Download PDF
Revolutionizing Dynamic Pricing with Pricemoov and Dataiku
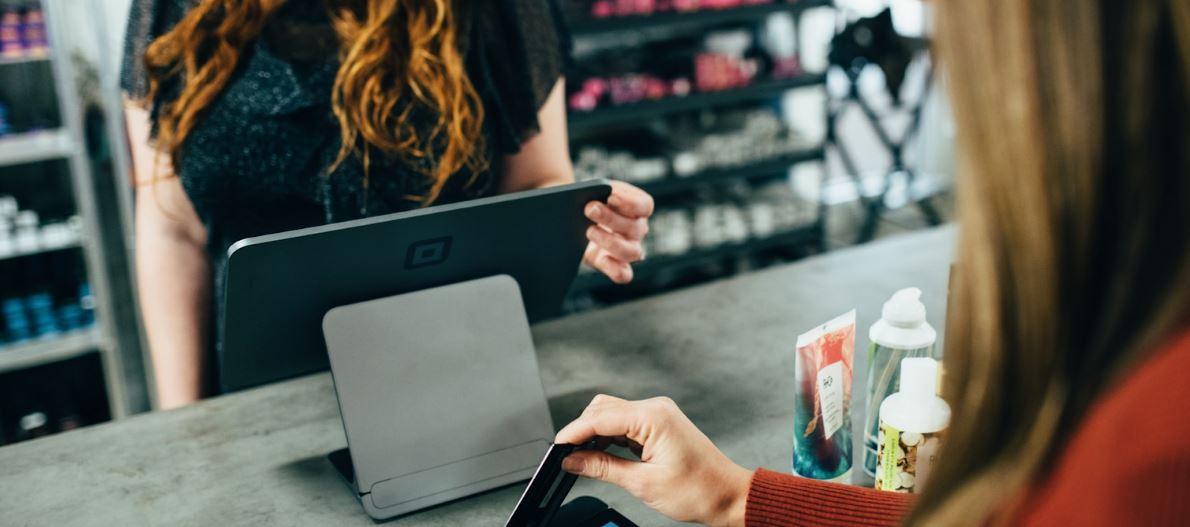
Technology Category
- Analytics & Modeling - Predictive Analytics
- Automation & Control - Human Machine Interface (HMI)
Applicable Industries
- E-Commerce
- Retail
Use Cases
- Personnel Tracking & Monitoring
- Time Sensitive Networking
The Challenge
Pricemoov, a yield management solution provider, faced a significant challenge in handling and cleaning data from old SI systems, Oracle, or MySql. The data was dirty and required a full-time developer to perform long ETL (extract-transform-load) steps in PHP for cleaning. Once cleaned, the datasets were painstakingly entered into a model, as they were custom-built pipelines. The replication and deployment process for the next customer was taking weeks. This slow and inefficient process was hindering Pricemoov's ability to provide optimal pricing suggestions and solutions to its customers in a timely manner.
The Customer
PriceMoov
About The Customer
Pricemoov is a Plug and Play Yield Management solution provider founded in 2016. It has been experiencing strong growth and its users include car rental services, airline companies, and event organizers. Pricemoov provides a service that delivers optimal pricing suggestions and solutions to its customers by weighing the intrinsic value of the item, its seasonality, and the attributes of the customer himself through detailed segmentation. To do so, Pricemoov collects datasets from its customers that are updated daily through partitioning.
The Solution
Pricemoov adopted Dataiku, a tool that transformed their business by significantly speeding up data cleaning processes and enabling quick replication of existing work. This allowed Pricemoov to run proof-of-concepts for potential customers on short notice and provide better pricing options overall. The Data Department at Pricemoov used Dataiku to replicate existing workflows, speed up data cleaning and exporting, and enable less experienced staff to assist with this process. This left tenured data scientists to focus on modeling rather than data prep and plumbing. Non-technical teams could build their skills and scale their efforts thanks to an intuitive, visual point-and-click interface. Dataiku also helped Pricemoov to better define a specific price per customer that evolves over time by melding data indicating demand with customers’ willingness to pay.
Operational Impact
Quantitative Benefit
Related Case Studies.
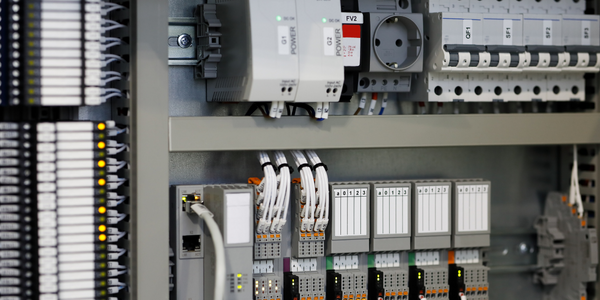
Case Study
Improving Production Line Efficiency with Ethernet Micro RTU Controller
Moxa was asked to provide a connectivity solution for one of the world's leading cosmetics companies. This multinational corporation, with retail presence in 130 countries, 23 global braches, and over 66,000 employees, sought to improve the efficiency of their production process by migrating from manual monitoring to an automatic productivity monitoring system. The production line was being monitored by ABB Real-TPI, a factory information system that offers data collection and analysis to improve plant efficiency. Due to software limitations, the customer needed an OPC server and a corresponding I/O solution to collect data from additional sensor devices for the Real-TPI system. The goal is to enable the factory information system to more thoroughly collect data from every corner of the production line. This will improve its ability to measure Overall Equipment Effectiveness (OEE) and translate into increased production efficiencies. System Requirements • Instant status updates while still consuming minimal bandwidth to relieve strain on limited factory networks • Interoperable with ABB Real-TPI • Small form factor appropriate for deployment where space is scarce • Remote software management and configuration to simplify operations
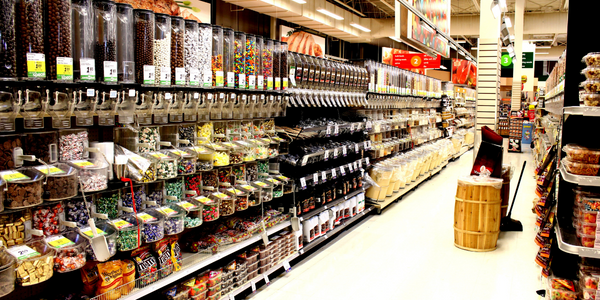
Case Study
How Sirqul’s IoT Platform is Crafting Carrefour’s New In-Store Experiences
Carrefour Taiwan’s goal is to be completely digital by end of 2018. Out-dated manual methods for analysis and assumptions limited Carrefour’s ability to change the customer experience and were void of real-time decision-making capabilities. Rather than relying solely on sales data, assumptions, and disparate systems, Carrefour Taiwan’s CEO led an initiative to find a connected IoT solution that could give the team the ability to make real-time changes and more informed decisions. Prior to implementing, Carrefour struggled to address their conversion rates and did not have the proper insights into the customer decision-making process nor how to make an immediate impact without losing customer confidence.
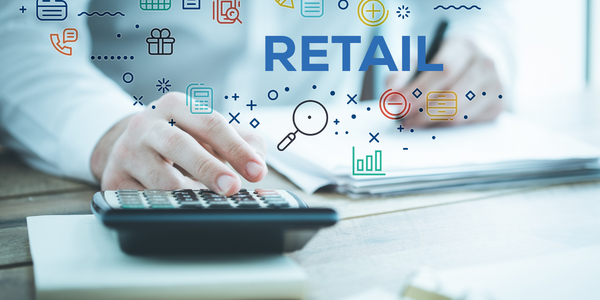
Case Study
Digital Retail Security Solutions
Sennco wanted to help its retail customers increase sales and profits by developing an innovative alarm system as opposed to conventional connected alarms that are permanently tethered to display products. These traditional security systems were cumbersome and intrusive to the customer shopping experience. Additionally, they provided no useful data or analytics.
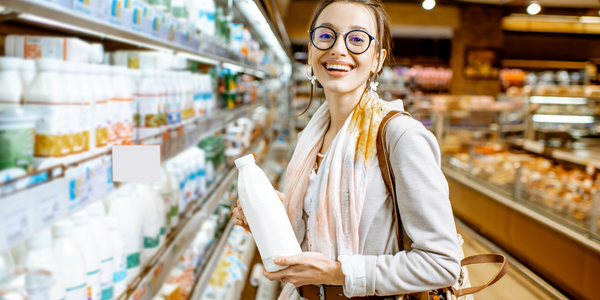
Case Study
Ensures Cold Milk in Your Supermarket
As of 2014, AK-Centralen has over 1,500 Danish supermarkets equipped, and utilizes 16 operators, and is open 24 hours a day, 365 days a year. AK-Centralen needed the ability to monitor the cooling alarms from around the country, 24 hours a day, 365 days a year. Each and every time the door to a milk cooler or a freezer does not close properly, an alarm goes off on a computer screen in a control building in southwestern Odense. This type of alarm will go off approximately 140,000 times per year, equating to roughly 400 alarms in a 24-hour period. Should an alarm go off, then there is only a limited amount of time to act before dairy products or frozen pizza must be disposed of, and this type of waste can quickly start to cost a supermarket a great deal of money.
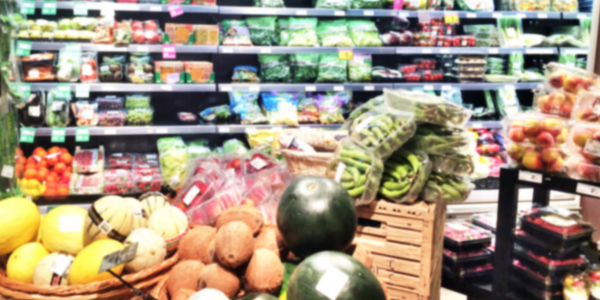
Case Study
Supermarket Energy Savings
The client had previously deployed a one-meter-per-store monitoring program. Given the manner in which energy consumption changes with external temperature, hour of the day, day of week and month of year, a single meter solution lacked the ability to detect the difference between a true problem and a changing store environment. Most importantly, a single meter solution could never identify root cause of energy consumption changes. This approach never reduced the number of truck-rolls or man-hours required to find and resolve issues.