下载PDF
Aircraft Predictive Maintenance and Workflow Optimization
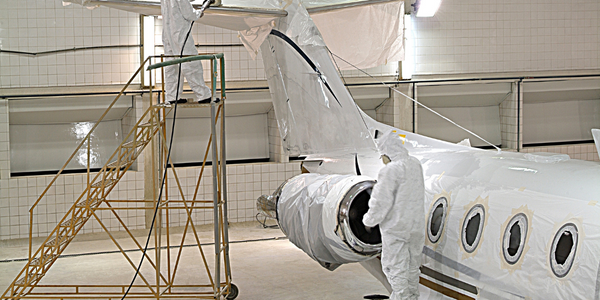
技术
- 分析与建模 - 机器学习
- 分析与建模 - 预测分析
适用行业
- 航天
适用功能
- 维护
用例
- 预测性维护
- 根因分析与诊断
挑战
首先,飞机制造商难以通过健康预测来监测飞机系统的健康状况并提供预测性维护见解。其次,飞机制造商需要一种能够提供上下文咨询并调整工作分配以匹配技术人员经验和专业知识的解决方案。
客户
未公开
关于客户
飞机制造商。
解决方案
SparkPredict 利用尖端机器学习技术构建机器规模的模式识别模型,以监控飞机内的机械系统并预测故障。这些算法的认知特性意味着 SparkPredict 可以部署到任何位置的飞机系统,并且洞察力将适应该特定飞机的独特特征。此外,SparkPredict 可以与诊断数据库、维护记录和人员记录等其他系统集成,以帮助对故障代码进行分类,推荐合适的人员,并以最佳方式安排维护。这将减少飞机必须在地面上花费的时间。
收集的数据
Asset Performance, Asset Status Tracking, Device Diagnostic Status, Fault Detection, Maintenance Records
运营影响
相关案例.
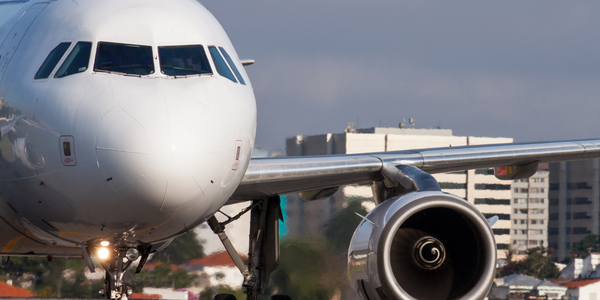
Case Study
Airbus Soars with Wearable Technology
Building an Airbus aircraft involves complex manufacturing processes consisting of thousands of moving parts. Speed and accuracy are critical to business and competitive advantage. Improvements in both would have high impact on Airbus’ bottom line. Airbus wanted to help operators reduce the complexity of assembling cabin seats and decrease the time required to complete this task.
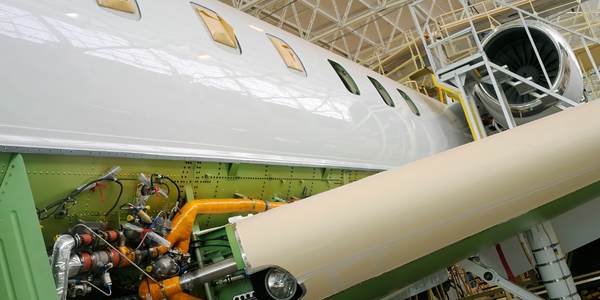
Case Study
Aerospace & Defense Case Study Airbus
For the development of its new wide-body aircraft, Airbus needed to ensure quality and consistency across all internal and external stakeholders. Airbus had many challenges including a very aggressive development schedule and the need to ramp up production quickly to satisfy their delivery commitments. The lack of communication extended design time and introduced errors that drove up costs.
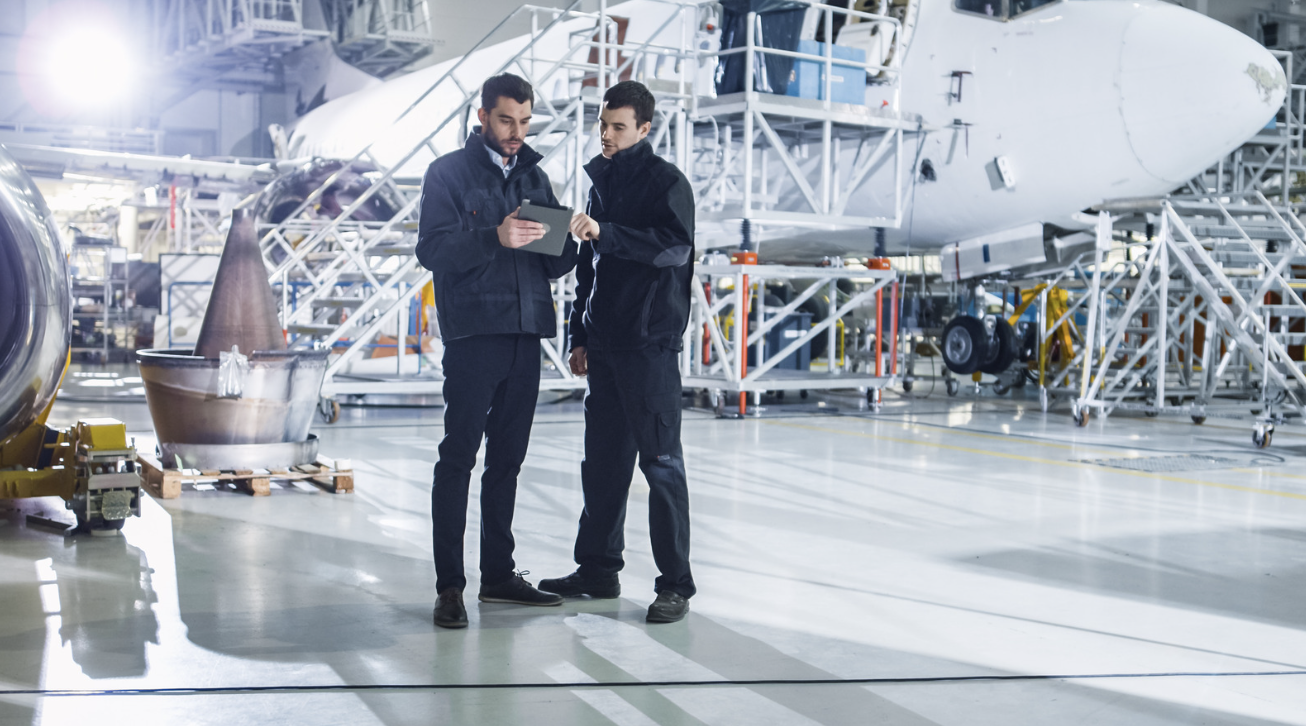
Case Study
Developing Smart Tools for the Airbus Factory
Manufacturing and assembly of aircraft, which involves tens of thousands of steps that must be followed by the operators, and a single mistake in the process could cost hundreds of thousands of dollars to fix, makes the room for error very small.
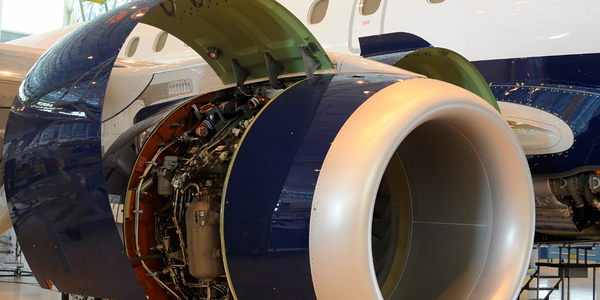
Case Study
Accelerate Production for Spirit AeroSystems
The manufacture and assembly of massive fuselage assemblies and other large structures generates a river of data. In fact, the bill of materials for a single fuselage alone can be millions of rows of data. In-house production processes and testing, as well as other manufacturers and customers created data flows that overwhelmed previous processes and information systems. Spirit’s customer base had grown substantially since their 2005 divestiture from Boeing, resulting in a $41 billion backlog of orders to fill. To address this backlog, meet increased customer demands and minimize additional capital investment, the company needed a way to improve throughput in the existing operational footprint. Spirit had a requirement from customers to increase fuselage production by 30%. To accomplish this goal, Spirit needed real-time information on its value chain and workflow. However, the two terabytes of data being pulled from their SAP ECC was unmanageable and overloaded their business warehouse. It had become time-consuming and difficult to pull aggregate data, disaggregate it for the needed information and then reassemble to create a report. During the 6-8 hours it took to build a report, another work shift (they run three per day) would have already taken place, thus the report content was out-of-date before it was ever delivered. As a result, supervisors often had to rely on manual efforts to provide charts, reports and analysis.
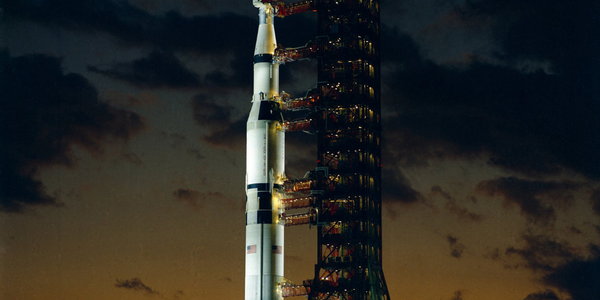
Case Study
SAP Leonardo Enabling Rocket Science
At times, ULA has as many as 15 different operating systems dedicated to overlapping processes, such as rocket design, testing, and launch. Multiple systems created unnecessary costs and unwanted confusion among workers at offices, factories, and launch sites in different location. In order to improve collaboration and transparency during vital activities that directly influence mission success, ULA wanted to improve data sharing and streamline manufacturing processes.