下载PDF
DeepL’s Transformation Journey with ClickHouse: A Case Study
技术
- 应用基础设施与中间件 - 中间件、SDK 和库
- 平台即服务 (PaaS) - 应用开发平台
适用行业
- 建筑物
- 电子商务
适用功能
- 维护
- 产品研发
用例
- 楼宇自动化与控制
- 实验自动化
服务
- 系统集成
- 培训
挑战
DeepL 是一家语言翻译服务公司,希望在 2020 年以保护隐私的方式增强其分析能力。该公司希望自行托管一个可以处理大量数据并提供快速查询时间的解决方案。他们评估了几个选项,包括 Hadoop 世界,但发现它的维护成本太高,而且设置起来也很耗时。 DeepL 还希望在前端开发人员创建新事件时自动执行更改表模式的过程,否则这会让团队不堪重负。该公司需要一个能够处理复杂事件和查询以了解用户交互的系统,这是 Google Analytics 等传统工具无法提供的。此外,DeepL 希望保持对数据的完全控制,同时牢记用户隐私。
关于客户
DeepL 是一种语言翻译服务,利用人工智能提供比其他服务更准确、更自然的翻译。该公司成立于2017年,总部位于德国科隆。 DeepL 支持多种语言之间的翻译,包括英语、德语、法语、西班牙语、意大利语、荷兰语和波兰语。公司致力于保护用户隐私,不与第三方共享个人数据。 DeepL 的使命是打破语言障碍,拉近文化的距离。该公司的服务被全球数百万人使用,包括个人、企业和组织。
解决方案
DeepL 选择 ClickHouse 作为他们的中央数据仓库,因为它来自 apt-repository 的单一二进制部署,这使得设置最小可行产品 (MVP) 变得简单快捷。 MVP 包含一个 API(用户的浏览器将事件发送到其中)、作为消息代理的 Kafka、从 Kafka 写入 ClickHouse 的接收器、ClickHouse 本身以及用于可视化结果的 Metabase。该公司在自动化方面投入巨资,并决定为所有事件和表架构提供一个组合的事实来源。当前端开发人员想要创建一个新事件时,他们需要在 protobuf 中定义该事件。该 protobuf 架构文件用于三个目的:验证事件、计算 ClickHouse 表架构以及创建有关所有事件的文档。随着时间的推移,DeepL 从单个节点设置扩展到由 3 个分片和 3 个副本组成的集群,每天摄取约 5 亿个原始行。 ClickHouse 在 DeepL 的实验框架和个性化 ML 基础设施中也发挥了至关重要的作用。
运营影响
数量效益
相关案例.
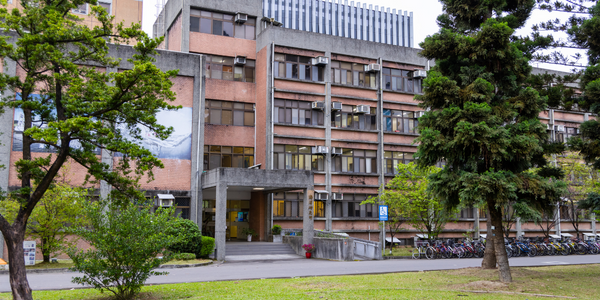
Case Study
Energy Saving & Power Monitoring System
Recently a university in Taiwan was experiencing dramatic power usage increases due to its growing number of campus buildings and students. Aiming to analyze their power consumption and increase their power efficiency across 52 buildings, the university wanted to build a power management system utilizing web-based hardware and software. With these goals in mind, they contacted Advantech to help them develop their system and provide them with the means to save energy in the years to come.
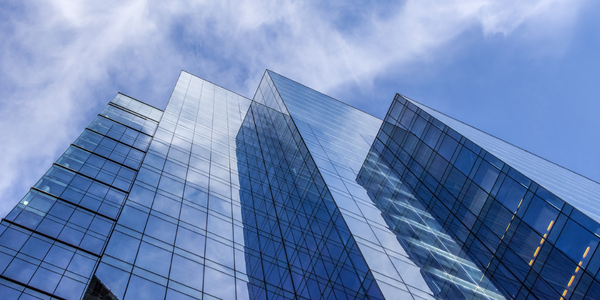
Case Study
Intelligent Building Automation System and Energy Saving Solution
One of the most difficult problems facing the world is conserving energy in buildings. However, it is not easy to have a cost-effective solution to reduce energy usage in a building. One solution for saving energy is to implement an intelligent building automation system (BAS) which can be controlled according to its schedule. In Indonesia a large university with a five floor building and 22 classrooms wanted to save the amount of energy being used.
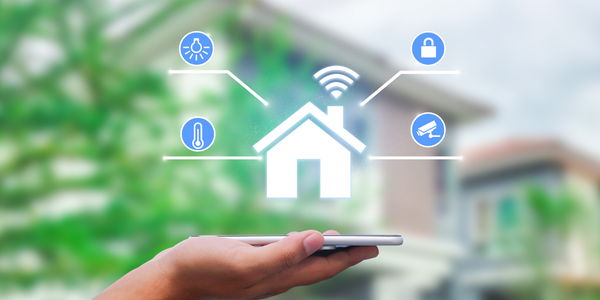
Case Study
Powering Smart Home Automation solutions with IoT for Energy conservation
Many industry leaders that offer Smart Energy Management products & solutions face challenges including:How to build a scalable platform that can automatically scale-up to on-board ‘n’ number of Smart home devicesData security, solution availability, and reliability are the other critical factors to deal withHow to create a robust common IoT platform that handles any kind of smart devicesHow to enable data management capabilities that would help in intelligent decision-making

Case Study
Commercial Building Automation Boosts Energy Efficiency
One of the challenges to building automation is the multitude of non-interoperable communications protocols that have evolved over the years. Buildings have several islands of automation. Bridging the islands of different automation without losing the considerable investment in each specialized control network is the main focus in this solution.
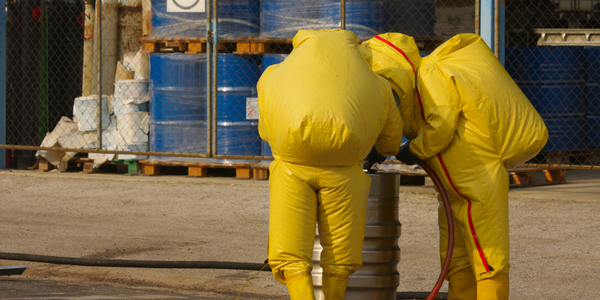
Case Study
Protecting a Stadium from Hazardous Materials Using IoT2cell's Mobility Platform
There was a need for higher security at the AT&T Stadium during the NFL draft. There was a need to ensure that nuclear radiation material was not smuggled inside the stadium. Hazmat materials could often be missed in a standard checkpoint when gaining entry into a stadium.