下载PDF
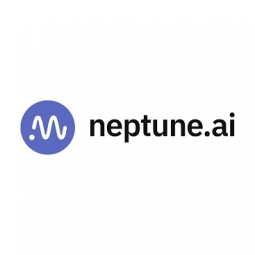
Neptune.ai
概述
公司介绍
Neptune 是一款实验追踪器,适用于那些在调试和重现实验、共享结果以及混乱的模型交接方面遇到困难的机器学习团队。
它提供了一个单一的地方来跟踪、比较、存储和协作实验,以便数据科学家可以更快地开发可用于生产的模型,而机器学习工程师可以立即访问模型工件以便将它们部署到生产中。
物联网应用简介
Neptune.ai 是分析与建模, 基础设施即服务 (iaas), 应用基础设施与中间件, 机器人, 平台即服务 (paas), 和 传感器等工业物联网科技方面的供应商。同时致力于建筑物, 水泥, 建筑与基础设施, 消费品, 教育, 设备与机械, 石油和天然气, 和 零售等行业。
技术
用例
功能区
行业
服务
技术栈
Neptune.ai的技术栈描绘了Neptune.ai在分析与建模, 基础设施即服务 (iaas), 应用基础设施与中间件, 机器人, 平台即服务 (paas), 和 传感器等物联网技术方面的实践。
-
设备层
-
边缘层
-
云层
-
应用层
-
配套技术
技术能力:
无
弱
中等
强
实例探究.
Case Study
Brainly's Integration with Amazon SageMaker and Neptune for Enhanced Machine Learning Capabilities
Brainly, a leading global learning platform, faced a challenge with their machine learning-powered feature, Snap to Solve. The feature allows users to upload a photo of a problem, which the system then detects and provides solutions for. The Visual Search team, responsible for the Visual Content Extraction (VICE) system of Snap to Solve, used Amazon SageMaker to run their computing workloads and serve their models. However, as the number of training runs on their large compute architectures increased, they found that their logs from Amazon SageMaker needed to be trackable and manageable to avoid workflow bottlenecks. They needed a tool that could scale regardless of the experiment volume. While they tried using SageMaker Experiments for tracking, they found the tracking UX and Python client unsatisfactory.
Case Study
Theta Tech AI: Enhancing Healthcare AI Systems with Neptune
Theta Tech AI, a company that builds customized artificial intelligence algorithms and front-end user interfaces for large-scale healthcare AI systems, faced several challenges in developing generalizable medical AI systems. The team had to manage thousands of experiments for large-scale parallel training workflows, which were run on GPU servers in AWS. However, they found that AWS CloudWatch Logs, their initial choice for monitoring the jobs, was inadequate for managing experiment logs. The team was unable to get experiment-relevant metrics from AWS CloudWatch Logs, debug problems with training jobs and experiments, integrate Optuna for hyperparameter optimization, and communicate the results of ML models to clients effectively.
Case Study
Optimizing Infrastructure Design with Continuum Industries' Optioneer Engine and Neptune
Continuum Industries, a company in the infrastructure industry, developed a product called Optioneer to automate and optimize the design of linear infrastructure assets. However, operating the Optioneer engine presented several challenges. The engine needed to be robust enough to handle different problems fed by different customers. Moreover, the company needed to ensure that the solutions provided by the engine were accurate and agreed upon by civil engineers. The team also had to constantly improve the optimization engine without breaking the algorithm. The nature of the problem they were trying to solve presented additional challenges. They could not automatically tell whether an algorithm output was correct or not. They needed a set of example problems that was representative of the kind of problem that the algorithm would be asked to solve in production. The team initially developed a custom solution to these problems, but it proved to be extremely clunky and complex to maintain.