Download PDF
Smart Pricing in Retail
Technology Category
- Analytics & Modeling - Predictive Analytics
Applicable Industries
- Retail
Applicable Functions
- Sales & Marketing
Services
- Data Science Services
The Challenge
A leading retailer in Europe with more than 3,500 stores and an e-commerce component was losing money due to being undercut by competitors on price. They also found that their customer base tended to wait until the end of seasons for huge markdowns and would only then buy certain seasonal products, which skewed their predictions for how to stock items in the future and perpetuated the pricing issue. In addition, they struggled to efficiently change prices and keep them consistent across stores and online - often, this resulted in inconsistent pricing, especially when individual store managers made their own decisions on sales. The retailer wanted to improve their pricing strategy by understanding what drove customer purchasing decisions for specific products and what prices would resonate best, easily understanding the price offered by all competitors in real time, and updating pricing consistently across stores and online.
About The Customer
The customer is a leading retailer in Europe with more than 3,500 stores as well as an e-commerce component that offers home delivery services. With hundreds of thousands of employees and customers spanning multiple countries, this retailer stays on the cutting-edge of big data technologies to remain competitive in a growing market.
The Solution
The retailer introduced Dataiku Data Science Studio (DSS) into their data team’s processes to incorporate predictive analytics at scale. They worked with Dataiku to produce a final data project that considers competitors’ pricing and uses it in a predictive model to determine, for specific products, whether the overall business can support aggressive price-based competition for that product. The solution leverages Dataiku’s REST API to adjust pricing in production automatically based on a specific set of predefined features. It uses real-time monitoring of models in production to ensure pricing model performance isn’t drifting and that pricing changes in production over time are well documented. The solution also includes a robust pricing dashboard based off of the predictive pricing model that alerts and allows physical stores to react to recommended pricing changes or online pricing changes.
Operational Impact
Quantitative Benefit
Related Case Studies.
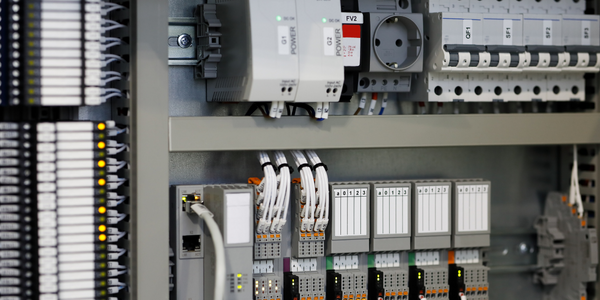
Case Study
Improving Production Line Efficiency with Ethernet Micro RTU Controller
Moxa was asked to provide a connectivity solution for one of the world's leading cosmetics companies. This multinational corporation, with retail presence in 130 countries, 23 global braches, and over 66,000 employees, sought to improve the efficiency of their production process by migrating from manual monitoring to an automatic productivity monitoring system. The production line was being monitored by ABB Real-TPI, a factory information system that offers data collection and analysis to improve plant efficiency. Due to software limitations, the customer needed an OPC server and a corresponding I/O solution to collect data from additional sensor devices for the Real-TPI system. The goal is to enable the factory information system to more thoroughly collect data from every corner of the production line. This will improve its ability to measure Overall Equipment Effectiveness (OEE) and translate into increased production efficiencies. System Requirements • Instant status updates while still consuming minimal bandwidth to relieve strain on limited factory networks • Interoperable with ABB Real-TPI • Small form factor appropriate for deployment where space is scarce • Remote software management and configuration to simplify operations
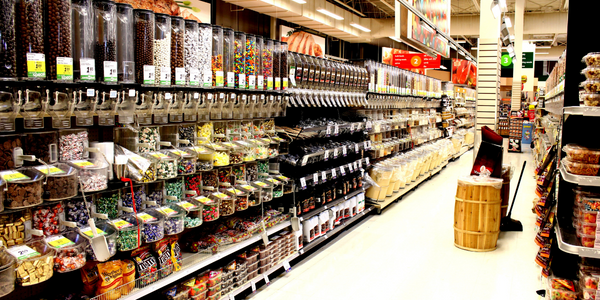
Case Study
How Sirqul’s IoT Platform is Crafting Carrefour’s New In-Store Experiences
Carrefour Taiwan’s goal is to be completely digital by end of 2018. Out-dated manual methods for analysis and assumptions limited Carrefour’s ability to change the customer experience and were void of real-time decision-making capabilities. Rather than relying solely on sales data, assumptions, and disparate systems, Carrefour Taiwan’s CEO led an initiative to find a connected IoT solution that could give the team the ability to make real-time changes and more informed decisions. Prior to implementing, Carrefour struggled to address their conversion rates and did not have the proper insights into the customer decision-making process nor how to make an immediate impact without losing customer confidence.
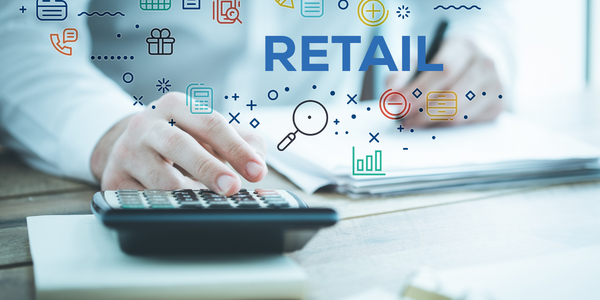
Case Study
Digital Retail Security Solutions
Sennco wanted to help its retail customers increase sales and profits by developing an innovative alarm system as opposed to conventional connected alarms that are permanently tethered to display products. These traditional security systems were cumbersome and intrusive to the customer shopping experience. Additionally, they provided no useful data or analytics.
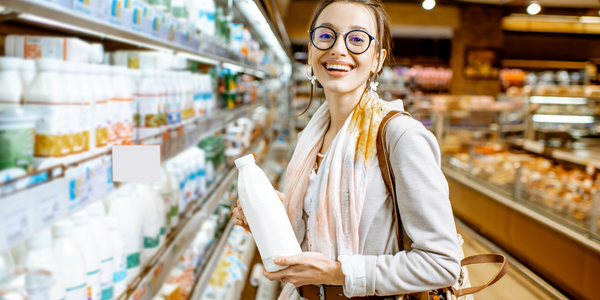
Case Study
Ensures Cold Milk in Your Supermarket
As of 2014, AK-Centralen has over 1,500 Danish supermarkets equipped, and utilizes 16 operators, and is open 24 hours a day, 365 days a year. AK-Centralen needed the ability to monitor the cooling alarms from around the country, 24 hours a day, 365 days a year. Each and every time the door to a milk cooler or a freezer does not close properly, an alarm goes off on a computer screen in a control building in southwestern Odense. This type of alarm will go off approximately 140,000 times per year, equating to roughly 400 alarms in a 24-hour period. Should an alarm go off, then there is only a limited amount of time to act before dairy products or frozen pizza must be disposed of, and this type of waste can quickly start to cost a supermarket a great deal of money.
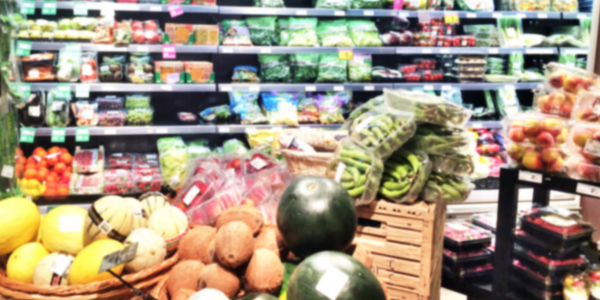
Case Study
Supermarket Energy Savings
The client had previously deployed a one-meter-per-store monitoring program. Given the manner in which energy consumption changes with external temperature, hour of the day, day of week and month of year, a single meter solution lacked the ability to detect the difference between a true problem and a changing store environment. Most importantly, a single meter solution could never identify root cause of energy consumption changes. This approach never reduced the number of truck-rolls or man-hours required to find and resolve issues.