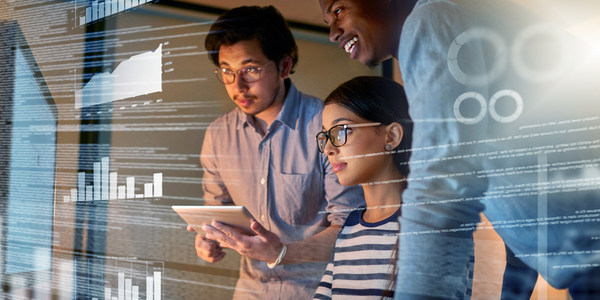
Case Study
CleanTelligent Enhances Janitorial Software Solutions with Infor Birst
CleanTelligent Software, a company that aids in-house and contracted janitorial teams in streamlining communication and improving quality control, faced a significant challenge. Their clients were demanding a more dynamic way to present reporting data. The company's software was primarily used to analyze and summarize a custodial team's performance, replacing a highly manual, paper-driven process. However, the initial differences between service providers in the janitorial industry are often unclear, and the cost of switching is comparatively low. This situation led to high client turnover, with a janitorial company's customer lifetime averaging four years or less. CleanTelligent needed to improve the customer experience with dynamic dashboards and reporting, retain customers through predictive analysis, capitalize on advanced analytics capabilities to build market differentiation, and improve client retention rates.
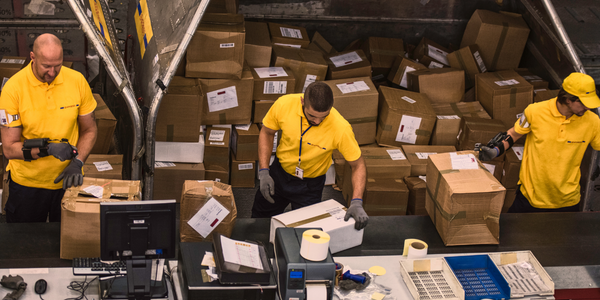
Case Study
Digitization of Pharmaceutical Packaging Machines: A Case Study of CVC Technologies
CVC Technologies, a leading manufacturer of pharmaceutical packaging machines, was seeking an end-to-end IoT solution to fully digitize their pharmaceutical liquid filling and capping machines. The company aimed to enhance the safety of their equipment, introduce digital maintenance capabilities, and gain visibility into machine status from anywhere at any time. The challenge was to find a solution that could provide real-time visibility into the machine's status, deliver direct cloud connectivity and digital services, and simplify all aspects of the machine's lifecycle, from engineering to maintenance.