下载PDF
ShopBack's Journey to Efficient Embedded Analytics with Cube
技术
- 应用基础设施与中间件 - 事件驱动型应用
- 基础设施即服务 (IaaS) - 云数据库
适用行业
- 电子商务
- 零售
适用功能
- 采购
- 销售与市场营销
用例
- 零售店自动化
- 时间敏感网络
挑战
对于 ShopBack 来说,面临的挑战是分析其平台上的所有交易,包括购买价值和销量,并为内部和外部用户提供汇总数据的仪表板报告。他们需要一个能够处理关系数据并将其预先聚合到 OLAP 多维数据集中进行分析的解决方案。
关于客户
ShopBack 是亚太地区最大的购物奖励和发现平台,业务遍及多个国家。他们拥有超过 2800 万用户,并为其商业合作伙伴带来了数十亿美元的销售额。他们需要一个解决方案来分析交易数据并为用户和合作伙伴提供见解。
解决方案
ShopBack 决定使用 Cube 作为嵌入式分析解决方案。 Cube 允许他们在预先聚合的 OLAP 多维数据集中存储和分析关系数据,从而提高查询性能。他们在架构中实现了 Cube,使用 AWS 中的 Node.js 应用程序和集成的 Cube API 实例来处理对其前端应用程序的请求。他们还使用数据管道进行 ETL 流程并使用 Redis 进行缓存。
运营影响
数量效益
相关案例.
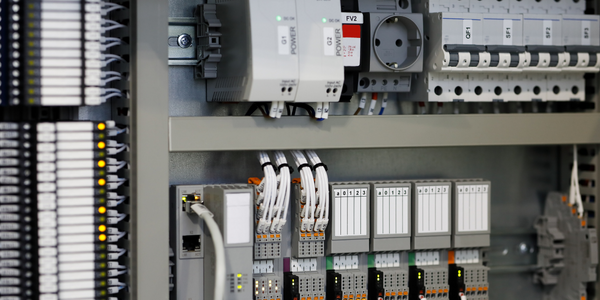
Case Study
Improving Production Line Efficiency with Ethernet Micro RTU Controller
Moxa was asked to provide a connectivity solution for one of the world's leading cosmetics companies. This multinational corporation, with retail presence in 130 countries, 23 global braches, and over 66,000 employees, sought to improve the efficiency of their production process by migrating from manual monitoring to an automatic productivity monitoring system. The production line was being monitored by ABB Real-TPI, a factory information system that offers data collection and analysis to improve plant efficiency. Due to software limitations, the customer needed an OPC server and a corresponding I/O solution to collect data from additional sensor devices for the Real-TPI system. The goal is to enable the factory information system to more thoroughly collect data from every corner of the production line. This will improve its ability to measure Overall Equipment Effectiveness (OEE) and translate into increased production efficiencies. System Requirements • Instant status updates while still consuming minimal bandwidth to relieve strain on limited factory networks • Interoperable with ABB Real-TPI • Small form factor appropriate for deployment where space is scarce • Remote software management and configuration to simplify operations
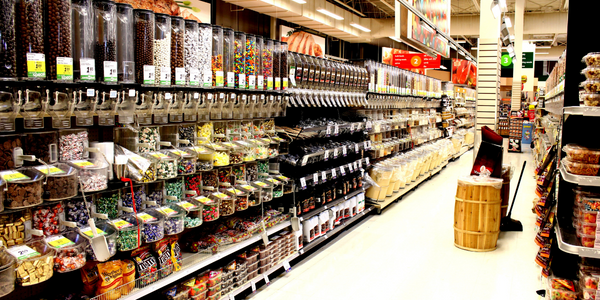
Case Study
How Sirqul’s IoT Platform is Crafting Carrefour’s New In-Store Experiences
Carrefour Taiwan’s goal is to be completely digital by end of 2018. Out-dated manual methods for analysis and assumptions limited Carrefour’s ability to change the customer experience and were void of real-time decision-making capabilities. Rather than relying solely on sales data, assumptions, and disparate systems, Carrefour Taiwan’s CEO led an initiative to find a connected IoT solution that could give the team the ability to make real-time changes and more informed decisions. Prior to implementing, Carrefour struggled to address their conversion rates and did not have the proper insights into the customer decision-making process nor how to make an immediate impact without losing customer confidence.
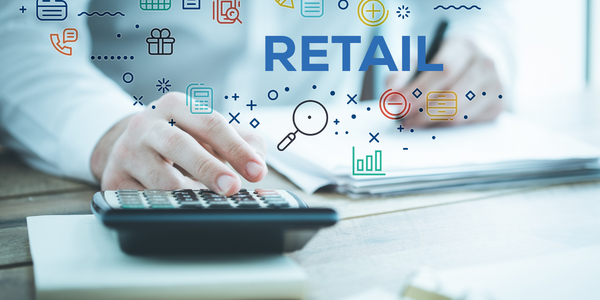
Case Study
Digital Retail Security Solutions
Sennco wanted to help its retail customers increase sales and profits by developing an innovative alarm system as opposed to conventional connected alarms that are permanently tethered to display products. These traditional security systems were cumbersome and intrusive to the customer shopping experience. Additionally, they provided no useful data or analytics.
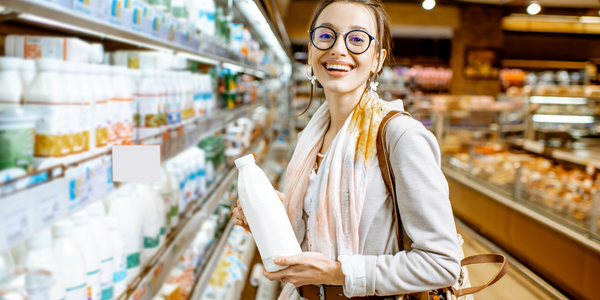
Case Study
Ensures Cold Milk in Your Supermarket
As of 2014, AK-Centralen has over 1,500 Danish supermarkets equipped, and utilizes 16 operators, and is open 24 hours a day, 365 days a year. AK-Centralen needed the ability to monitor the cooling alarms from around the country, 24 hours a day, 365 days a year. Each and every time the door to a milk cooler or a freezer does not close properly, an alarm goes off on a computer screen in a control building in southwestern Odense. This type of alarm will go off approximately 140,000 times per year, equating to roughly 400 alarms in a 24-hour period. Should an alarm go off, then there is only a limited amount of time to act before dairy products or frozen pizza must be disposed of, and this type of waste can quickly start to cost a supermarket a great deal of money.
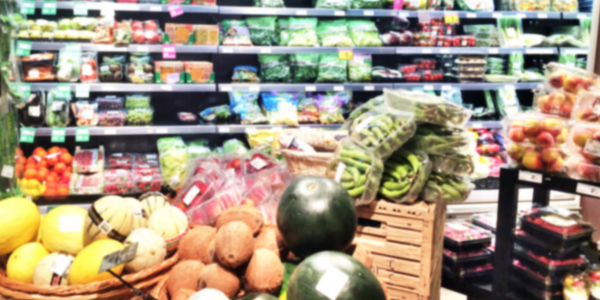
Case Study
Supermarket Energy Savings
The client had previously deployed a one-meter-per-store monitoring program. Given the manner in which energy consumption changes with external temperature, hour of the day, day of week and month of year, a single meter solution lacked the ability to detect the difference between a true problem and a changing store environment. Most importantly, a single meter solution could never identify root cause of energy consumption changes. This approach never reduced the number of truck-rolls or man-hours required to find and resolve issues.