Download PDF
AppAdvice: Personalized iOS app news and reviews powered by IBM Cloudant DBaaS
Technology Category
- Application Infrastructure & Middleware - Database Management & Storage
- Infrastructure as a Service (IaaS) - Cloud Databases
Applicable Industries
- Retail
Applicable Functions
- Maintenance
Use Cases
- Retail Store Automation
- Time Sensitive Networking
The Challenge
AppAdvice, a Los Angeles-based company providing a comprehensive range of iPhone and iPad application reviews, news, and app discovery services, faced a significant challenge in managing its variably structured data. The company needed to store this data in its application catalog in a way that was not only easy and inexpensive to start with but also capable of handling potentially massive future growth. Given the nature of the data, a relational SQL database was not a viable option for the platform. As an early-stage company, AppAdvice was looking for a NoSQL database that was easy and inexpensive to get started with, but built for growth.
About The Customer
AppAdvice is a Los Angeles-based company that provides a comprehensive range of iPhone and iPad application reviews, news, and app discovery services. The company helps online and mobile visitors discover interesting and new iOS apps. AppAdvice filters through the 1 million+ apps in the App Store to help novice and experienced smart device owners find relevant new apps and reviews by personalizing content based on hobbies, industry verticals, and other personalized themes. As an early-stage company, AppAdvice was looking for a NoSQL database that was easy and inexpensive to get started with, but built for growth.
The Solution
AppAdvice chose IBM Cloudant, a NoSQL database-as-a-service (DBaaS) solution, to manage its multi-structured data. The company opted for Cloudant for several reasons, including its schemaless JSON data storage, which made it a natural fit for the multi-structured apps catalog, reviews, and news that AppAdvice manages. Cloudant's scale-out architecture was another key feature, making it ideal to support AppAdvice’s user base and database growth. Its fault-tolerance, with data distributed across several data centers for high availability, was also a significant factor. Cloudant is hosted and managed by IBM Cloud Data Services, enabling AppAdvice to remain focused on development rather than being distracted by database administration. AppAdvice started out running on a multi-tenant Cloudant database cluster for free in 2010, and began paying as its application grew. The company eventually moved to a dedicated, single-tenant cluster for even better price-performance.
Operational Impact
Quantitative Benefit
Related Case Studies.
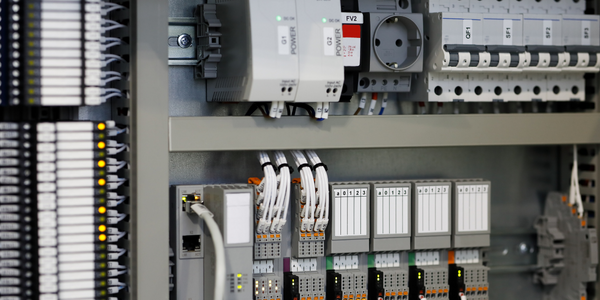
Case Study
Improving Production Line Efficiency with Ethernet Micro RTU Controller
Moxa was asked to provide a connectivity solution for one of the world's leading cosmetics companies. This multinational corporation, with retail presence in 130 countries, 23 global braches, and over 66,000 employees, sought to improve the efficiency of their production process by migrating from manual monitoring to an automatic productivity monitoring system. The production line was being monitored by ABB Real-TPI, a factory information system that offers data collection and analysis to improve plant efficiency. Due to software limitations, the customer needed an OPC server and a corresponding I/O solution to collect data from additional sensor devices for the Real-TPI system. The goal is to enable the factory information system to more thoroughly collect data from every corner of the production line. This will improve its ability to measure Overall Equipment Effectiveness (OEE) and translate into increased production efficiencies. System Requirements • Instant status updates while still consuming minimal bandwidth to relieve strain on limited factory networks • Interoperable with ABB Real-TPI • Small form factor appropriate for deployment where space is scarce • Remote software management and configuration to simplify operations
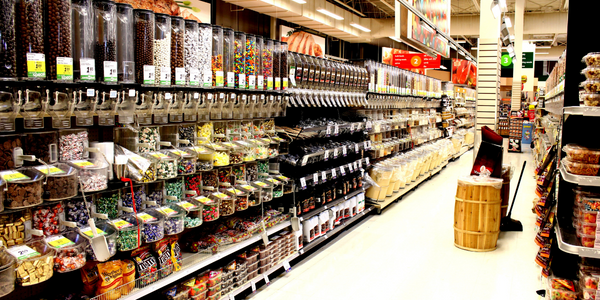
Case Study
How Sirqul’s IoT Platform is Crafting Carrefour’s New In-Store Experiences
Carrefour Taiwan’s goal is to be completely digital by end of 2018. Out-dated manual methods for analysis and assumptions limited Carrefour’s ability to change the customer experience and were void of real-time decision-making capabilities. Rather than relying solely on sales data, assumptions, and disparate systems, Carrefour Taiwan’s CEO led an initiative to find a connected IoT solution that could give the team the ability to make real-time changes and more informed decisions. Prior to implementing, Carrefour struggled to address their conversion rates and did not have the proper insights into the customer decision-making process nor how to make an immediate impact without losing customer confidence.
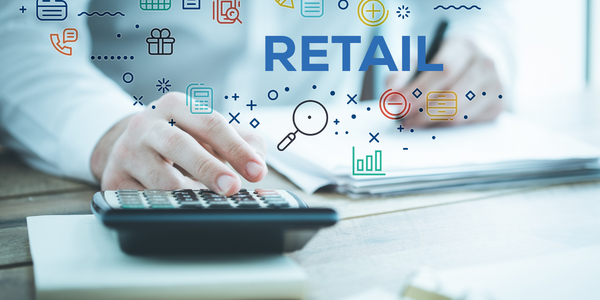
Case Study
Digital Retail Security Solutions
Sennco wanted to help its retail customers increase sales and profits by developing an innovative alarm system as opposed to conventional connected alarms that are permanently tethered to display products. These traditional security systems were cumbersome and intrusive to the customer shopping experience. Additionally, they provided no useful data or analytics.
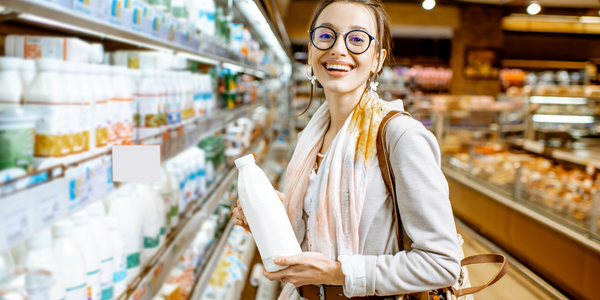
Case Study
Ensures Cold Milk in Your Supermarket
As of 2014, AK-Centralen has over 1,500 Danish supermarkets equipped, and utilizes 16 operators, and is open 24 hours a day, 365 days a year. AK-Centralen needed the ability to monitor the cooling alarms from around the country, 24 hours a day, 365 days a year. Each and every time the door to a milk cooler or a freezer does not close properly, an alarm goes off on a computer screen in a control building in southwestern Odense. This type of alarm will go off approximately 140,000 times per year, equating to roughly 400 alarms in a 24-hour period. Should an alarm go off, then there is only a limited amount of time to act before dairy products or frozen pizza must be disposed of, and this type of waste can quickly start to cost a supermarket a great deal of money.
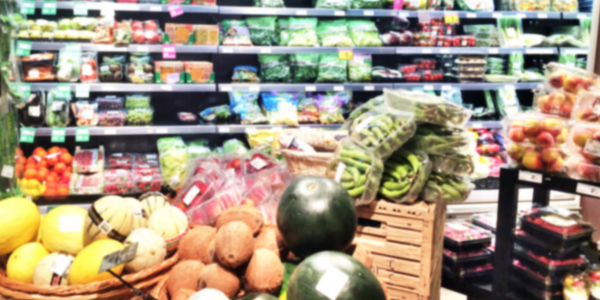
Case Study
Supermarket Energy Savings
The client had previously deployed a one-meter-per-store monitoring program. Given the manner in which energy consumption changes with external temperature, hour of the day, day of week and month of year, a single meter solution lacked the ability to detect the difference between a true problem and a changing store environment. Most importantly, a single meter solution could never identify root cause of energy consumption changes. This approach never reduced the number of truck-rolls or man-hours required to find and resolve issues.