Download PDF
Boosting Oil Production by Optimizing ESP Operating Parameters with AI
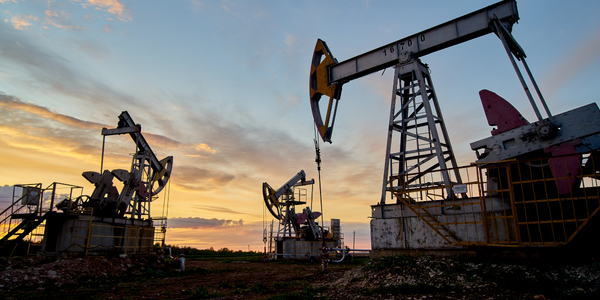
Technology Category
- Analytics & Modeling - Machine Learning
- Sensors - Utility Meters
Applicable Industries
- Oil & Gas
Applicable Functions
- Maintenance
Use Cases
- Asset Health Management (AHM)
- Leakage & Flood Monitoring
Services
- Data Science Services
- Testing & Certification
The Challenge
Vital Energy, a leading energy company, was seeking ways to increase productivity, lower production costs, avoid downtime, and prevent asset failures. The company's Electrical Submersible Pumps (ESP) played a critical role in their operations, but the manual methods for ESP monitoring and optimization were time-consuming, expensive, and prone to human error. Vital Energy wanted to create an AI-based solution for ESP optimization that would automatically recommend ESP operating parameters, provide visualization of all necessary data, and allow for feedback, input limitations, and operating constraints.
The Customer
Laredo Petroleum
About The Customer
Vital Energy is a leading energy company that focuses on the acquisition, exploration, and development of oil and natural gas properties. Operational efficiency and production optimization are among the company’s key priorities. Currently, Vital Energy is investing in digital technologies to ensure asset integrity, reduce operating costs, and increase production rates. ESP-operated wells play a strategic role in Vital Energy's digital transformation strategy. The company is constantly monitoring and tuning the operating parameters of these wells to achieve high efficiency and production targets.
The Solution
SoftServe partnered with Vital Energy to build a comprehensive solution on Amazon Web Services (AWS) using Deep Neural Network (DNN) algorithms and optimization techniques. This solution models the entire system by simulating telemetry and production with different scenarios. It allows Vital Energy to find the best possible controls to meet constraints such as motor temperature, voltage, intake pressure, and gas or water volume. The algorithm can be adapted to various ESP systems and provides operators with optimal daily control. The solution includes an ML model with high prediction accuracy of oil/gas/water production, an automatically retrained pipeline deployed to the AWS environment, interactive visualizations of simulation scenarios, a Streamlit dashboard for parameter recommendations testing, and a combination of ML- and physical-based models.
Operational Impact
Quantitative Benefit
Related Case Studies.
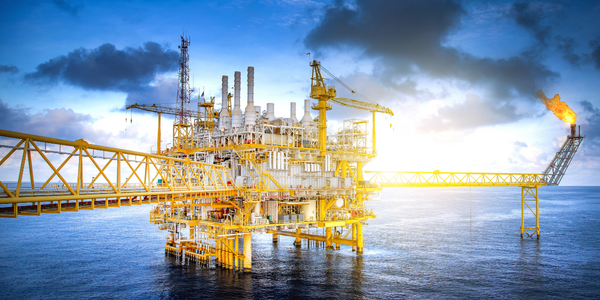
Case Study
Taking Oil and Gas Exploration to the Next Level
DownUnder GeoSolutions (DUG) wanted to increase computing performance by 5 to 10 times to improve seismic processing. The solution must build on current architecture software investments without sacrificing existing software and scale computing without scaling IT infrastructure costs.
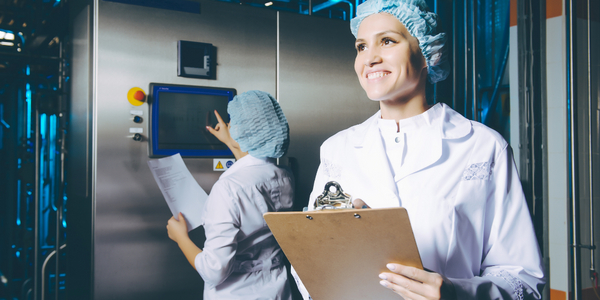
Case Study
Remote Wellhead Monitoring
Each wellhead was equipped with various sensors and meters that needed to be monitored and controlled from a central HMI, often miles away from the assets in the field. Redundant solar and wind generators were installed at each wellhead to support the electrical needs of the pumpstations, temperature meters, cameras, and cellular modules. In addition to asset management and remote control capabilities, data logging for remote surveillance and alarm notifications was a key demand from the customer. Terra Ferma’s solution needed to be power efficient, reliable, and capable of supporting high-bandwidth data-feeds. They needed a multi-link cellular connection to a central server that sustained reliable and redundant monitoring and control of flow meters, temperature sensors, power supply, and event-logging; including video and image files. This open-standard network needed to interface with the existing SCADA and proprietary network management software.
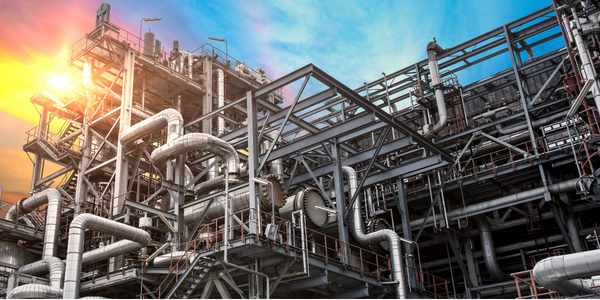
Case Study
Refinery Saves Over $700,000 with Smart Wireless
One of the largest petroleum refineries in the world is equipped to refine various types of crude oil and manufacture various grades of fuel from motor gasoline to Aviation Turbine Fuel. Due to wear and tear, eight hydrogen valves in each refinery were leaking, and each cost $1800 per ton of hydrogen vented. The plant also had leakage on nearly 30 flare control hydrocarbon valves. The refinery wanted a continuous, online monitoring system that could catch leaks early, minimize hydrogen and hydrocarbon production losses, and improve safety for maintenance.