Automating Data Processing for Enhanced Scalability: A Case Study on LeadGenius
- Application Infrastructure & Middleware - Database Management & Storage
- Infrastructure as a Service (IaaS) - Cloud Storage Services
- Oil & Gas
- Transportation
- Quality Assurance
- Sales & Marketing
- Demand Planning & Forecasting
- Visual Quality Detection
- Testing & Certification
LeadGenius, a marketing automation and demand generation company, was facing significant challenges with its data processing pipeline. The pipeline was inefficient due to a high amount of manual processes, including data incorporation and verification. This inefficiency led to bottlenecks, slowing down data delivery to customers. The data, parsed from various sources, had to be verified carefully, which when done manually, further slowed down the process. The pipeline was also lacking in terms of data quality and data consistency due to the variety of data sources and the reliance on manual processing. The company needed a solution that was not only automated but also fault-tolerant and scalable, capable of running on-demand in case of any issues with its components.
LeadGenius is a marketing automation and demand generation company that uses AI and human computation to help clients identify and communicate with targeted leads. The company needed to enhance and automate its data processing pipeline to improve its sales and marketing performance. The company's data processing pipeline was inefficient due to the high amount of manual processes and did not ensure data quality and consistency. The company approached Provectus to automate its data processing pipeline processes and optimize the platform for further scaling.
Provectus designed and built an automated, scalable, and fault-tolerant data processing and data storage solution for LeadGenius. The solution utilized cutting-edge algorithms to clean and enrich parsed data. The data parsing and processing pipeline was based on Apache Spark managed by Amazon EMR, while the data storage solution was built with Amazon S3, Amazon RDS with PostgreSQL, Amazon Redshift, and Amazon Elasticsearch service. The use of Apache Spark with Amazon EMR accelerated the collection and processing of large amounts of data from varying sources. Amazon S3 was used to optimize object data storage in the cloud, ensuring the solution’s reliability and compatibility with other AWS services to accelerate and simplify scaling. Amazon RDS and Amazon Redshift services were used for data storage, offering scalability, fault tolerance, and low latency. Amazon Elasticsearch was used to ensure timely, uninhibited customer access to the data.
Related Case Studies.
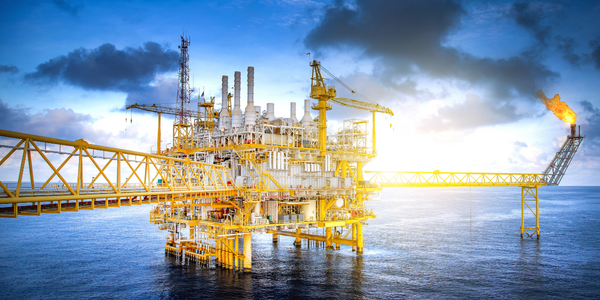
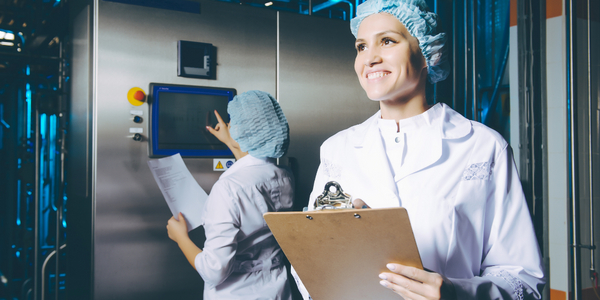
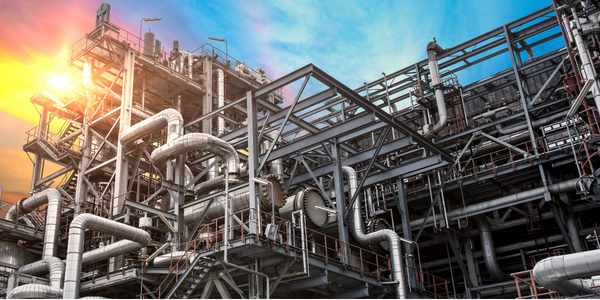
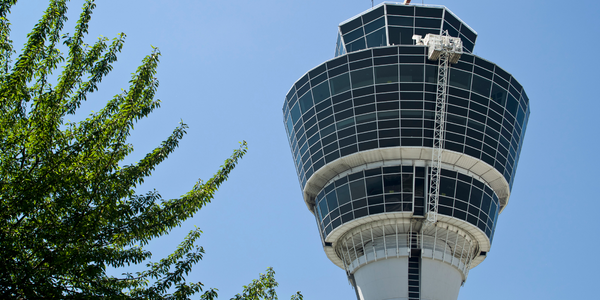