Download PDF
US Foods Analyzes Transactions from 300,000 Customers with Snowflake and DataRobot
Technology Category
- Infrastructure as a Service (IaaS) - Cloud Computing
- Analytics & Modeling - Predictive Analytics
Applicable Industries
- Food & Beverage
Applicable Functions
- Sales & Marketing
- Business Operation
Use Cases
- Predictive Maintenance
- Supply Chain Visibility
Services
- Data Science Services
- Cloud Planning, Design & Implementation Services
The Challenge
US Foods, one of America's largest food companies, was facing significant challenges with its legacy, on-premises data warehouse. The system required constant maintenance, experienced frequent resource contention, and could not affordably store more than two years’ worth of data. Business analysts took weeks to prepare a single report due to the system’s counterintuitive user interface, inability to load large data sets, and limited BI features. Reporting delays led some business users to seek insights from siloed Microsoft Access databases and Excel spreadsheets. Data science modeling to predict customer loyalty and churn rate was simply impossible. US Foods evaluated several cloud data management solutions, but none offered the right mix of performance and affordability.
About The Customer
US Foods is a food service distributor and one of America's largest food companies. The company is based in Rosemont, Illinois and has approximately 26,000 employees. US Foods provides an expansive catalog of food products, culinary equipment, supplies, and technology to approximately 300,000 restaurants and food service operators. The company uses data analytics and data science to monitor performance, predict churn rate, and accelerate growth.
The Solution
US Foods adopted Snowflake’s cloud data platform, which scaled to become their single analytics repository for transaction data. The Snowflake Connector for Python and bulk loading from Amazon S3 enabled daily ingestion of large data sets without causing bottlenecks. Snowflake’s native support for SQL and clean, easy-to-navigate interface accelerated report creation. DataRobot integration enabled predictive analytics for churn rate that identified at-risk customers in need of proactive outreach by US Foods’ retention team. This solution provided a significant improvement in data management and predictive analytics capabilities for US Foods.
Operational Impact
Quantitative Benefit
Related Case Studies.
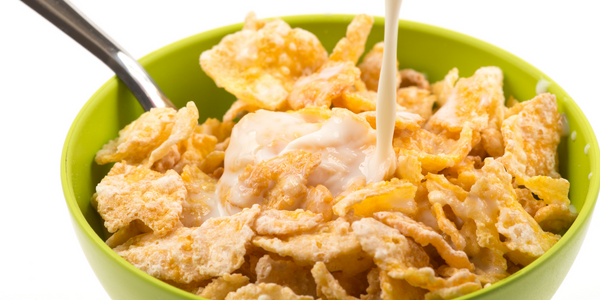
Case Study
The Kellogg Company
Kellogg keeps a close eye on its trade spend, analyzing large volumes of data and running complex simulations to predict which promotional activities will be the most effective. Kellogg needed to decrease the trade spend but its traditional relational database on premises could not keep up with the pace of demand.
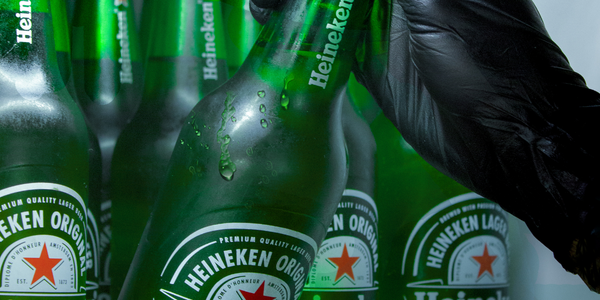
Case Study
HEINEKEN Uses the Cloud to Reach 10.5 Million Consumers
For 2012 campaign, the Bond promotion, it planned to launch the campaign at the same time everywhere on the planet. That created unprecedented challenges for HEINEKEN—nowhere more so than in its technology operation. The primary digital content for the campaign was a 100-megabyte movie that had to play flawlessly for millions of viewers worldwide. After all, Bond never fails. No one was going to tolerate a technology failure that might bruise his brand.Previously, HEINEKEN had supported digital media at its outsourced datacenter. But that datacenter lacked the computing resources HEINEKEN needed, and building them—especially to support peak traffic that would total millions of simultaneous hits—would have been both time-consuming and expensive. Nor would it have provided the geographic reach that HEINEKEN needed to minimize latency worldwide.
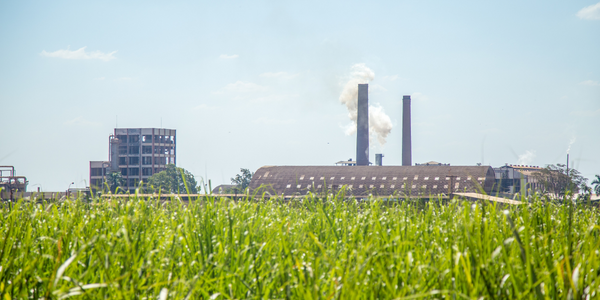
Case Study
Energy Management System at Sugar Industry
The company wanted to use the information from the system to claim under the renewable energy certificate scheme. The benefit to the company under the renewable energy certificates is Rs 75 million a year. To enable the above, an end-to-end solution for load monitoring, consumption monitoring, online data monitoring, automatic meter data acquisition which can be exported to SAP and other applications is required.
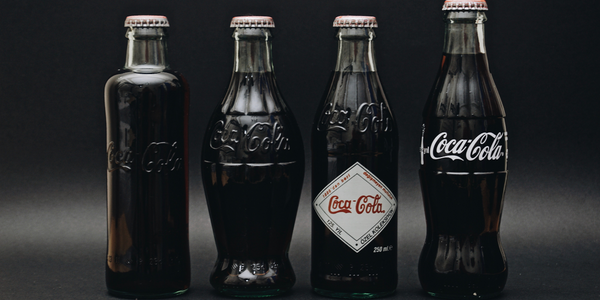
Case Study
Coca Cola Swaziland Conco Case Study
Coco Cola Swaziland, South Africa would like to find a solution that would enable the following results: - Reduce energy consumption by 20% in one year. - Formulate a series of strategic initiatives that would enlist the commitment of corporate management and create employee awareness while helping meet departmental targets and investing in tools that assist with energy management. - Formulate a series of tactical initiatives that would optimize energy usage on the shop floor. These would include charging forklifts and running cold rooms only during off-peak periods, running the dust extractors only during working hours and basing lights and air-conditioning on someone’s presence. - Increase visibility into the factory and other processes. - Enable limited, non-intrusive control functions for certain processes.
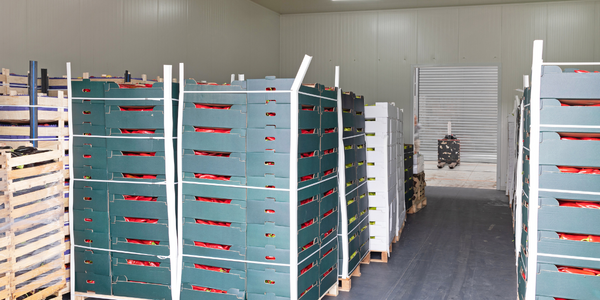
Case Study
Temperature Monitoring for Restaurant Food Storage
When it came to implementing a solution, Mr. Nesbitt had an idea of what functionality that he wanted. Although not mandated by Health Canada, Mr. Nesbitt wanted to ensure quality control issues met the highest possible standards as part of his commitment to top-of-class food services. This wish list included an easy-to use temperature-monitoring system that could provide a visible display of the temperatures of all of his refrigerators and freezers, including historical information so that he could review the performance of his equipment. It also had to provide alert notification (but email alerts and SMS text message alerts) to alert key staff in the event that a cooling system was exceeding pre-set warning limits.
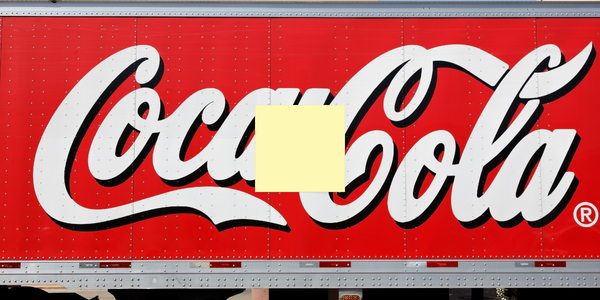
Case Study
Coca-Cola Refreshments, U.S.
Coca-Cola Refreshments owns and manages Coca-Cola branded refrigerators in retail establishments. Legacy systems were used to locate equipment information by logging onto multiple servers which took up to 8 hours to update information on 30-40 units. The company had no overall visibility into equipment status or maintenance history.