InMarket Enhances Data Platform with ML-Powered Solution for Improved Efficiency and ROI
- Analytics & Modeling - Machine Learning
- Platform as a Service (PaaS) - Application Development Platforms
- Cement
- Retail
- Product Research & Development
- Sales & Marketing
- Real-Time Location System (RTLS)
- Retail Store Automation
- Data Science Services
- Testing & Certification
InMarket, an omnichannel marketing platform, was grappling with an inefficient legacy data platform that was unable to handle the growing volume of real-time location data collected from multiple sources. The platform, built using 50 AWS nodes and 400 bare metal nodes managed by Apache Mesos, was processing over 5 billion events daily, leading to delays, bottlenecks, and inefficiencies. The platform's performance was subpar, with a job success rate of only 40%, and 60% of Apache Spark jobs were randomly aborted in the system. This inefficiency led to developmental delays, inaccurate timeline projections, and a slow handoff process from data scientists to data engineers and operations. These issues were detrimental to InMarket's ability to attract marquee brands and slowed down revenue growth.
InMarket is an omnichannel marketing platform that assists Fortune 500 brands in identifying new prospects and customers, driving store visits, and increasing sales using AI- and data-driven consumer intelligence. The company collects and processes billions of real-time location data events daily to provide actionable insights to its clients. However, the company's legacy data platform was inefficient and unable to handle the growing volume of data, leading to developmental delays and a slow handoff process that was detrimental to its business model and revenue growth.
Provectus designed and built an ML-powered data & analytics platform capable of scaling thousands of analytics operations. The platform was implemented using Apache Spark, managed by Amazon EMR, and used Amazon S3 for storing RTB Logs from partners. AWS Lambda was used to process Amazon S3 events and notify the Kinesis producers into SQS for initial Kinesis processing. Data landed in Amazon Kinesis streams, triggering Spark Streaming jobs to perform required data transformations, aggregation, and cleaning. The cleaned and aggregated data was then uploaded to the output S3 bucket and loaded into the Snowflake DWH for BI & Analytics. The solution was deployed across four main clusters: a data cluster powered by Amazon EMR and AWS Lambda, a Kubernetes cluster with stateful microservices powered by Apache Kafka, a batch data processing cluster with Apache Spark and Apache Mesos, and a Snowflake cluster used for custom research and analytics.
Related Case Studies.
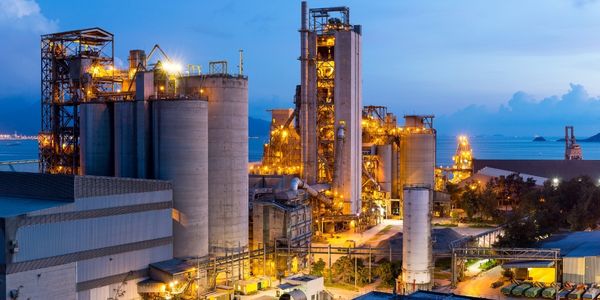
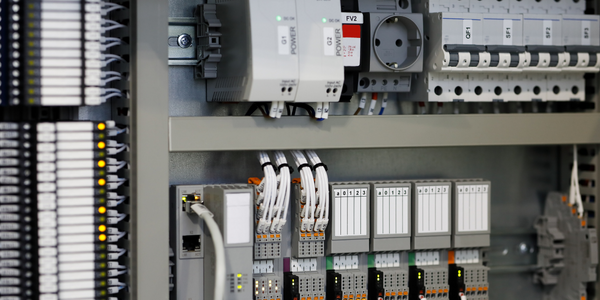
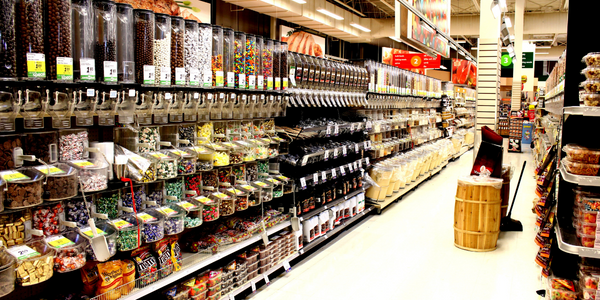
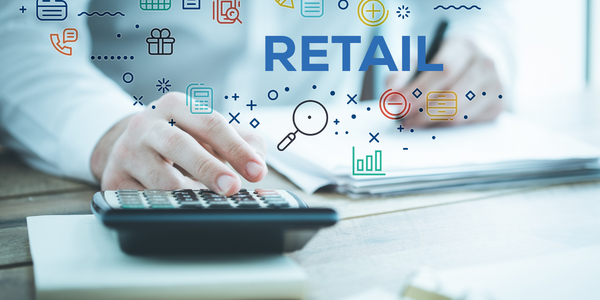
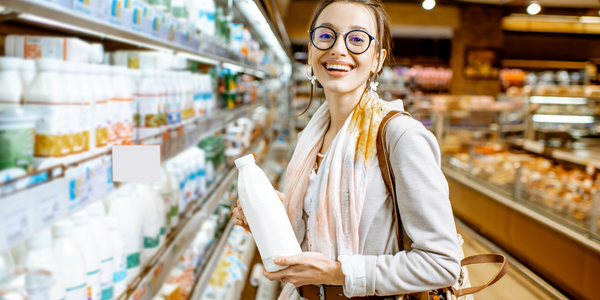